Text-Free Controllable 3-D Point Cloud Generation
IEEE TRANSACTIONS ON INSTRUMENTATION AND MEASUREMENT(2024)
摘要
Generating 3-D shapes with text inputs has long been a peculiar challenge in computer vision, which requires methodological know-how as well as a sense of art. Recently, text-to-image generation has driven remarkable progress, raising tremendous interest in text-guided shape generation, which further paves the way for industrial design. Nevertheless, prior efforts on text-guided 3-D synthesis either lack geometric details, are limited by the simple text input, or need expensive optimization and additional postprocessing, which make them unfriendly for novices. In this research, we present TFCNet, a novel approach for text-free controllable point cloud generation. In the training phase, we first design an empirically robust cross-modal skeletal point generator (CM-SPG) to predict skeletal points of the specific shape conditioned on the single image input. Then, we develop a diffusion-based dense point generator, which takes skeletal points as geometric guidance to produce dense point clouds that are faithful to the input images. In the inference phase, we propose an efficient text-free nonparametric transfer regime, which does not require separate training and can directly generate point cloud shapes while being semantically faithful to the provided text input. As evidenced by our experiments on the ShapeNet(v2) and CO3D datasets, our proposed method outperforms existing state of-the-art methods both quantitatively and qualitatively.
更多查看译文
关键词
3-D point cloud,text-free controllable point cloud generation,text-guided 3-D generative modeling
AI 理解论文
溯源树
样例
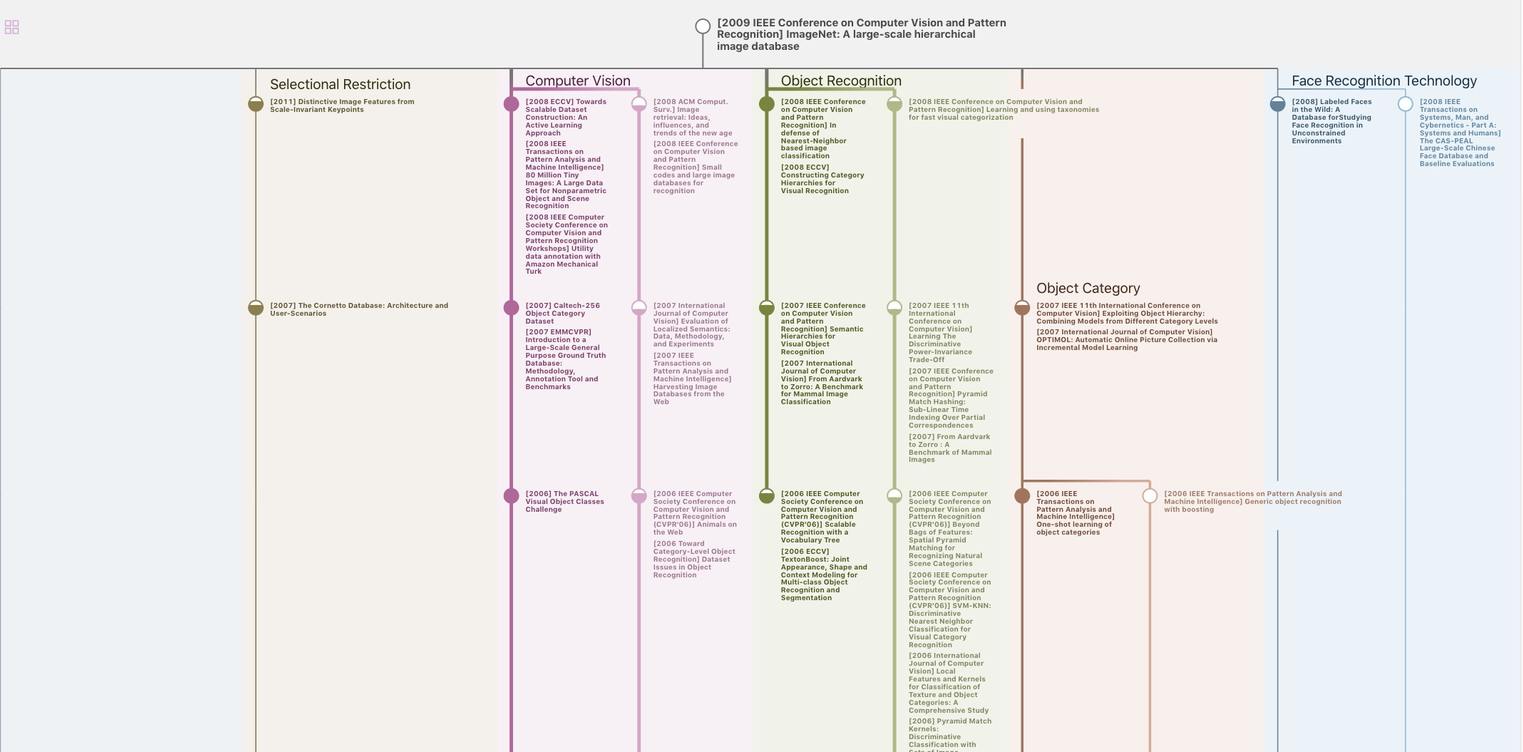
生成溯源树,研究论文发展脉络
Chat Paper
正在生成论文摘要