Attacking Robot Vision Models Efficiently Based on Improved Fast Gradient Sign Method
APPLIED SCIENCES-BASEL(2024)
摘要
The robot vision model is the basis for the robot to perceive and understand the environment and make correct decisions. However, the security and stability of robot vision models are seriously threatened by adversarial examples. In this study, we propose an adversarial attack algorithm, RMS-FGSM, for robot vision models based on root-mean-square propagation (RMSProp). RMS-FGSM uses an exponentially weighted moving average (EWMA) to reduce the weight of the historical cumulative squared gradient. Additionally, it can suppress the gradient growth based on an adaptive learning rate. By integrating with the RMSProp, RMS-FGSM is more likely to generate optimal adversarial examples, and a high attack success rate can be achieved. Experiments on two datasets (MNIST and CIFAR-100) and several models (LeNet, Alexnet, and Resnet-101) show that the attack success rate of RMS-FGSM is higher than the state-of-the-art methods. Above all, our generated adversarial examples have a smaller perturbation than those generated by existing methods under the same attack success rate.
更多查看译文
关键词
robot vision model,adversarial example,artificial intelligence security,fast gradient sign method
AI 理解论文
溯源树
样例
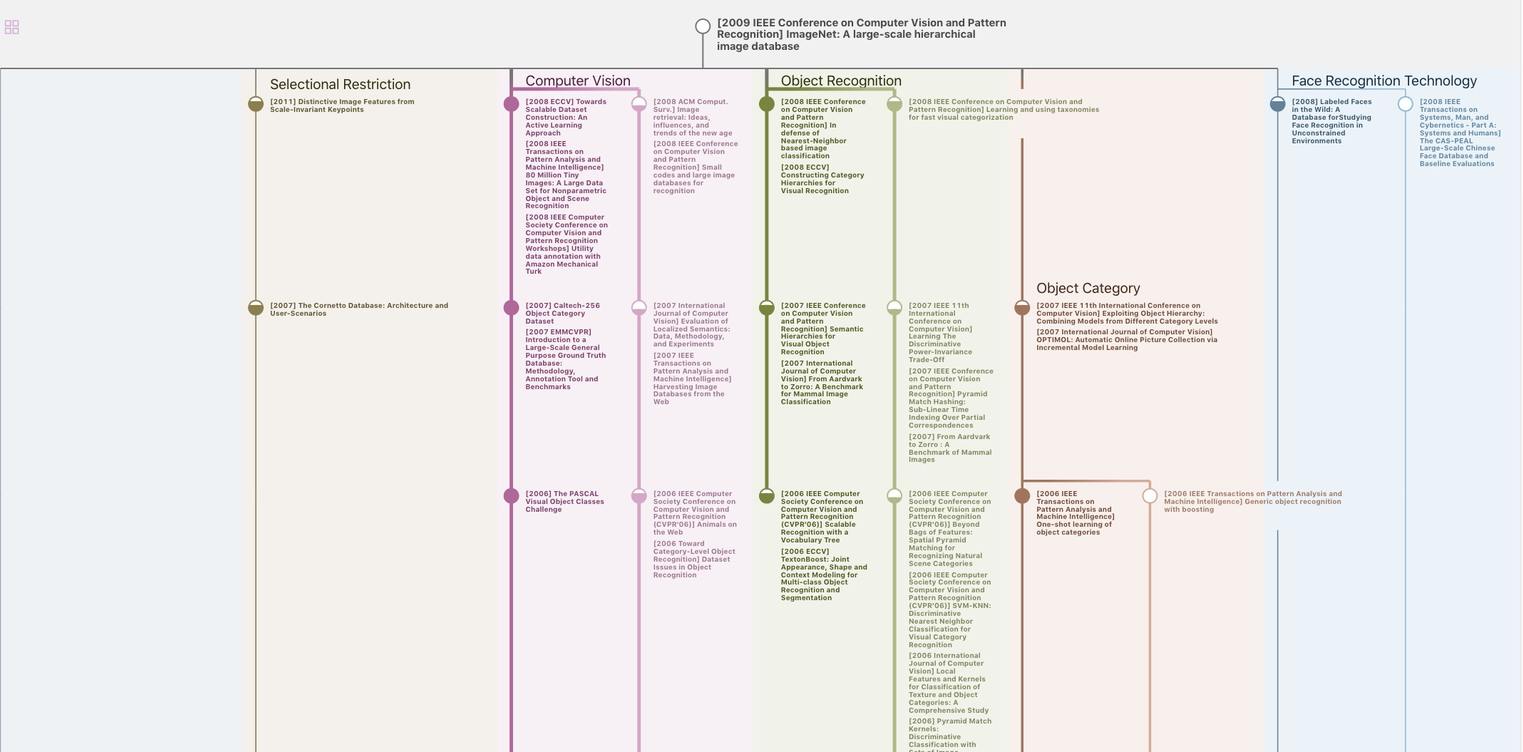
生成溯源树,研究论文发展脉络
Chat Paper
正在生成论文摘要