Reliability Evaluation of Slopes Considering Spatial Variability of Soil Parameters Based on Efficient Surrogate Model
ASCE-ASME JOURNAL OF RISK AND UNCERTAINTY IN ENGINEERING SYSTEMS PART A-CIVIL ENGINEERING(2024)
摘要
The conventional surrogate model for slope reliability assessment often is faced with the issues of high dimensionality and sample selection disorder, which are caused by the spatial variability of soil parameters and which compromise the precision and efficiency of slope reliability assessment. Previous studies focused on solving this problem mainly by choosing more-accurate models; studies of optimizing the training samples for constructing surrogate models are relatively scarce. This paper proposes a multivariate adaptive regression spline model based on active learning (AMARS) for slope reliability analysis in spatially variable soils, combined with the sliced inverse regression (SIR) method. The active learning includes self-supervised learning methods that optimize the sample set for constructing surrogate models. The training samples are processed using the SIR method to prevent the model from falling into dimensionality disaster. The proposed method was validated using two slope cases with spatial variation. Comparison of computational efficiency and accuracy in estimating slope failure probability revealed that the method suggested here outperforms others. Moreover, for both single-layer simple and multilayer complex spatially varying slopes, the proposed method not only reduces computational costs effectively but can also be used to evaluate the reliability of slopes with small failure probabilities.
更多查看译文
关键词
Slope stability,Sliced inverse regression (SIR),Spatial variability,Multivariate adaptive regression splines,Active learning
AI 理解论文
溯源树
样例
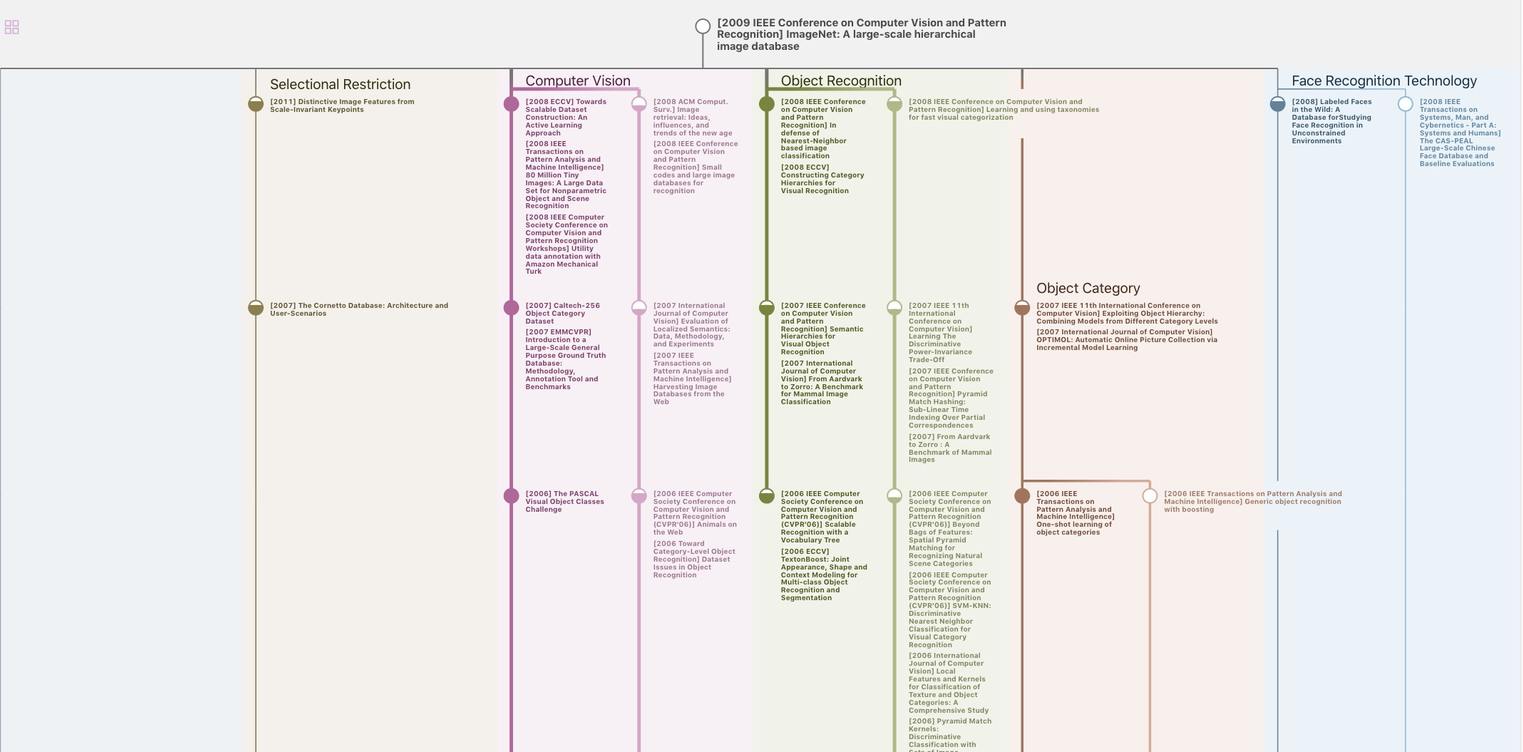
生成溯源树,研究论文发展脉络
Chat Paper
正在生成论文摘要