UCEpic: Unifying Aspect Planning and Lexical Constraints for Generating Explanations in Recommendation
PROCEEDINGS OF THE 29TH ACM SIGKDD CONFERENCE ON KNOWLEDGE DISCOVERY AND DATA MINING, KDD 2023(2023)
摘要
Personalized natural language generation for explainable recommendations plays a key role in justifying why a recommendation might match a user's interests. Existing models usually control the generation process by aspect planning. While promising, these aspect-planning methods struggle to generate specific information correctly, which prevents generated explanations from being convincing. In this paper, we claim that introducing lexical constraints can alleviate the above issues. We propose a model, UCEpic, that generates high-quality personalized explanations for recommendation results by unifying aspect planning and lexical constraints in an insertion-based generation manner. Methodologically, to ensure text generation quality and robustness to various lexical constraints, we pre-train a non-personalized text generator via our proposed robust insertion process. Then, to obtain personalized explanations under this framework of insertion-based generation, we design a method of incorporating aspect planning and personalized references into the insertion process. Hence, UCEpic unifies aspect planning and lexical constraints into one framework and generates explanations for recommendations under different settings. Compared to previous recommendation explanation generators controlled by only aspects, UCEpic incorporates specific information from keyphrases and then largely improves the diversity and informativeness of generated explanations for recommendations on datasets such as RateBeer and Yelp.
更多查看译文
关键词
Recommender Systems,Explainable Recommendation,Natural Language Generation,Lexical Constraints
AI 理解论文
溯源树
样例
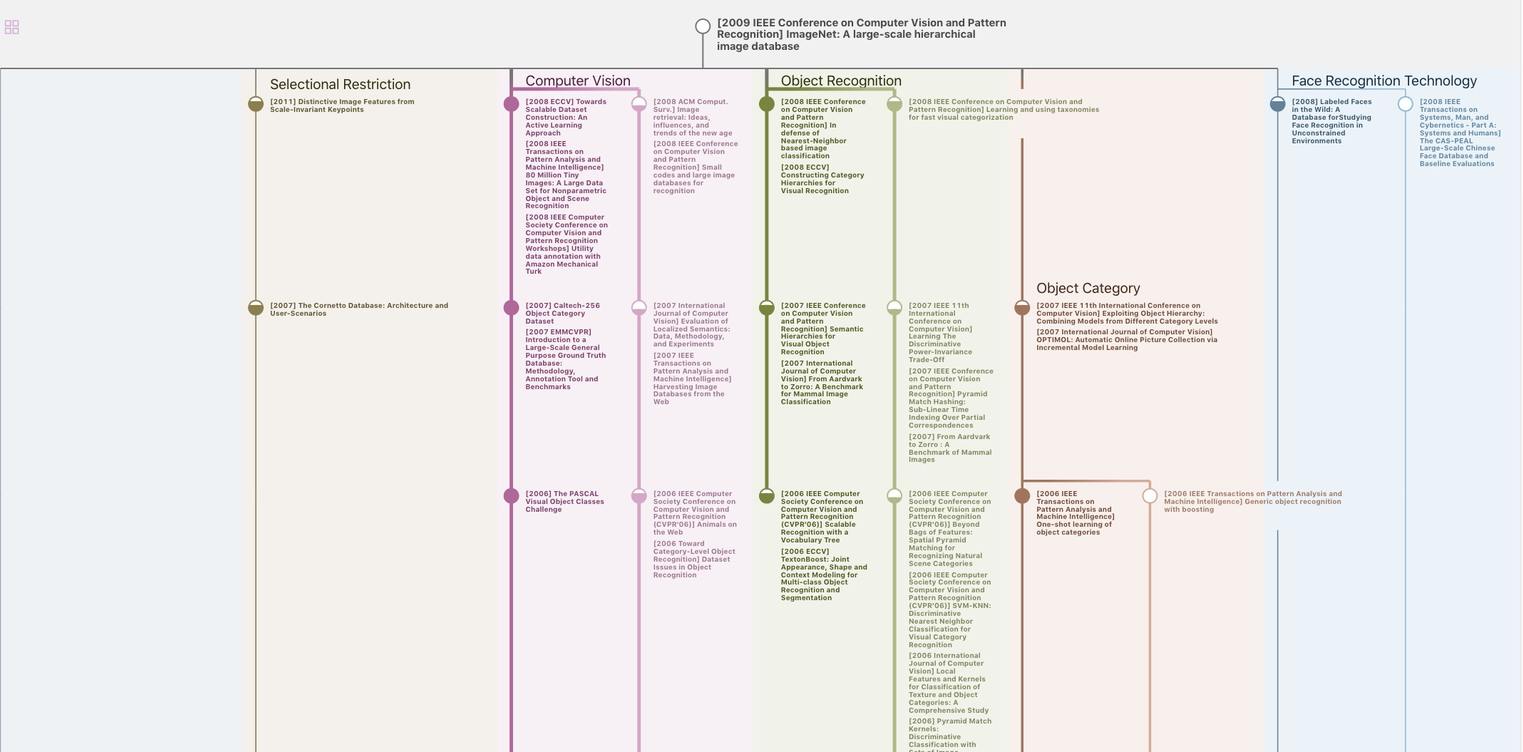
生成溯源树,研究论文发展脉络
Chat Paper
正在生成论文摘要