Machine learning study of D:A1:A2 ternary organic solar cells
ORGANIC ELECTRONICS(2024)
摘要
Introducing third component into donor:acceptor heterojunction provide new dimension to improve power conversion efficiency of organic solar cells (OSCs). However, the combining different donors and acceptors, as well as optimizing fabricating procedures are exhaust and tedious by experiment for ternary OSCs. Herein, we constructed the database, including 280 groups of D:A1:A2 type ternary OSCs with non-fullerene acceptors. The descriptors include the mass ratios, the root-mean-square roughness of morphology, the frontier molecular orbital energies and molecular fingerprints. Four kinds of machine learning (ML) models, including random forest (RF), extra tree regression, gradient boosted regression tree and adaptive boosting, were trained and tested. The coefficient of determination R2, Pearson's correlation coefficient r, root mean square error and mean absolute error were used to score the prediction results of ML models. The score and the slope of regression lines indicate RF model is prevail to other ML models in power conversion efficiency (PCE) prediction. Combining donors and acceptors in the constructed dataset generates 429,688 groups of D:A1:A2 type new ternary OSCs. Their photovoltaic performances were predicted with the trained RF model and screened. There are 138 groups of new ternary OSCs with the predicted PCE greater than 18.50%, and the champion predicted PCE (18.83%) corresponds to D18:AQx-18:ZH2 ternary OSC. The results of this work provide the guidance for optimizing ternary OSCs and can accelerate the development of high performance ternary OSCs.
更多查看译文
关键词
Ternary organic solar cells,Machine learning,Organic photovoltaics,Non-fullerene acceptors
AI 理解论文
溯源树
样例
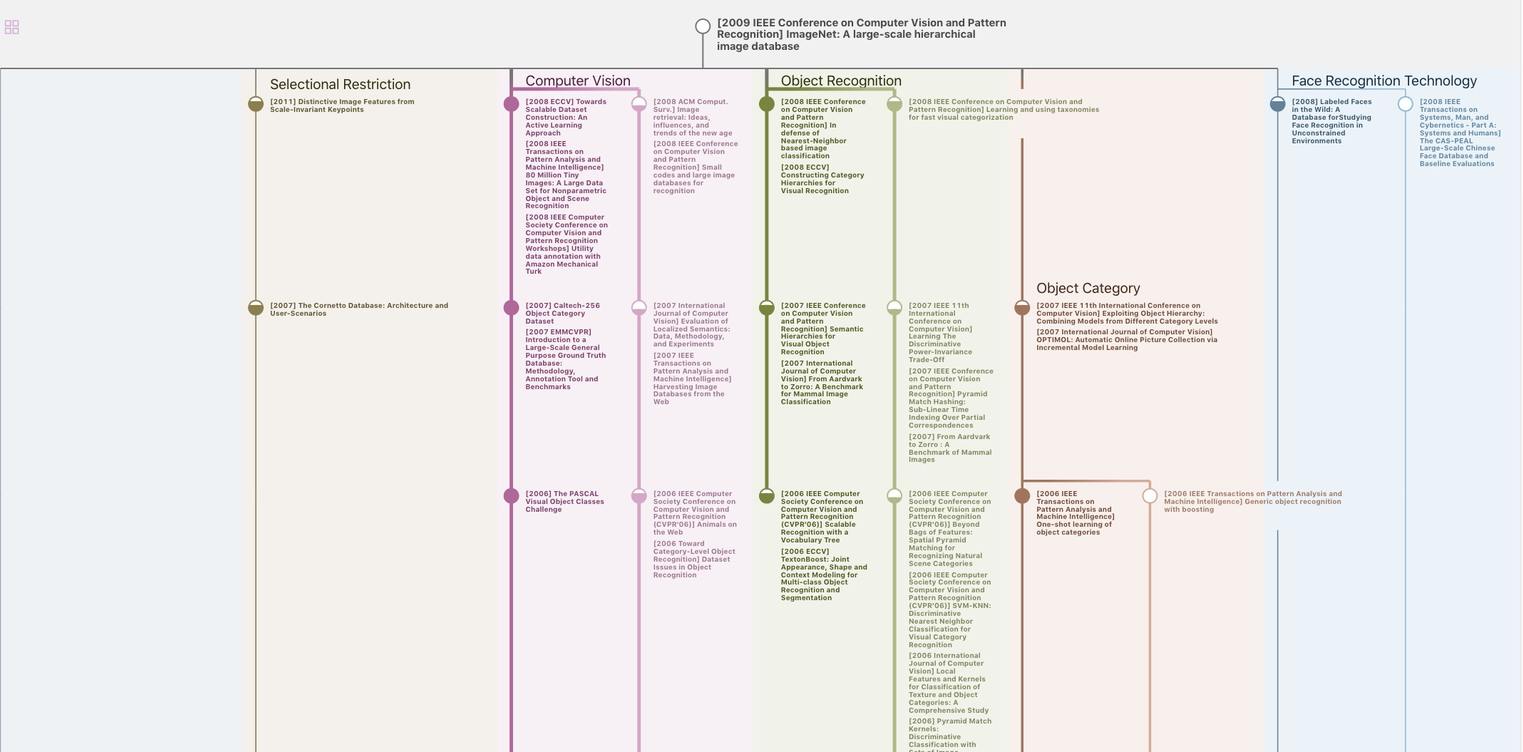
生成溯源树,研究论文发展脉络
Chat Paper
正在生成论文摘要