Eigen Is All You Need: Efficient Lidar-Inertial Continuous-Time Odometry with Internal Association.
CoRR(2024)
摘要
In this paper, we propose a continuous-time lidar-inertial odometry (CT-LIO) system named SLICT2, which promotes two main insights. One, contrary to conventional wisdom, CT-LIO algorithm can be optimized by linear solvers in only a few iterations, which is more efficient than commonly used nonlinear solvers. Two, CT-LIO benefits more from the correct association than the number of iterations. Based on these ideas, we implement our method with a customized solver where the feature association process is performed immediately after each incremental step, and the solution can converge within a few iterations. Our implementation can achieve real-time performance with a high density of control points while yielding competitive performance in highly dynamical motion scenarios. We demonstrate the advantages of our method by comparing with other existing state-of-the-art CT-LIO methods. For the benefits of the community, the source code will be released at
https://github.com/brytsknguyen/slict
.
更多查看译文
关键词
SLAM,Lidar-Inertial Odometry and Mapping,Continuous-time Optimization,Nonlinear Solver
AI 理解论文
溯源树
样例
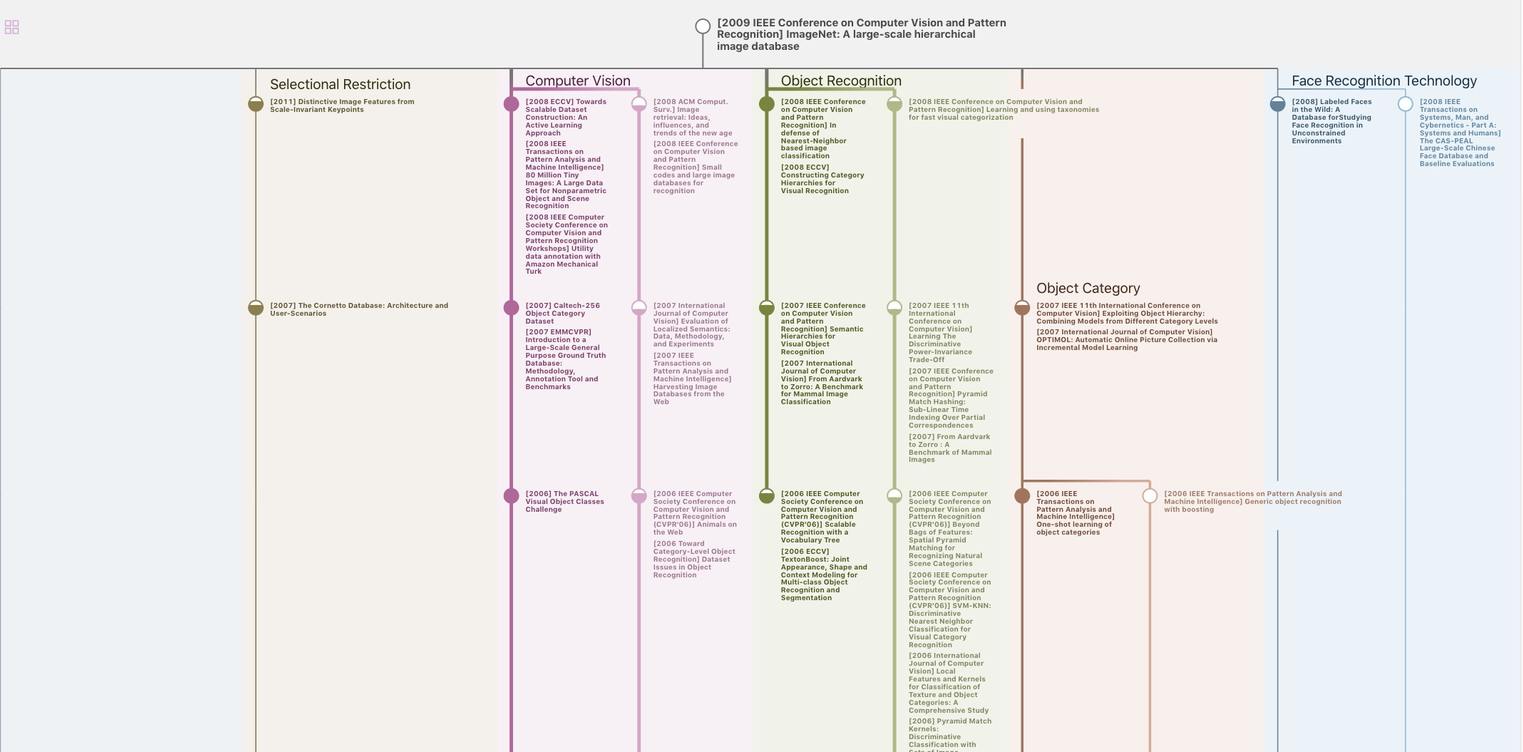
生成溯源树,研究论文发展脉络
Chat Paper
正在生成论文摘要