A Novel Multi-Data-Augmentation and Multi-Deep-Learning Framework for Counting Small Vehicles and Crowds
INTERNATIONAL JOURNAL OF PATTERN RECOGNITION AND ARTIFICIAL INTELLIGENCE(2024)
摘要
Counting small pixel-sized vehicles and crowds in unmanned aerial vehicles (UAV) images is crucial across diverse fields, including geographic information collection, traffic monitoring, item delivery, communication network relay stations, as well as target segmentation, detection, and tracking. This task poses significant challenges due to factors such as varying view angles, non-fixed drone cameras, small object sizes, changing illumination, object occlusion, and image jitter. In this paper, we introduce a novel multi-data-augmentation and multi-deep-learning framework designed for counting small vehicles and crowds in UAV images. The framework harnesses the strengths of specific deep-learning detection models, coupled with the convolutional block attention module and data augmentation techniques. Additionally, we present a new method for detecting cars, motorcycles, and persons with small pixel sizes. Our proposed method undergoes evaluation on the test dataset v2 of the 2022 AI Cup competition, where we secured the first place on the private leaderboard by achieving the highest harmonic mean. Subsequent experimental results demonstrate that our framework outperforms the existing YOLOv7-E6E model. We also conducted comparative experiments using the publicly available VisDrone datasets, and the results show that our model outperforms the other models with the highest AP50 score of 52%.
更多查看译文
关键词
Object segmentation,object tracking,vehicles and crowds counting,UAV images,data augmentation,deep learning
AI 理解论文
溯源树
样例
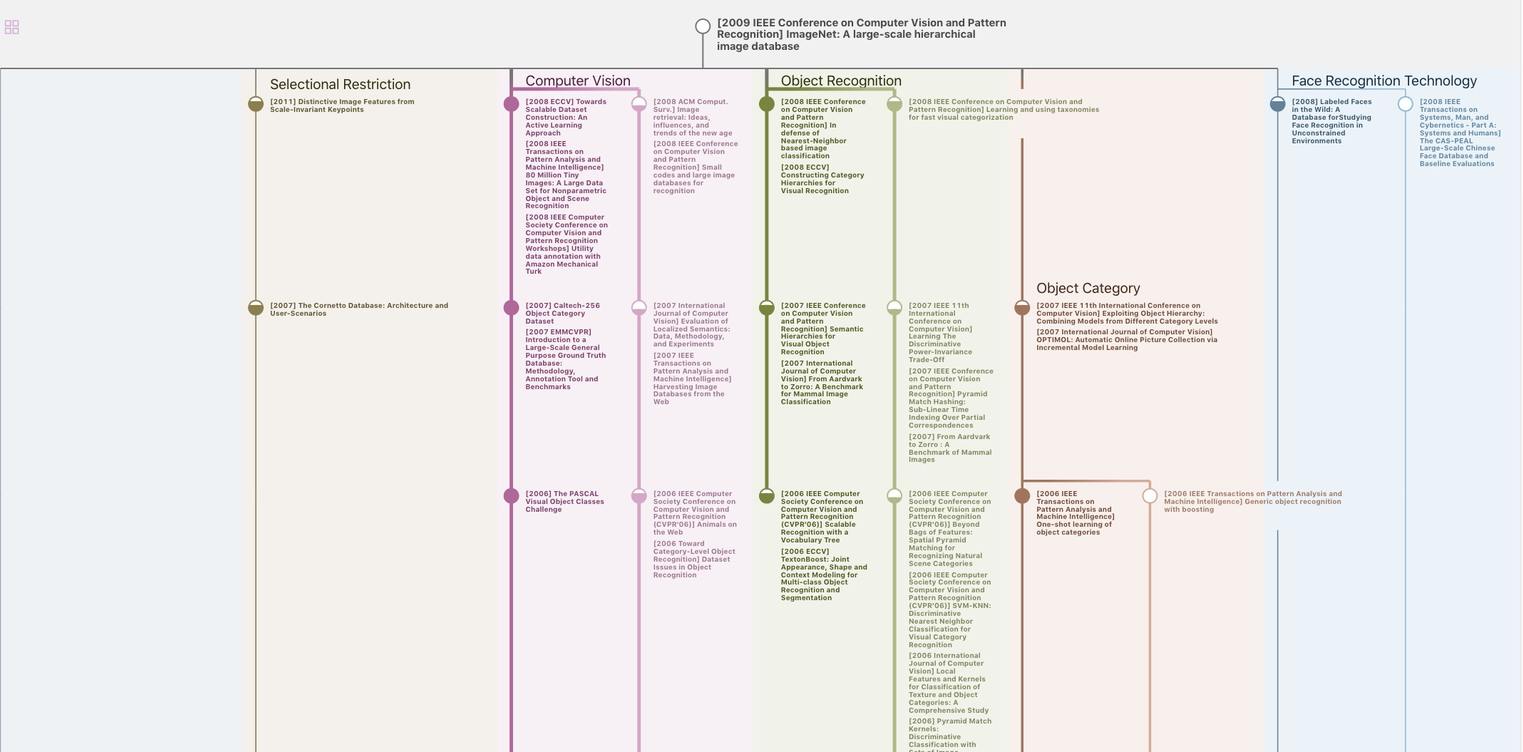
生成溯源树,研究论文发展脉络
Chat Paper
正在生成论文摘要