Sharp optimality for high-dimensional covariance testing under sparse signals
ANNALS OF STATISTICS(2023)
摘要
This paper considers one-sample testing of a high-dimensional covariance matrix by deriving the detection boundary as a function of the signal sparsity and signal strength under the sparse alternative hypotheses. It first shows that the optimal detection boundary for testing sparse means is the minimax detection lower boundary for testing the covariance matrix. A multilevel thresholding test is proposed and is shown to be able to attain the detection lower boundary over a substantial range of the sparsity parameter, implying that the multilevel thresholding test is sharp optimal in the minimax sense over the range. The asymptotic distribution of the multilevel thresholding statistic for covariance matrices is derived under both Gaussian and non-Gaussian distributions by developing a novel U-statistic decomposition in conjunction with the matrix blocking and the coupling techniques to handle the complex dependence among the elements of the sample covariance matrix. The superiority in the detection boundary of the multilevel thresholding test over the existing tests is also demonstrated.
更多查看译文
关键词
Covariance matrix,detection boundary,high dimensionality,minimax,rare and faint,signal,thresholding
AI 理解论文
溯源树
样例
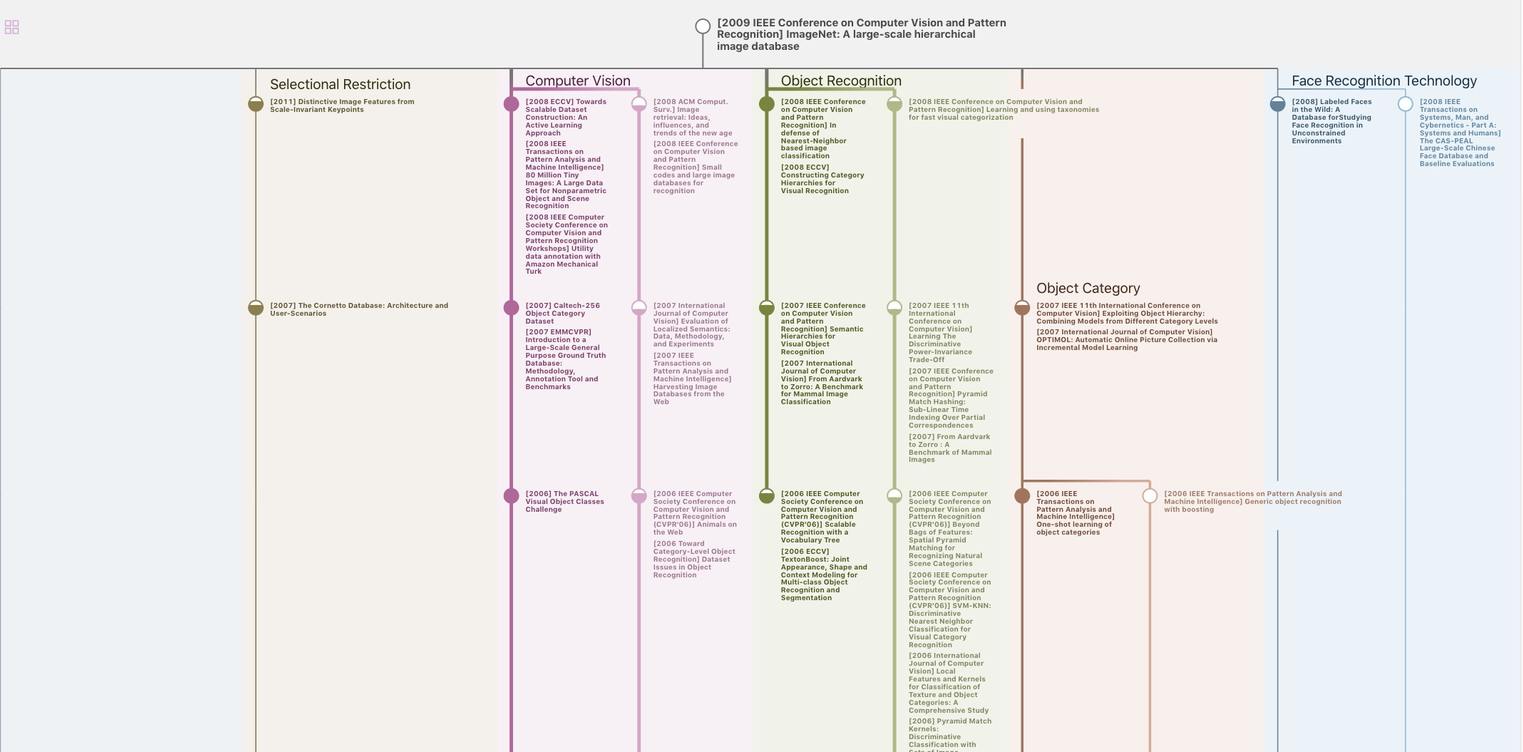
生成溯源树,研究论文发展脉络
Chat Paper
正在生成论文摘要