Online class incremental learning for multi- pose point cloud targets
CHINESE JOURNAL OF LIQUID CRYSTALS AND DISPLAYS(2023)
摘要
In response to current phenomenon that all targets in incremental learning are fixed pose,this paper considers a more rigorous setting, i. e. online class incremental learning for multi-pose targets, which innovatively proposes an ignoring pose replay method to alleviate the catastrophic forgetting in facing multi-pose targets in online class incremental learning. Firstly, 2D/3D targets are point-clouded to facilitate the extraction of useful geometric information. Secondly,the network modifies for equivariance based on the SE ( d)( d = 2,3) group to enable the network to extract richer geometric information,thus reducing the impact of target poses on the model in each task. Finally,specific samples are sampled for replay to mitigate catastrophic forgetting based on loss variation. Experimental results show that when facing fixed posture targets MNIST and CIFAR-10,final average accuracy reaches to 88% and 42. 6% respectively,which is comparable to the comparison method,and final average forgetting is significantly better than the comparison method,with a reduction of about 3% and 15% respectively. In the case of the multi-pose target RotMNIST and trCIFAR-10,the proposed method continues to perform well in fixedpose targets,largely independent of target pose. In addition,the performance in 3D datasets ModelNet40 and trModelNet40 remains stable. The method proposed is able to be independent of the target pose in online class incremental learning, while achieving catastrophic forgetting mitigation, with excellent stability and plasticity.
更多查看译文
关键词
online class-incremental learning,catastrophic forgetting,ignoring pose replay,equivariance,point cloud classification
AI 理解论文
溯源树
样例
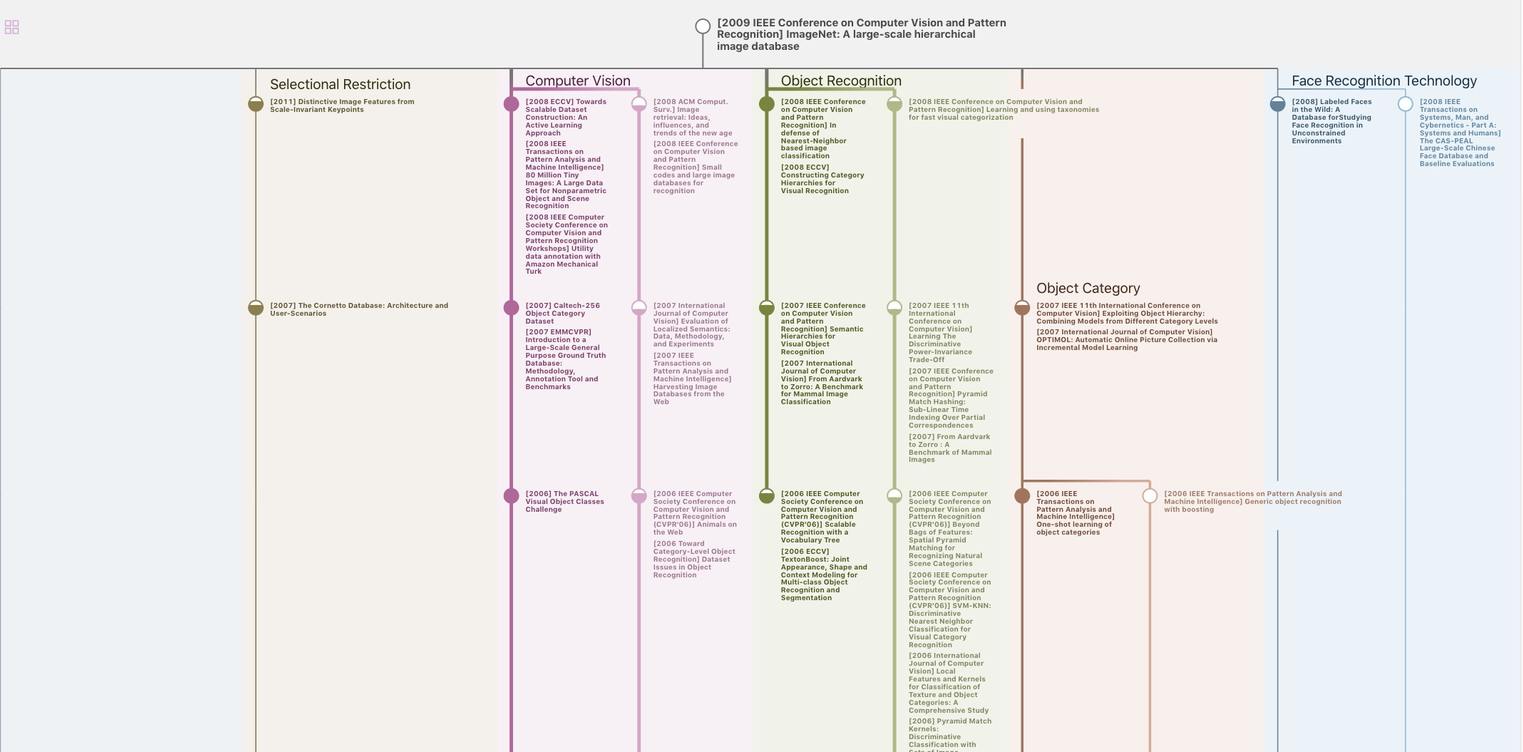
生成溯源树,研究论文发展脉络
Chat Paper
正在生成论文摘要