Ensemble-Based Knowledge Distillation for Video Anomaly Detection
APPLIED SCIENCES-BASEL(2024)
摘要
Video anomaly detection has become a vital task for smart video surveillance systems because of its significant potential to minimize the video data to be analyzed by choosing unusual and critical patterns in the scenes. In this paper, we introduce three novel ensemble and knowledge distillation-based adaptive training methods to handle robust detection of different abnormal patterns in video scenes. Our approach leverages the adaptation process by providing information transfer from multiple teacher models with different network structures and further alleviates the catastrophic forgetting issue. The proposed ensemble knowledge distillation methods are implemented on two state-of-the-art anomaly detection models. We extensively evaluate our methods on two public video anomaly datasets and present a detailed analysis of our results. Finally, we show that not only does our best version model achieve comparable performance with a frame-level AUC of 75.82 to other state-of-the-art models on UCF-Crime as the target dataset, but more importantly our approaches prevent catastrophic forgetting and dramatically improve our model's performance.
更多查看译文
关键词
computer vision,ensemble-based methods,knowledge distillation,video anomaly detection,weak supervision
AI 理解论文
溯源树
样例
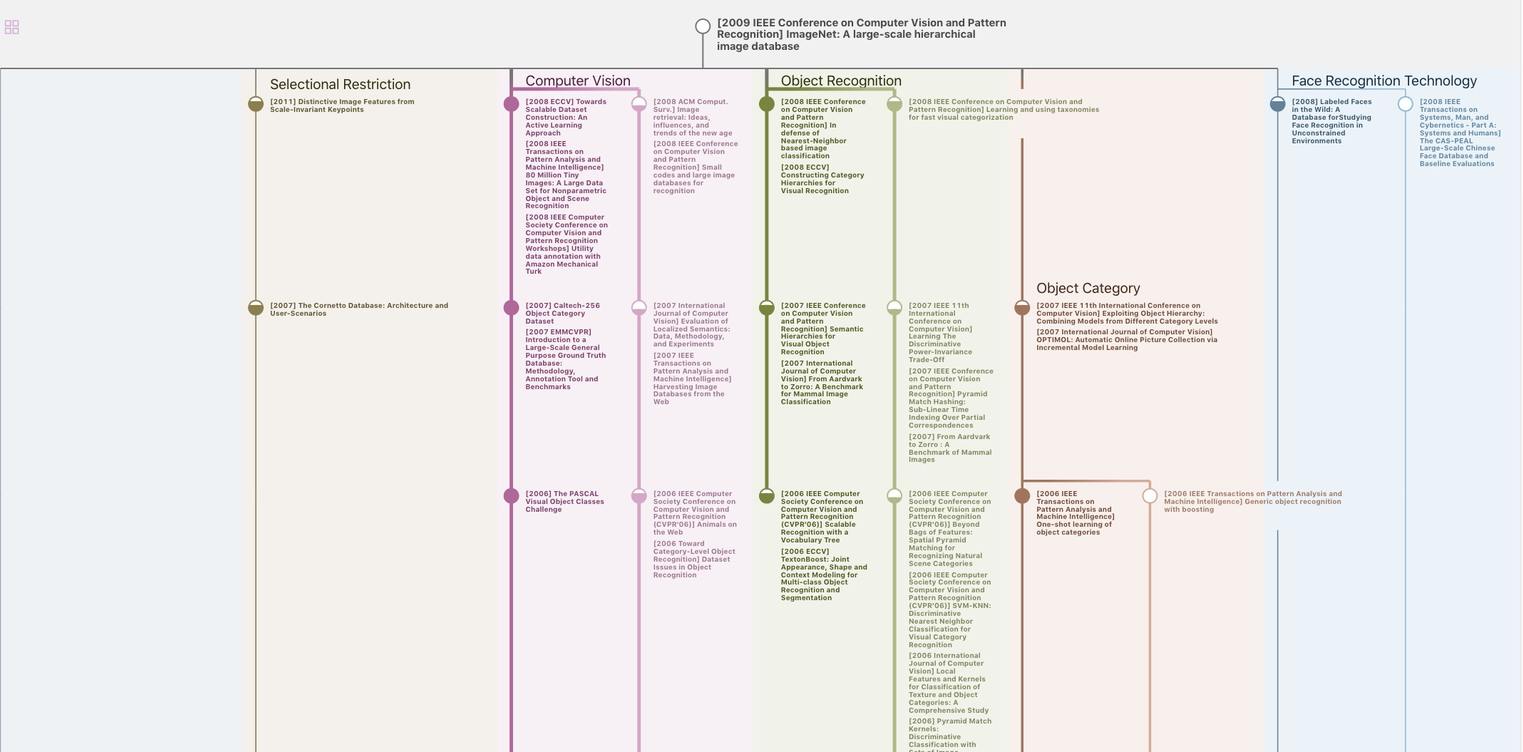
生成溯源树,研究论文发展脉络
Chat Paper
正在生成论文摘要