Bayesian dual systems population estimation for small domains
STATISTICS SURVEYS(2024)
摘要
Dual systems estimation is a capture-recapture approach to population estimation restricted to two captures of the population. When applied to human populations, the population captures may be existing incomplete listings of the population, as provided by census and administrative datasets. In contrast to much capture-recapture analysis, dual systems applications in official statistics are usually concerned with estimating the distribution of a human population, over combinations of covariates, such as age, sex, ethnic group and small geographic area, rather than focussing primarily on the total population count. We synthesise theory and methods for Bayesian dual systems estimation for this problem, which we refer to as small domain population estimation. We primarily work within a model framework that combines a general model for the covariate distribution, such as an unrestricted multinomial in the case of categorical covariates, with hierarchical logistic models for the probability of inclusion on the lists. We explore the issue of dependence between the two lists which leads to a well-known identifiability problem. We illustrate the use of informative priors for the degree of dependence expressed as an odds ratio, or for certain aggregate level population totals. Although progress can be made using informative priors, the underlying identifiability problem means that inferences are sensitive to prior choices. We relate our approach to the popular log-linear modelling approach to capture-recapture analysis and note that the latter is primarily a re-parameterisation of our model set-up, though with a specific implied prior for the total population in the case of Poisson log-linear models.
更多查看译文
关键词
Population estimation,Bayesian inference,small domain estimation
AI 理解论文
溯源树
样例
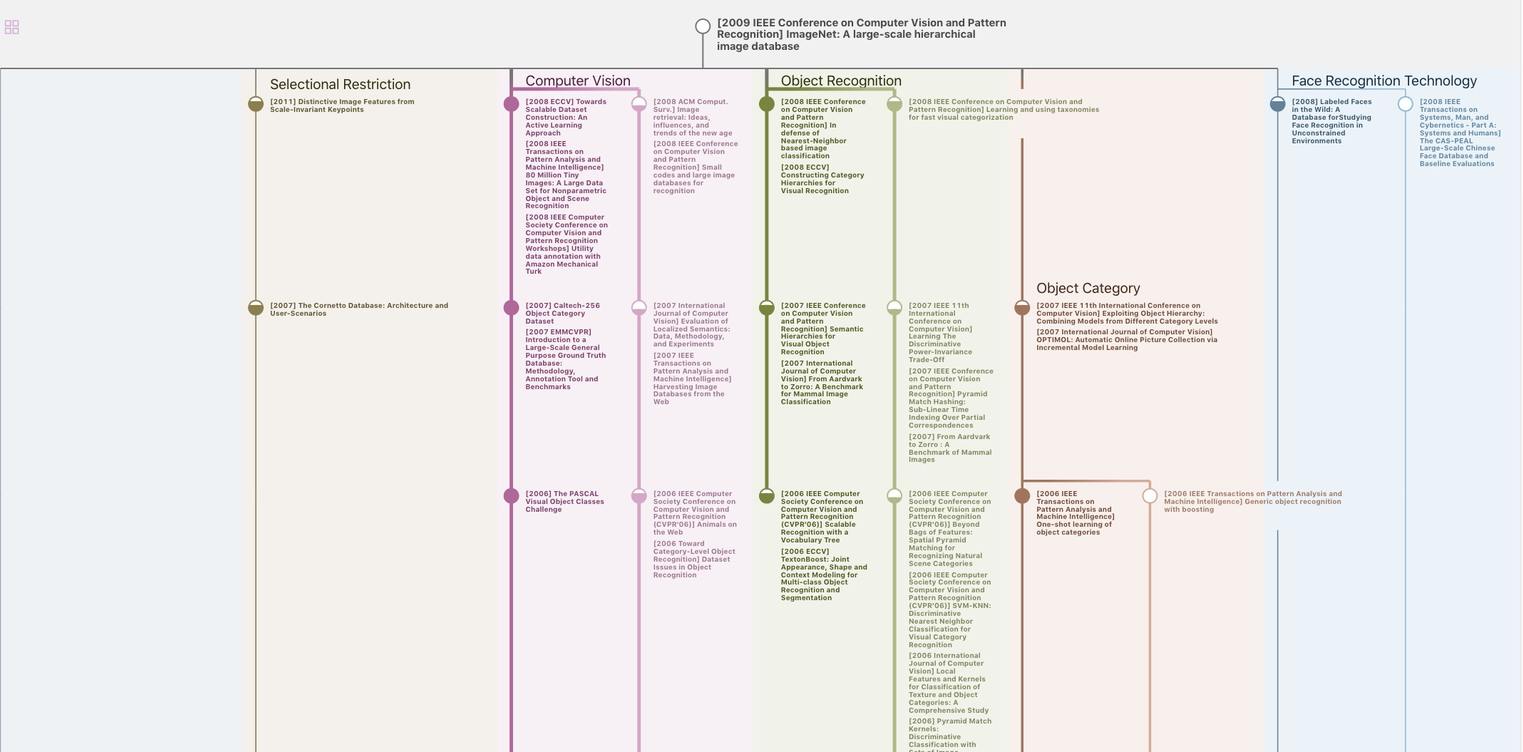
生成溯源树,研究论文发展脉络
Chat Paper
正在生成论文摘要