Investigation and optimization of multiple objectives by hybrid evolutionary algorithms for turning of Nimonic 80A under nano MQL environment
ADVANCES IN MATERIALS AND PROCESSING TECHNOLOGIES(2024)
摘要
Nimonic80A is one of the hard-to-cut superalloy materials. However, machining the same remains difficult. The present study's objective is attained by taking surface quality and tool wear as output core attributes of machining by deploying conventional statistical approaches and meta-heuristic optimisation algorithms to find optimal input attributes. Optimal input parameters were found using ANN hybridised with meta-heuristic optimisation algorithms, considering cutting acceleration as one of the response attributes along with cutting force (Fz), flank wear (Vb) and surface roughness (Ra). In this process, nanofluids were formulated by suspensions of graphene oxide into rice bran oil as the minimum quantity lubrication (MQL). Experiments were conducted based on L27 Taguchi experimental trials, and the experimental results revealed that minimum feed rate and cutting velocity reduced the cutting force, surface roughness, and tool flank wear up to 51% during nano-MQL turning conditions compared to dry machining. Thus, an artificial neural network (ANN) was used to generate the model for each machining response, which was further interfaced with multiobjective particle swarm optimisation (MOPSO) and multiobjective mayfly algorithms (MOMA) as a hybrid algorithm for finding optimal parameters. The optimal results were compared, and ANN-MOMA was found to be better.
更多查看译文
关键词
Nimonic 80A,nanofluids,superalloy machining,ANN modelling,multiobjective optimisation
AI 理解论文
溯源树
样例
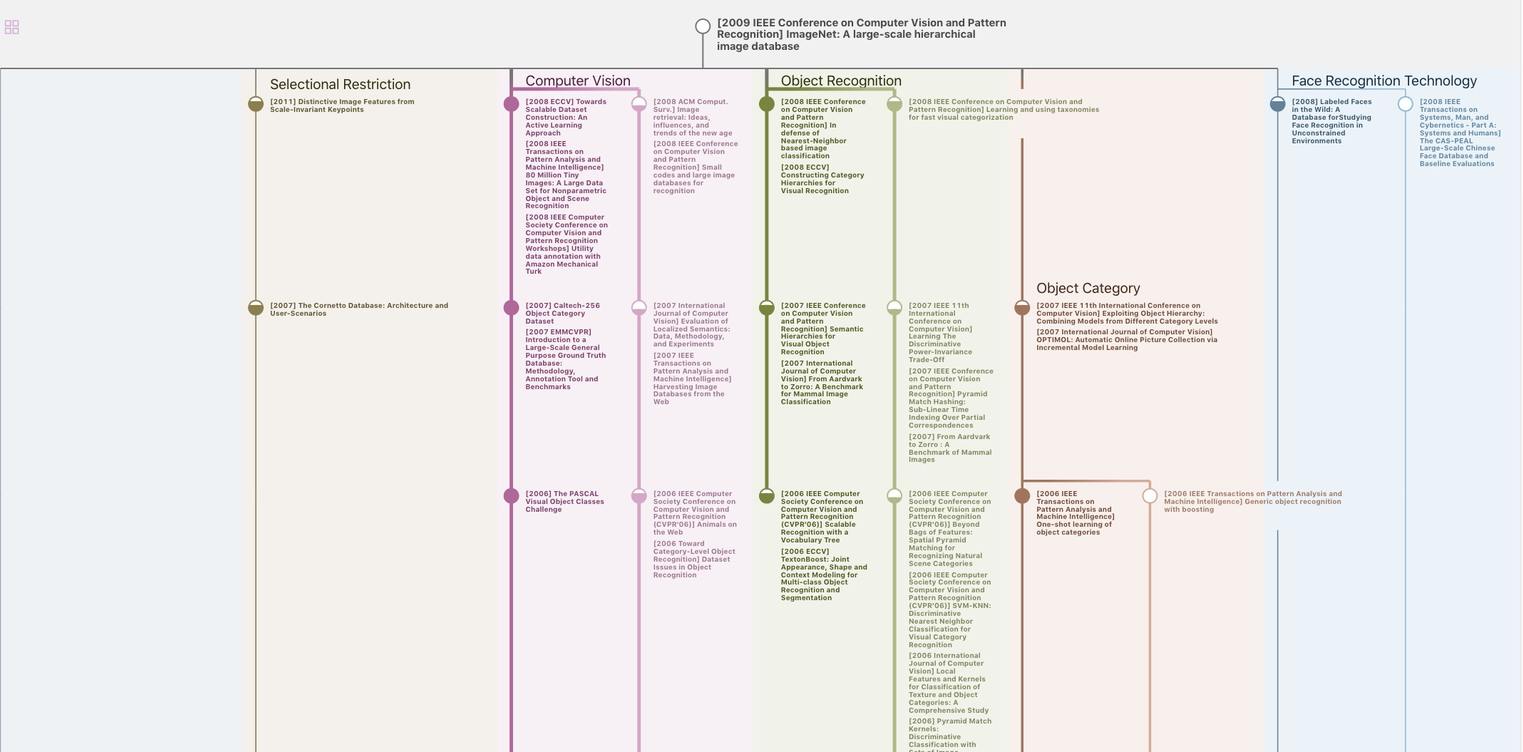
生成溯源树,研究论文发展脉络
Chat Paper
正在生成论文摘要