H2-Nets: Hyper-hodge Convolutional Neural Networks for Time-Series Forecasting
MACHINE LEARNING AND KNOWLEDGE DISCOVERY IN DATABASES: RESEARCH TRACK, ECML PKDD 2023, PT V(2023)
摘要
Hypergraphs recently have emerged as a new promising alternative to describe complex dependencies in spatio-temporal processes, resulting in the newest trend in multivariate time series forecasting, based semi-supervised learning of spatio-temporal data with Hypergraph Convolutional Networks. Nevertheless, such recent approaches are often limited in their capability to accurately describe higher-order interactions among spatio-temporal entities and to learn hidden interrelations among network substructures. Motivated by the emerging results on simplicial convolution, we introduce the concepts of Hodge theory and Hodge Laplacians, that is, a higher-order generalization of the graph Laplacian, to hypergraph learning. Furthermore, we develop a novel framework for hyper-simplex-graph representation learning which describes complex relationships among both graph and hyper-simplex-graph simplices and, as a result, simultaneously extracts latent higher-order spatiotemporal dependencies. We provide theoretical foundations behind the proposed hyper-simplex-graph representation learning and validate our new Hodge-style Hyper-simplex-graph Neural Networks (H-2-Nets) on 7 real world spatio-temporal benchmark datasets. Our experimental results indicate that H-2-Nets outperforms the state-of-the-art methods by a significant margin, while demonstrating lower computational costs.
更多查看译文
关键词
Hypergraph,Higher-order network,Simplicial complex,Spatio-temporal forecasting
AI 理解论文
溯源树
样例
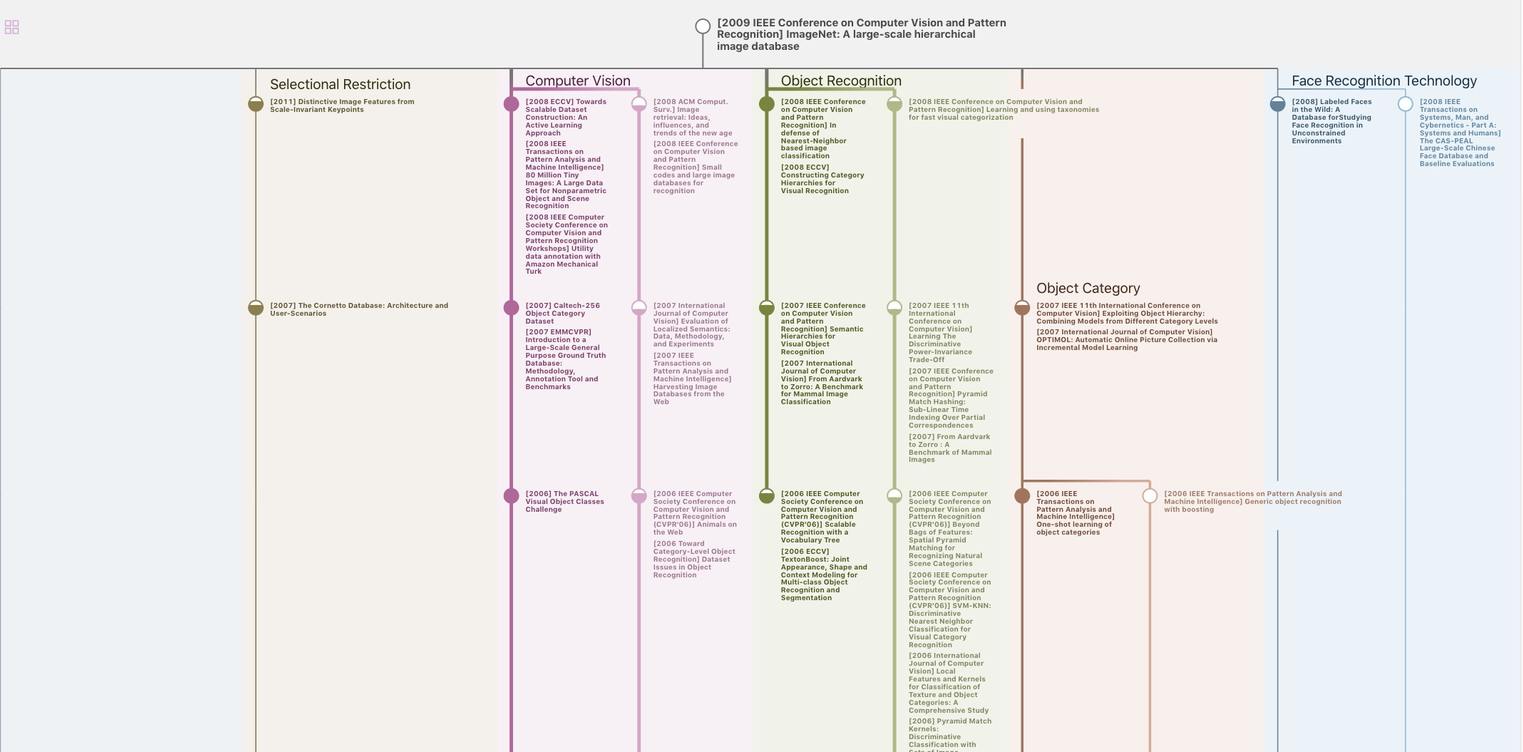
生成溯源树,研究论文发展脉络
Chat Paper
正在生成论文摘要