Hyperspectral image classification using a double-branch hierarchical partial convolution network
REMOTE SENSING LETTERS(2024)
摘要
Recently, convolutional neural network (CNN) and Transformer have been successfully applied in hyperspectral image (HSI) classification. Despite their success, some challenges persist. Popular HSI classification methods typically employ only a single patch as the network input, which may not provide robust information for various datasets or within different scenarios of a specific dataset. Large-scale patches are usually conducive to classifying large-scale scenes, while small-scale patches excel at classifying boundary locations. In addition, the majority of methods do not distinguish between the central pixel and its adjacent pixels, although the information from the central position is crucial. In this paper, a novel double-branch hierarchical partial convolution network (DBHP-Net) is proposed to address the aforementioned challenges. Concretely, DBHP-Net employs a new convolution, named TConv, to emphasize mining the spatial-channel features of the central position. Then, the TConvFormer Block is built by adopting the advanced engineering techniques of Transformer. Furthermore, we construct our model in a hierarchical style. The double-branch structure with large-scale and small-scale patches provides complementary information from the dataset. Experimental results based on randomly selected samples and spatially disjoint samples of two benchmark datasets demonstrate that our method outperforms other state-of-the-art (SOTA) methods.
更多查看译文
关键词
Hyperspectral image (HSI) classification,convolutional neural network (CNN),Transformer,partial convolution
AI 理解论文
溯源树
样例
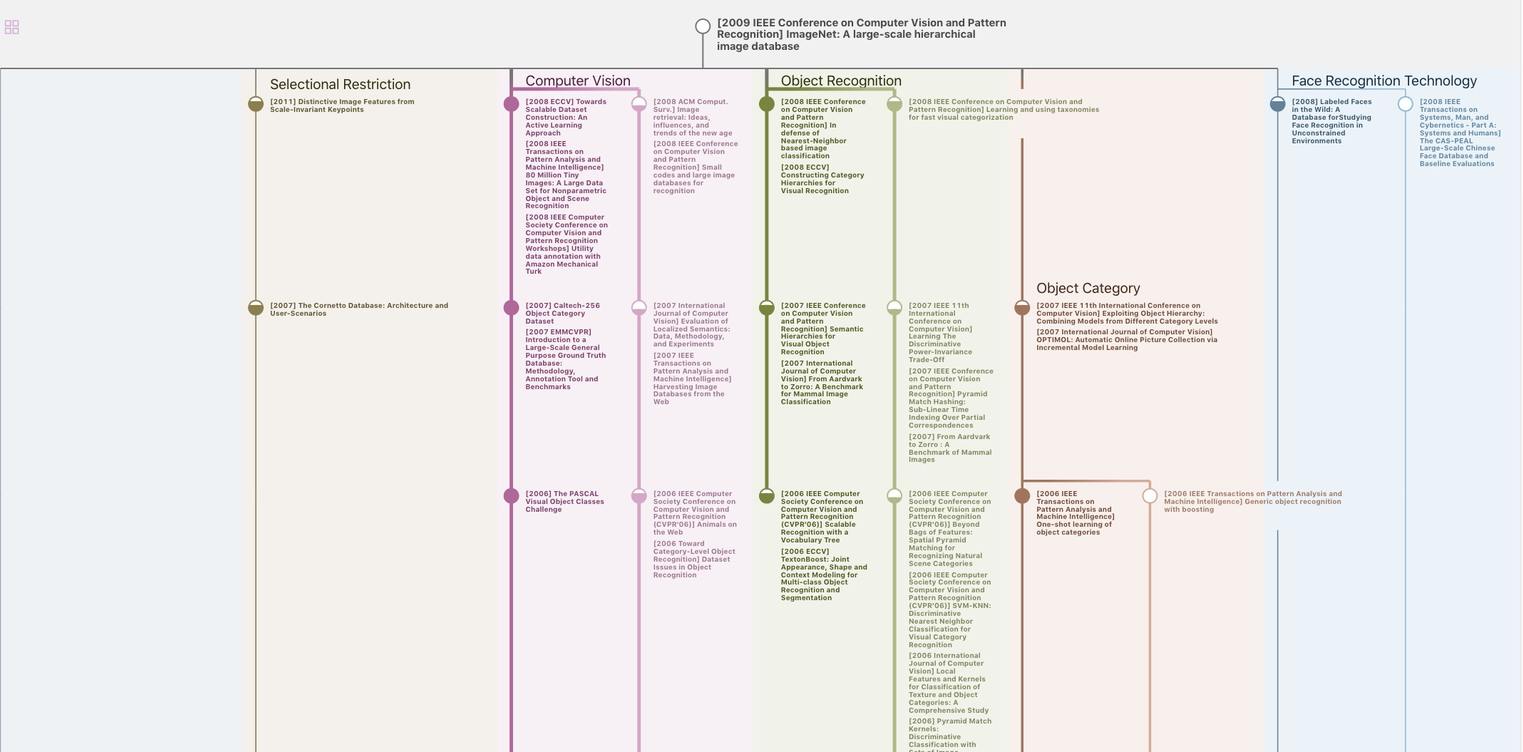
生成溯源树,研究论文发展脉络
Chat Paper
正在生成论文摘要