Length Aggregating intrinsic information to enhance BCI performance through federated learning
NEURAL NETWORKS(2024)
摘要
Insufficient data is a long-standing challenge for Brain-Computer Interface (BCI) to build a high-performance deep learning model. Though numerous research groups and institutes collect a multitude of EEG datasets for the same BCI task, sharing EEG data from multiple sites is still challenging due to the heterogeneity of devices. The significance of this challenge cannot be overstated, given the critical role of data diversity in fostering model robustness. However, existing works rarely discuss this issue, predominantly centering their attention on model training within a single dataset, often in the context of inter -subject or inter -session settings. In this work, we propose a hierarchical personalized Federated Learning EEG decoding (FLEEG) framework to surmount this challenge. This innovative framework heralds a new learning paradigm for BCI, enabling datasets with disparate data formats to collaborate in the model training process. Each client is assigned a specific dataset and trains a hierarchical personalized model to manage diverse data formats and facilitate information exchange. Meanwhile, the server coordinates the training procedure to harness knowledge gleaned from all datasets, thus elevating overall performance. The framework has been evaluated in Motor Imagery (MI) classification with nine EEG datasets collected by different devices but implementing the same MI task. Results demonstrate that the proposed framework can boost classification performance up to 8.4% by enabling knowledge sharing between multiple datasets, especially for smaller datasets. Visualization results also indicate that the proposed framework can empower the local models to put a stable focus on task -related areas, yielding better performance. To the best of our knowledge, this is the first end -to -end solution to address this important challenge.
更多查看译文
关键词
Federated learning,Brain-computer interface,Heterogeneous datasets,Motor imagery
AI 理解论文
溯源树
样例
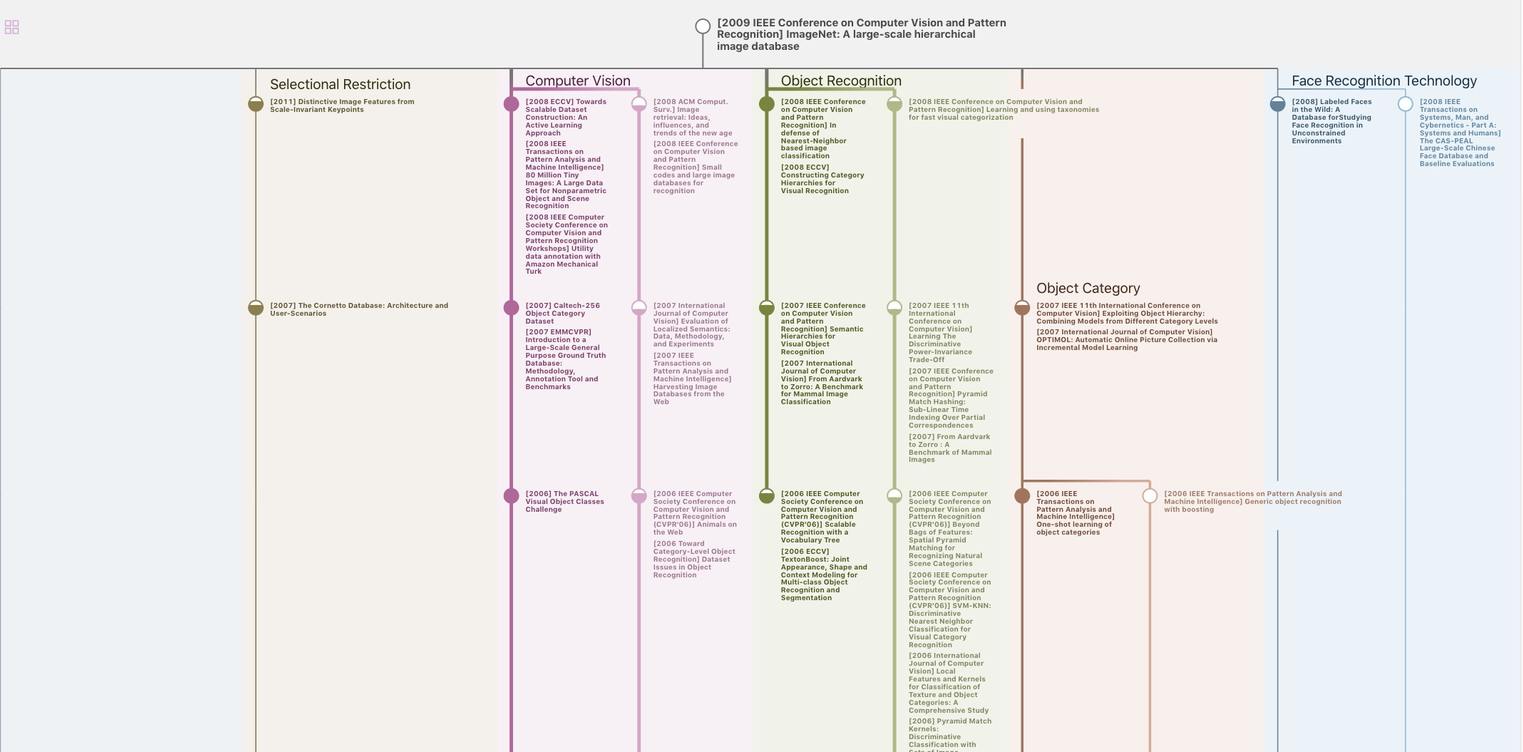
生成溯源树,研究论文发展脉络
Chat Paper
正在生成论文摘要