On the Security of "LSFL: A Lightweight and Secure Federated Learning Scheme for Edge Computing"
IEEE TRANSACTIONS ON INFORMATION FORENSICS AND SECURITY(2024)
摘要
Zhang et al. (2023) recently proposed a secure federated learning (FL) scheme named LSFL to guarantee Byzantine robustness while protecting privacy in FL. In this work, we show that LSFL breaches privacy it claimed. Specifically, we demonstrate that the secure Byzantine robustness procedure of LSFL exposes significant information of all participant models and data to a semi-honest server, thereby damaging privacy. Then, we analyze the reason for this security issue and give a suggestion to prevent privacy breaches in LSFL.
更多查看译文
关键词
Computational modeling,Robustness,Servers,Data models,Security,Federated learning,Data privacy,Byzantine robustness,privacy protection,secret sharing
AI 理解论文
溯源树
样例
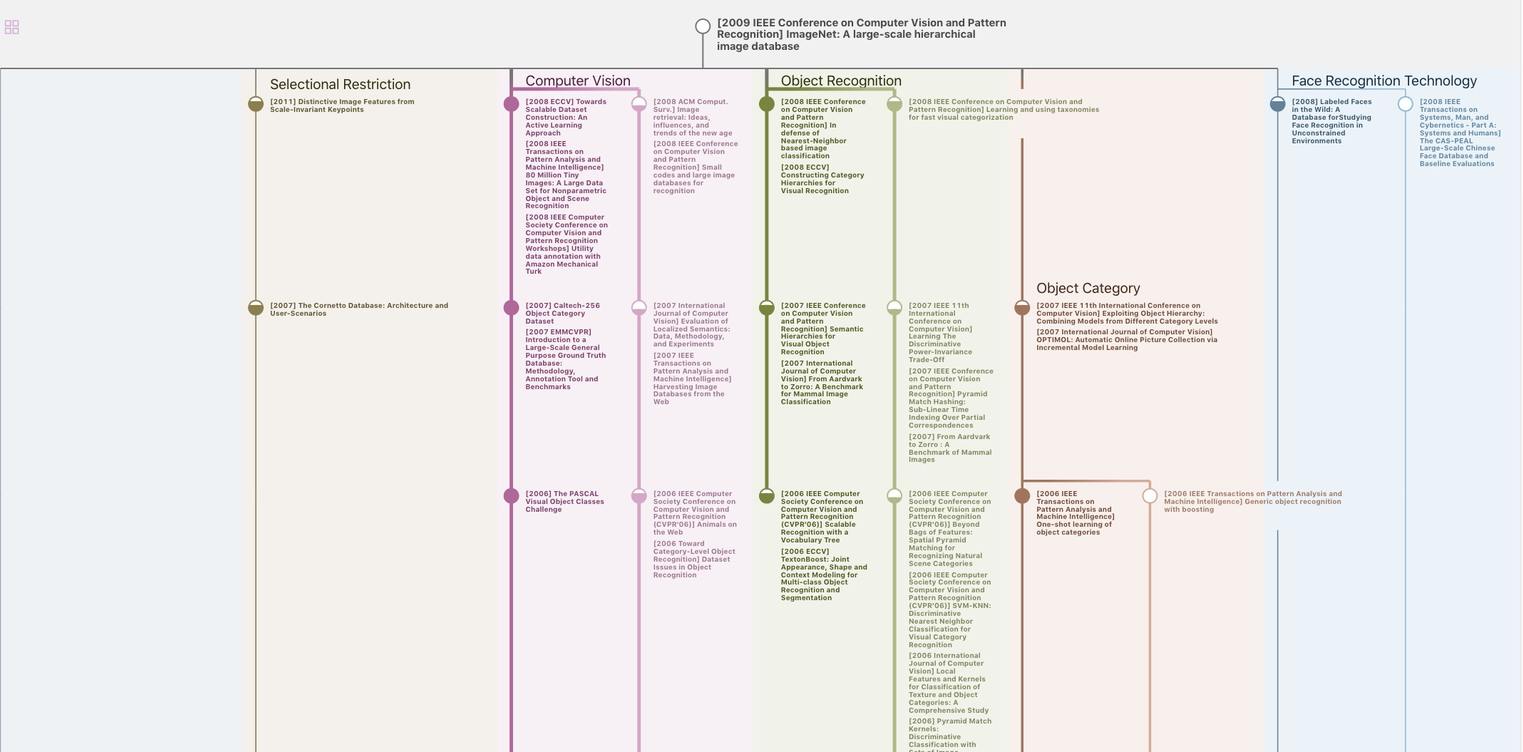
生成溯源树,研究论文发展脉络
Chat Paper
正在生成论文摘要