Martingale posterior distributions
JOURNAL OF THE ROYAL STATISTICAL SOCIETY SERIES B-STATISTICAL METHODOLOGY(2024)
摘要
The prior distribution is the usual starting point for Bayesian uncertainty. In this paper, we present a different perspective that focuses on missing observations as the source of statistical uncertainty, with the parameter of interest being known precisely given the entire population. We argue that the foundation of Bayesian inference is to assign a distribution on missing observations conditional on what has been observed. In the i.i.d. setting with an observed sample of size n, the Bayesian would thus assign a predictive distribution on the missing Yn+1:infinity conditional on Y1:n, which then induces a distribution on the parameter. We utilize Doob's theorem, which relies on martingales, to show that choosing the Bayesian predictive distribution returns the conventional posterior as the distribution of the parameter. Taking this as our cue, we relax the predictive machine, avoiding the need for the predictive to be derived solely from the usual prior to posterior to predictive density formula. We introduce the martingale posterior distribution, which returns Bayesian uncertainty on any statistic via the direct specification of the joint predictive. To that end, we introduce new predictive methodologies for multivariate density estimation, regression and classification that build upon recent work on bivariate copulas.
更多查看译文
关键词
Bayesian uncertainty,copula,martingale,predictive inference
AI 理解论文
溯源树
样例
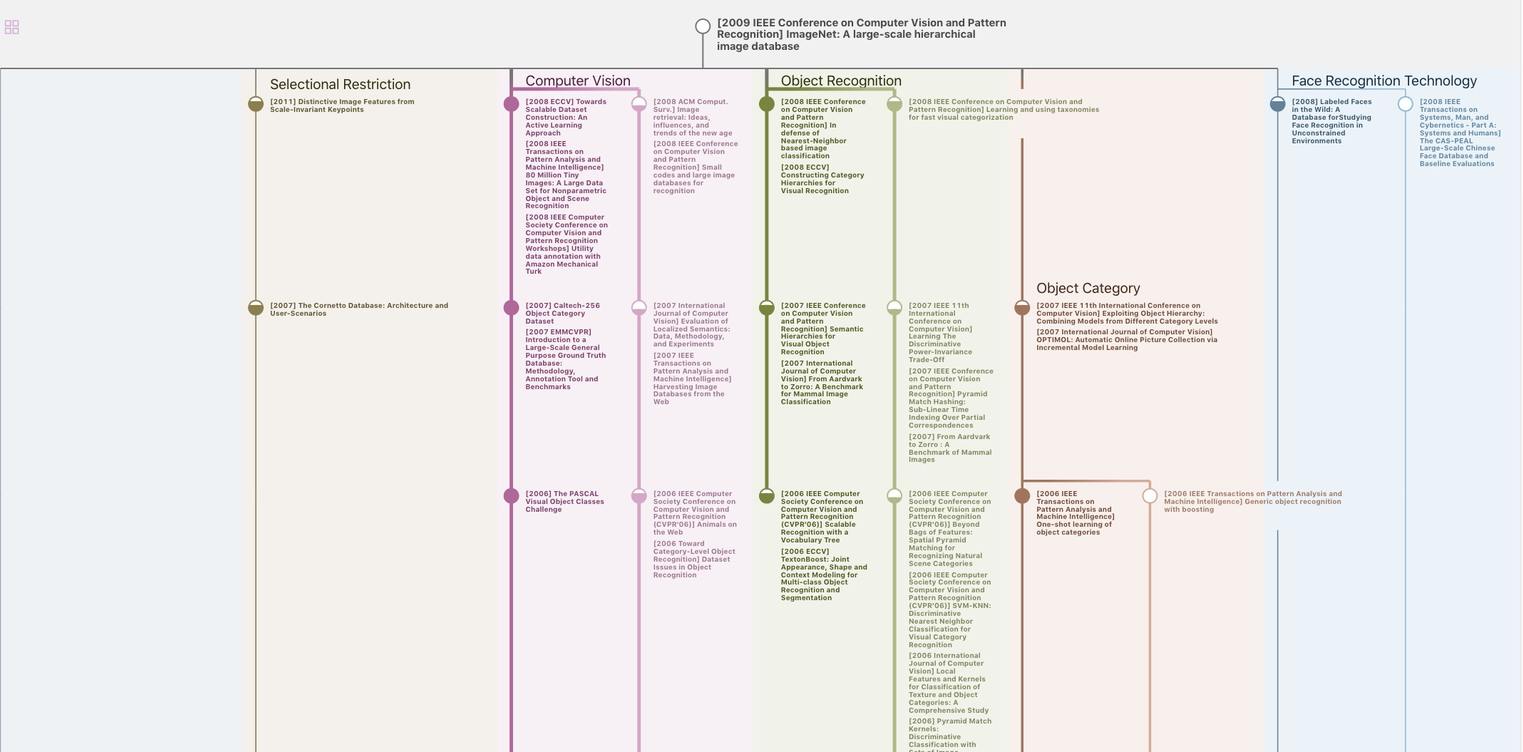
生成溯源树,研究论文发展脉络
Chat Paper
正在生成论文摘要