A Multiagent Meta-Based Task Offloading Strategy for Mobile-Edge Computing
IEEE TRANSACTIONS ON COGNITIVE AND DEVELOPMENTAL SYSTEMS(2024)
摘要
Task offloading in mobile-edge computing (MEC) improves the efficacy of mobile devices (MDs) in terms of computing performance, data storage, and energy consumption by offloading computational tasks to edge servers. Efficient task offloading can leverage MEC technology to reduce task processing latency and energy consumption. By integrating the reasoning ability and machine intelligence of the cognitive computing architecture, such as SOAR and ACT-R, reinforcement learning (RL) algorithms have been applied to resolve the task offloading in MEC. To solve the problem that conventional deep RL (DRL) algorithms cannot adapt to dynamic environments, this article proposed a task offloading scheduling strategy which combined multiagent RL and meta-learning. In order to make the two actions of charging time and offloading strategy fully considered at the same time, we implemented a learning network of two agents on an MD. To efficiently train the policy network, we proposed a first-order approximation method based on the clipped surrogate objective. Finally, the experiments are designed with a variety of the number of subtasks, transmission rate, and edge server performance, and the results show that the MRL-based strategy has the overwhelming overall performance and can be quickly applied in various environments with good stability and generalization.
更多查看译文
关键词
Task analysis,Heuristic algorithms,Servers,Energy consumption,Mobile handsets,Computational modeling,Resource management,Deep reinforcement learning (DRL),edge task offloading,meta-learning,multiagent
AI 理解论文
溯源树
样例
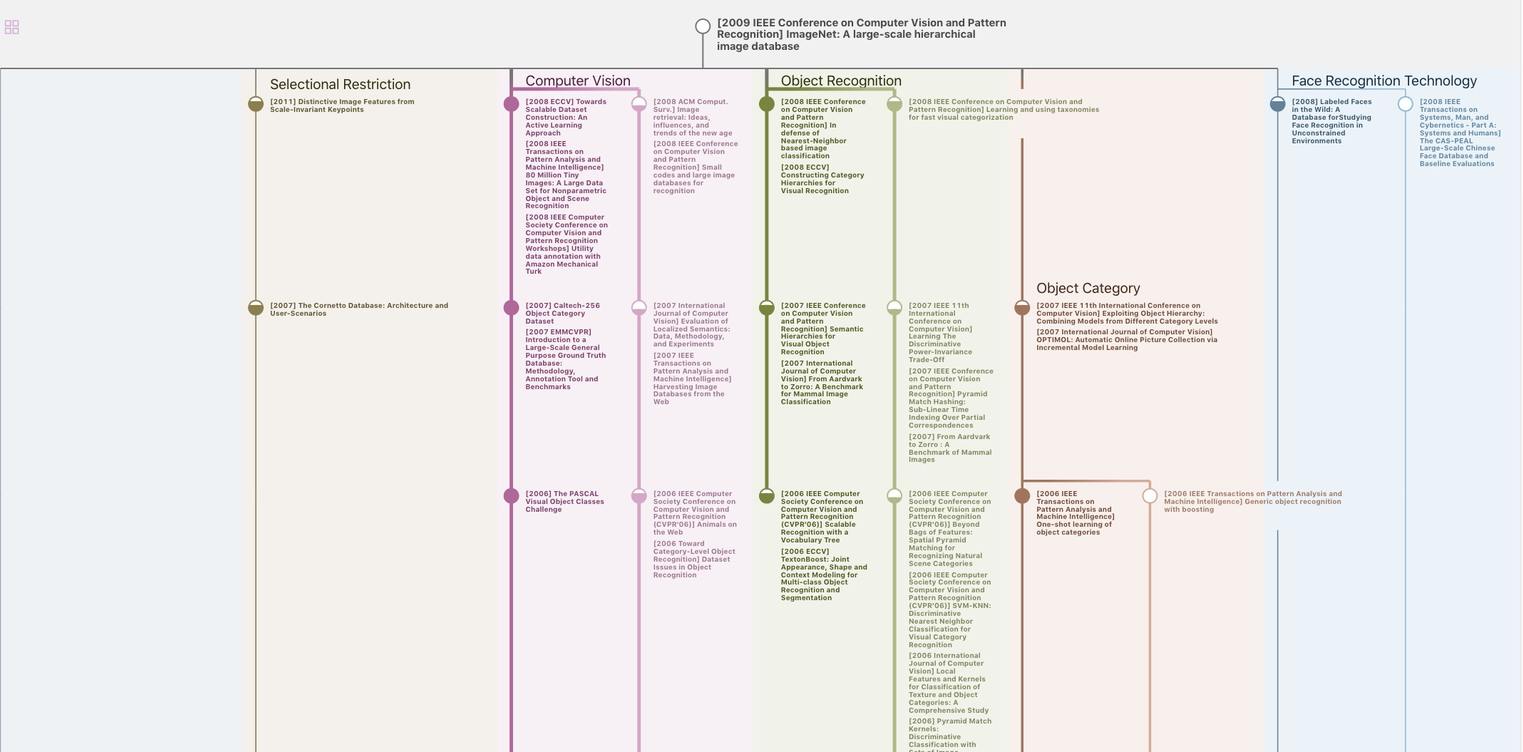
生成溯源树,研究论文发展脉络
Chat Paper
正在生成论文摘要