Robustness and Transferability of Adversarial Attacks on Different Image Classification Neural Networks
ELECTRONICS(2024)
摘要
Recent works demonstrated that imperceptible perturbations to input data, known as adversarial examples, can mislead neural networks' output. Moreover, the same adversarial sample can be transferable and used to fool different neural models. Such vulnerabilities impede the use of neural networks in mission-critical tasks. To the best of our knowledge, this is the first paper that evaluates the robustness of emerging CNN- and transformer-inspired image classifier models such as SpinalNet and Compact Convolutional Transformer (CCT) against popular white- and black-box adversarial attacks imported from the Adversarial Robustness Toolbox (ART). In addition, the adversarial transferability of the generated samples across given models was studied. The tests were carried out on the CIFAR-10 dataset, and the obtained results show that the level of susceptibility of SpinalNet against the same attacks is similar to that of the traditional VGG model, whereas CCT demonstrates better generalization and robustness. The results of this work can be used as a reference for further studies, such as the development of new attacks and defense mechanisms.
更多查看译文
关键词
adversarial attacks,robustness,transferability,CCT,VGG,SpinalNet,ART toolbox
AI 理解论文
溯源树
样例
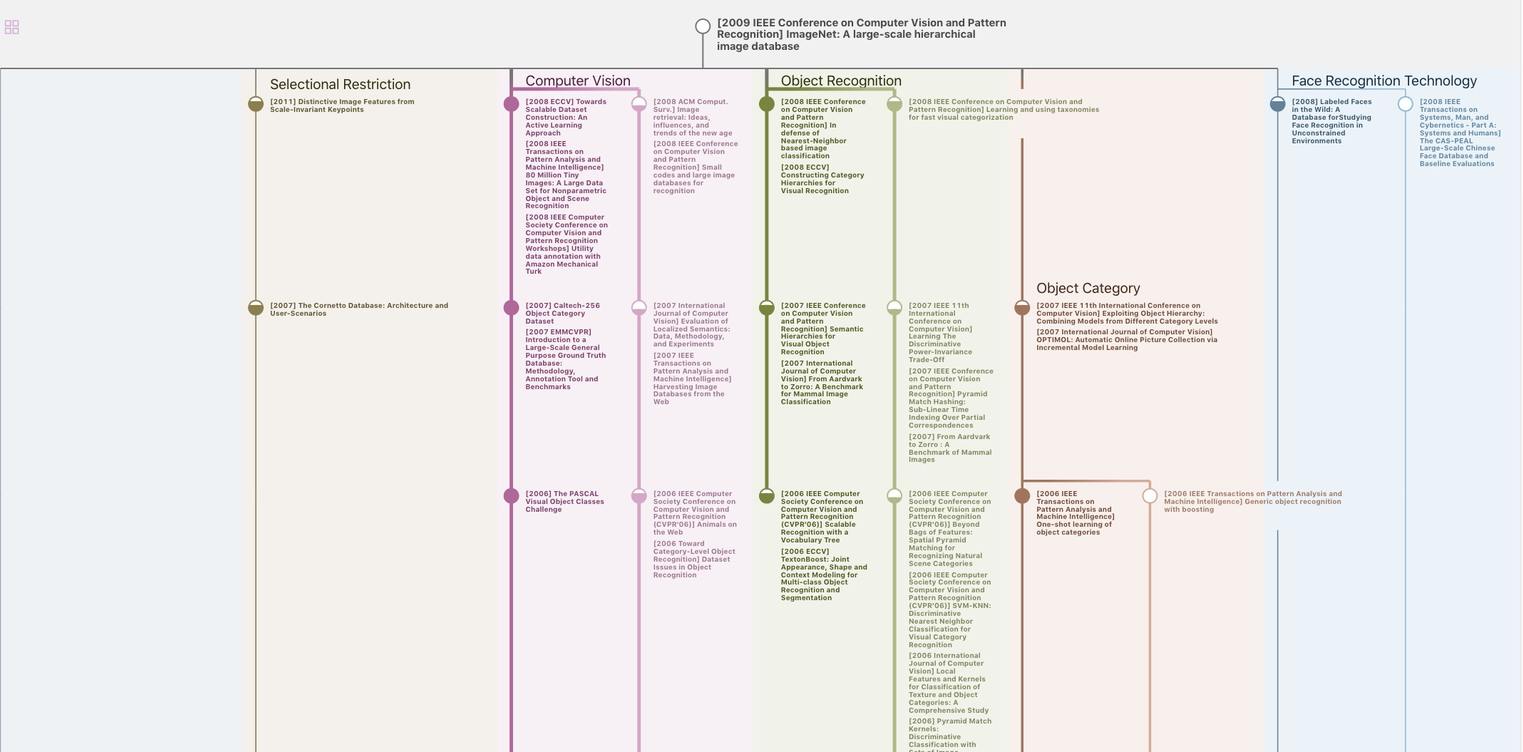
生成溯源树,研究论文发展脉络
Chat Paper
正在生成论文摘要