Bearing Fault Diagnosis based on Convolution Neural Network with Logistic Chaotic Map
ADVANCED THEORY AND SIMULATIONS(2024)
摘要
Bearing is the most basic component of motor, and prone to failure. Bearing fault diagnosis is paramount for improving the reliability and safety in motor-drive systems. Therefore, convolutional neural network (CNN) is proposed with Logistic chaotic map and its corresponding fault diagnosis approach, which can effectively advance the accuracy of bearing fault diagnosis. Specifically, the Logistic chaotic map and Sigmoid function are combined into a non-monotonic excitation function, which is employed to the full connection layer of the CNN. The proposed chaotic CNN can solve two issues that the conventional neural network inclines to get the local minimum value and the gradient of Sigmoid excitation function disappears. It is applied to fault data from the center of Western Reserve University and from the American Society for Mechanical Failure Prevention technology (in noiseless and noisy conditions). The results indicate the diagnosis accuracy of the algorithm outperforms other classical bearing diagnosis algorithms. Moreover, the chaotic CNN exhibits better anti-noise performance. A Convolutional Neural Network (CNN) enhanced with a Logistic chaotic map is proposed, and a novel approach for precise bearing fault diagnosis in motor-drive systems is designed. Results demonstrate superior accuracy compared to classical algorithms, showcasing the chaotic CNN's remarkable anti-noise performance. image
更多查看译文
关键词
chaotic convolutional neural network,chaotic map,excitation function,fault diagnosis
AI 理解论文
溯源树
样例
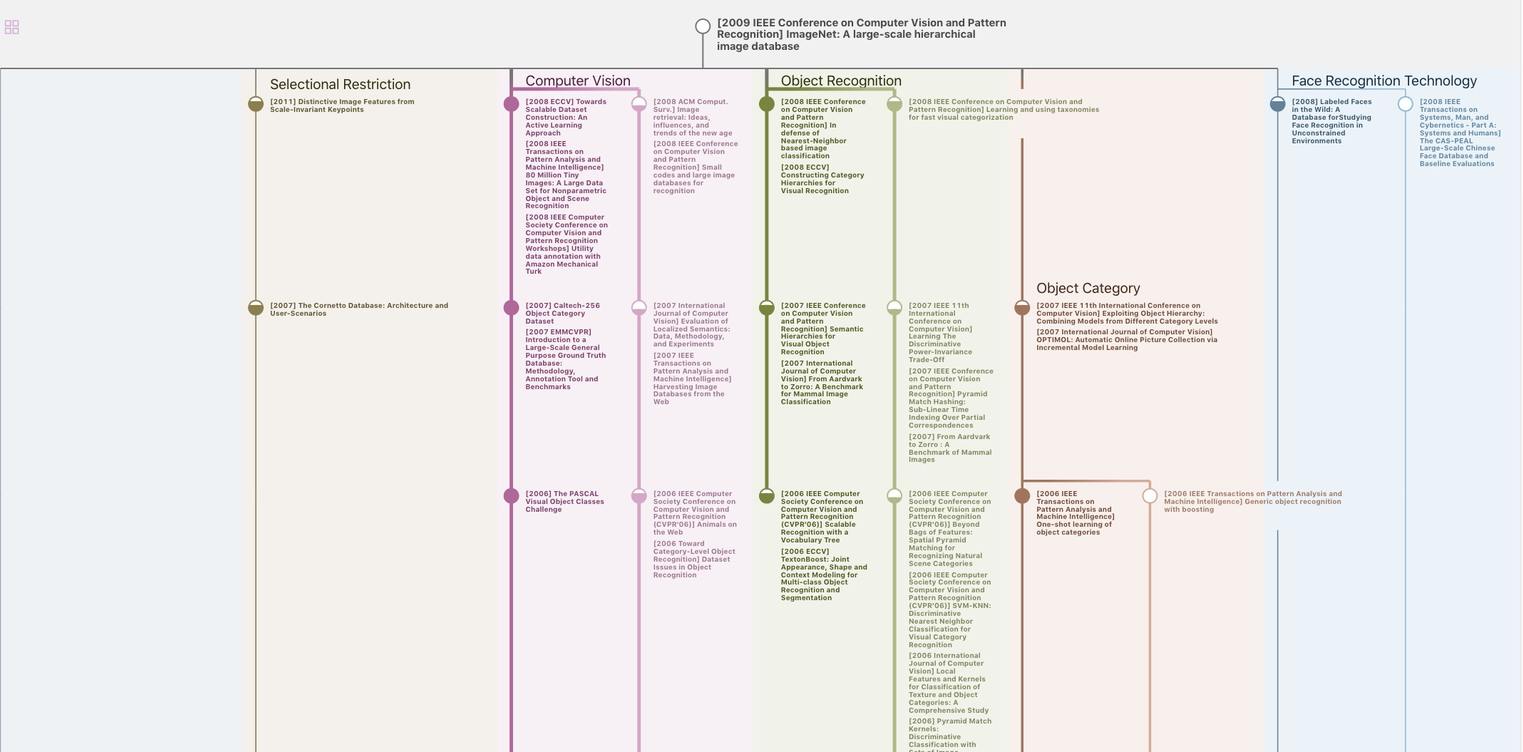
生成溯源树,研究论文发展脉络
Chat Paper
正在生成论文摘要