Relative vectoring using dual object detection for autonomous aerial refueling
Neural Computing and Applications(2024)
摘要
Once realized, autonomous aerial refueling will revolutionize unmanned aviation by removing current range and endurance limitations. Previous attempts at establishing vision-based solutions have come close but rely heavily on near perfect extrinsic camera calibrations that often change midflight. In this paper, we propose dual object detection, a technique that overcomes such requirement by transforming aerial refueling imagery directly into receiver aircraft reference frame probe-to-drogue vectors regardless of camera position and orientation. These vectors are precisely what autonomous agents need to successfully maneuver the tanker and receiver aircraft in synchronous flight during refueling operations. Our method follows a common 4-stage process of capturing an image, finding 2D points in the image, matching those points to 3D object features, and analytically solving for the object pose. However, we extend this pipeline by simultaneously performing these operations across two objects instead of one using machine learning and add a fifth stage that transforms the two pose estimates into a relative vector. Furthermore, we propose a novel supervised learning method using bounding box corrections such that our trained artificial neural networks can accurately predict 2D image points corresponding to known 3D object points. Simulation results show that this method is reliable, accurate (within 3 cm at contact), and fast (45.5 fps).
更多查看译文
关键词
YOLO,Perspective-n-point,Relative navigation,Computer vision,Machine learning,Software simulations
AI 理解论文
溯源树
样例
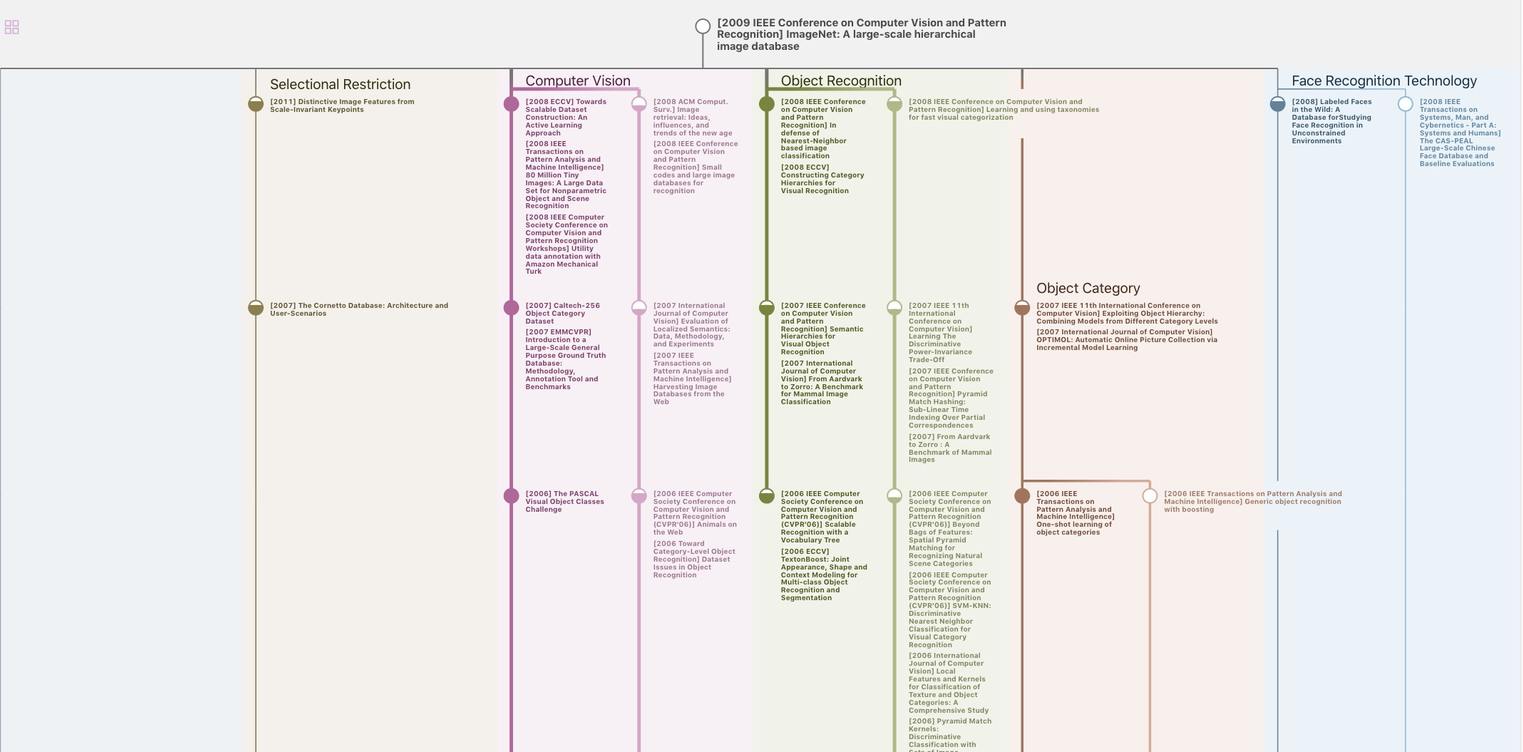
生成溯源树,研究论文发展脉络
Chat Paper
正在生成论文摘要