Optimal scheduling of entropy regularizer for continuous-time linear-quadratic reinforcement learning
SIAM JOURNAL ON CONTROL AND OPTIMIZATION(2024)
摘要
This work uses the entropy -regularized relaxed stochastic control perspective as a principled framework for designing reinforcement learning (RL) algorithms. Herein, an agent interacts with the environment by generating noisy controls distributed according to the optimal relaxed policy. The noisy policies, on the one hand, explore the space and hence facilitate learning, but, on the other hand, they introduce bias by assigning a positive probability to nonoptimal actions. This exploration -exploitation trade-off is determined by the strength of entropy regularization. We study algorithms resulting from two entropy regularization formulations: the exploratory control approach, where entropy is added to the cost objective, and the proximal policy update approach, where entropy penalizes policy divergence between consecutive episodes. We focus on the finite horizon continuous -time linear -quadratic (LQ) RL problem, where a linear dynamics with unknown drift coefficients is controlled subject to quadratic costs. In this setting, both algorithms yield a Gaussian relaxed policy. We quantify the precise difference between the value functions of a Gaussian policy and its noisy evaluation and show that the execution noise must be independent across time. By tuning the frequency of sampling from relaxed policies and the parameter governing the strength of entropy regularization, we prove that the regret, for both learning algorithms, is of p the order O( root N) (up to a logarithmic factor) over N episodes, matching the best known result from the literature.
更多查看译文
关键词
continuous-time reinforcement learning,linear-quadratic,entropy regularization,exploratory control,proximal policy update,regret analysis
AI 理解论文
溯源树
样例
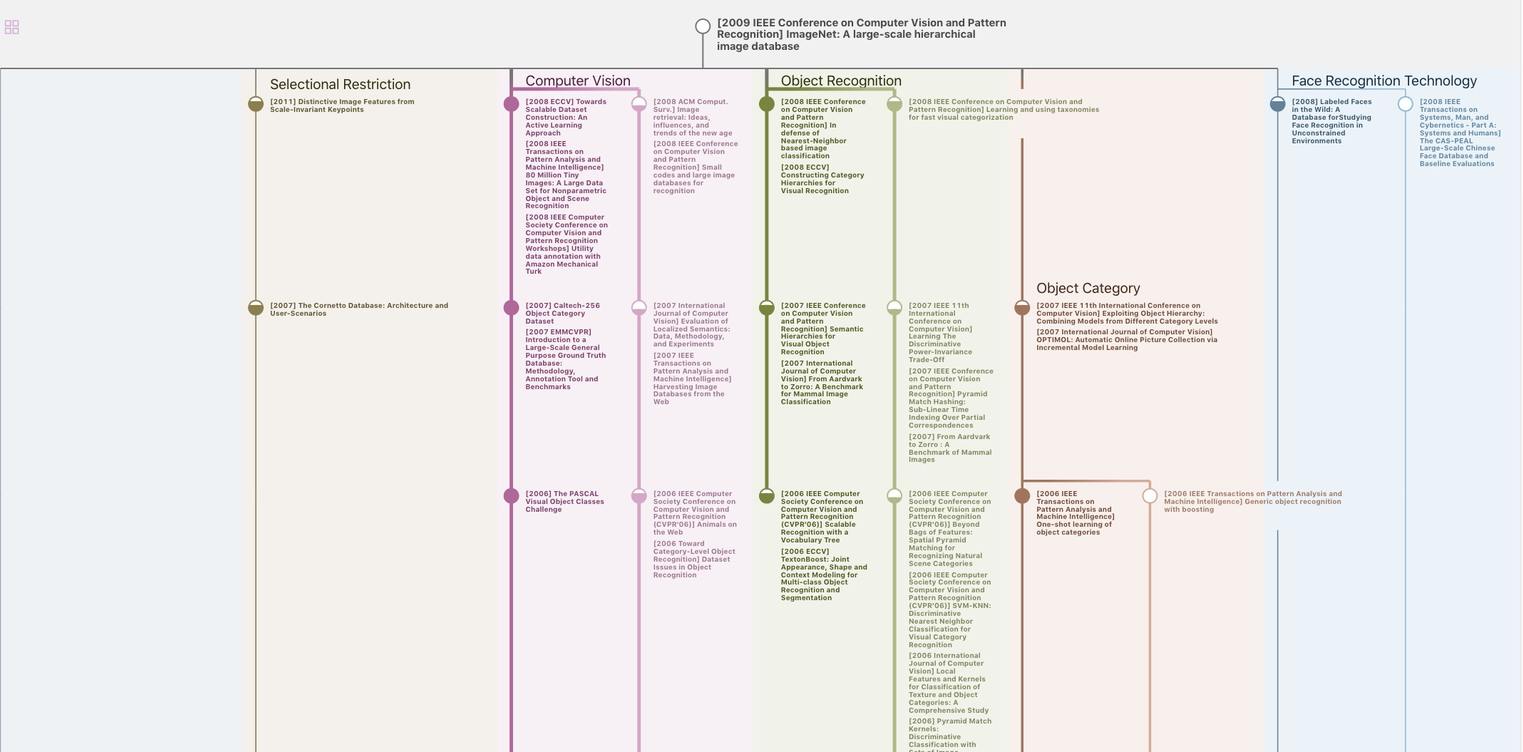
生成溯源树,研究论文发展脉络
Chat Paper
正在生成论文摘要