Identifying Pareto Fronts Reliably Using a Multistage Reference-Vector-Based Framework
IEEE TRANSACTIONS ON EVOLUTIONARY COMPUTATION(2024)
摘要
Evolutionary multiobjective and many-objective optimization (EMO and EMaO) algorithms are increasingly used to identify the true shape and location of the Pareto-optimal front using a few representative well-converged and well-distributed solutions. The reason for their popularity is due to their ability to provide a better understanding of objective relationships for optimal solutions, and also to facilitate the choice of a preferred solution using an interactive or post-optimal multicriterion decision analysis. However, since EMO and EMaO algorithms are stochastic, a single application may not provide a true representative set with a desired number of Pareto solutions reliably in repetitive runs and importantly with a well-distributed set of solutions. In this article, we propose a multistage framework involving reference-vector-based evolutionary multi- and many-objective algorithms (MuSt-EMO and MuSt-EMaO) that attempts to recursively rectify shortcomings of previous stages by careful executions of subsequent stages so that a prescribed number of well-distributed and well-converged solutions are achieved at the end. The proposed multistage approach is implemented to a number of popular reference vector-based EMO/EMaO algorithms and is applied on various multi- and many-objective test and real-world problems.
更多查看译文
关键词
Convergence,Statistics,Sociology,Optimization,Reliability,Heuristic algorithms,Evolutionary computation,Evolutionary algorithms (EAs),gap-filling method,multiobjective optimization,pareto front
AI 理解论文
溯源树
样例
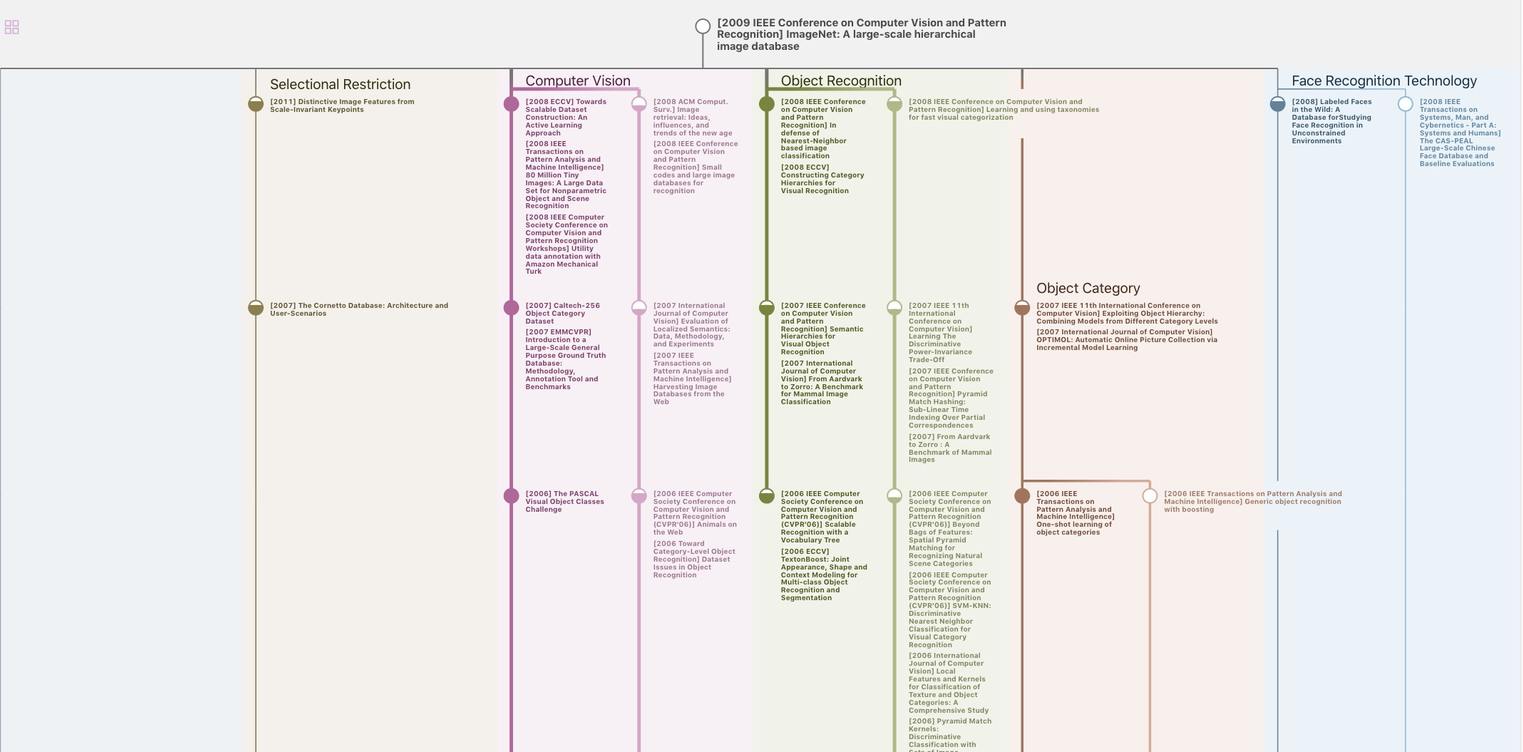
生成溯源树,研究论文发展脉络
Chat Paper
正在生成论文摘要