Depthwise Convolution with Channel Mixer: Rethinking MLP in MetaFormer for Faster and More Accurate Vehicle Detection
ARTIFICIAL NEURAL NETWORKS AND MACHINE LEARNING, ICANN 2023, PART X(2023)
摘要
Vehicle detection is an important task in intelligent traffic monitoring and autonomous driving. However, vehicle detection not only requires fast detection speed, but also presents a challenge due to detection of small targets under occlusion and illumination. Although some current approaches introduce lightweight networks to improve detection speed or Transformer to improve accuracy, they still struggle to achieve an excellent balance between speed and accuracy. To address these issues, this paper proposes a simple yet effective MetaFormerd-based vehicle detection model, which is constructed based on depthwise convolution with channel mixer (DWCM), using depthwise convolution as the token mixer and replacing the MLP residual block with a channel mixer (CM) module to boost feature reuse. The DWCM module enables enhanced feature extraction capabilities and can be applied to different detection models to improve vehicle detection performance. In extensive experiments, the proposed model not only achieves 38.0% AP on MS COCO, but also achieves 64.2% AP50 and 45.8% AP on UA-DETRAC with 278FPS on a RTX 2080Ti, which is significantly faster and more accurate than the state-of-the-art YOLO detectors without bells and whistles. Furthermore, the proposed CM-based model is more promising than the MLP-based model for vehicle detection which shows the superior potential of CM structure.
更多查看译文
关键词
Vehicle Detection,MetaFormer,Channel Mixer,YOLO
AI 理解论文
溯源树
样例
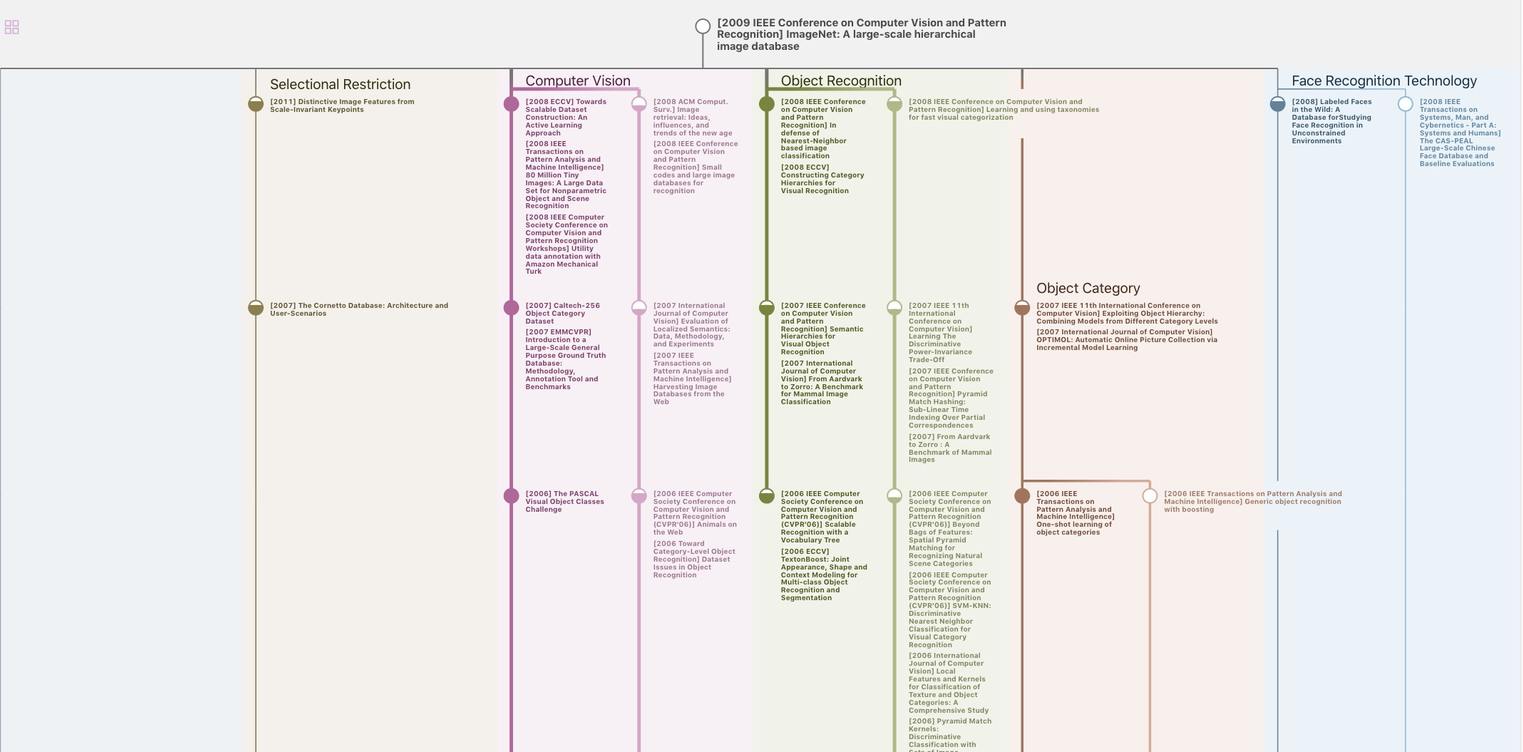
生成溯源树,研究论文发展脉络
Chat Paper
正在生成论文摘要