Shale porosity prediction based on random forest algorithm
Youqi dizhi yu caishoulu(2023)
摘要
Precise and fast acquisition of shale porosity is important for the prediction of the spatial distribution of shale oil and the exploration target. To address the problem of low accuracy of porosity prediction using logging response equation, a porosity prediction model based on random forest algorithm is established, and the prediction accuracy is compared with those of BP neural network, support vector machine, and XGBoost algorithm, and the importance and influence range of logging parameters are analyzed by SHAP method. The results show that the random forest algorithm can better predict shale porosity, and the prediction effect is better than BP neural network, support vector machine, and XGBoost algorithm; the application of shale porosity prediction based on random forest algorithm in a depression in Bohai Bay Basin finds that the top three most important logging parameters for model prediction of porosity are compensation neutron, natural gamma, and ordinary apparent resistivity; the shale porosity prediction model based on random forest algorithm can quickly identify the porosity of a single well, which can not only compensate for the difficulty of obtaining the complete porosity distribution characteristics due to the inability of continuous coring but also significantly improve the efficiency and accuracy of porosity prediction.
更多查看译文
关键词
random forest,machine learning,logging,porosity prediction,shale
AI 理解论文
溯源树
样例
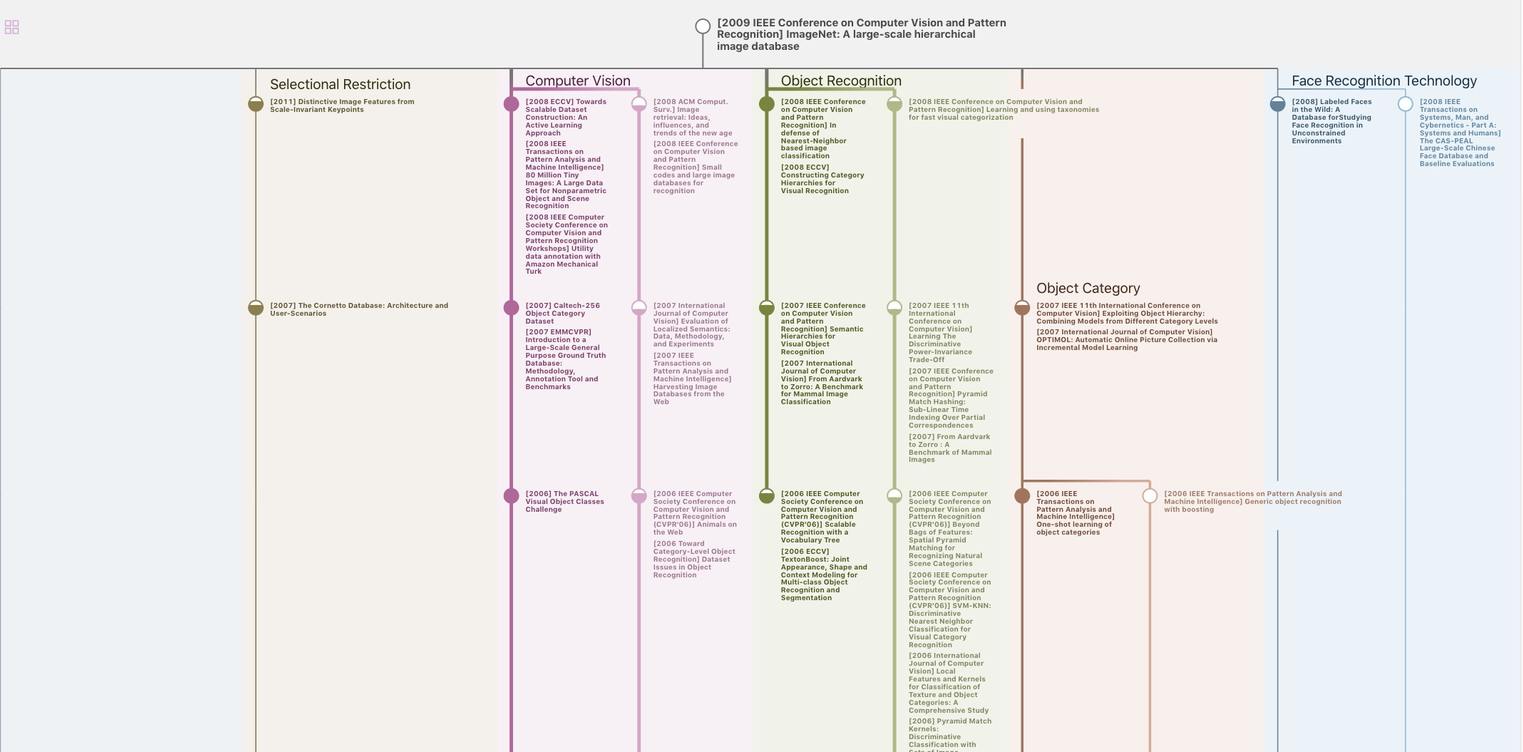
生成溯源树,研究论文发展脉络
Chat Paper
正在生成论文摘要