Dynamic Weighted Adversarial Learning for Semi-Supervised Classification under Intersectional Class Mismatch
ACM TRANSACTIONS ON MULTIMEDIA COMPUTING COMMUNICATIONS AND APPLICATIONS(2024)
摘要
Nowadays, class-mismatch problem has drawn intensive attention in Semi-Supervised Learning (SSL), where the classes of labeled data are assumed to be only a subset of the classes of unlabeled data. However, in a more realistic scenario, the labeled data and unlabeled data often share some common classes while they also have their individual classes, which leads to an "intersectional class-mismatch" problem. As a result, existing SSL methods are often confused by these individual classes and suffer from performance degradation. To address this problem, we propose a novel Dynamic Weighted Adversarial Learning (DWAL) framework to properly utilize unlabeled data for boosting the SSL performance. Specifically, to handle the influence of the individual classes in unlabeled data (i.e., Out-Of-Distribution classes), we propose an enhanced adversarial domain adaptation to dynamically assign weight for each unlabeled example from the perspectives of domain adaptation and a class-wise weighting mechanism, which consists of transferability score and prediction confidence value. Besides, to handle the influence of the individual classes in labeled data (i.e., private classes), we propose a dissimilarity maximization strategy to suppress the inaccurate correlations caused by the examples of individual classes within labeled data. Therefore, our DWAL can properly make use of unlabeled data to acquire an accurate SSL classifier under intersectional class-mismatch setting, and extensive experimental results on five public datasets demonstrate the effectiveness of the proposed model over other state-of-the-art SSL methods.
更多查看译文
关键词
Semi-supervised learning,intersectional class mismatch,adversarial domain adaptation,dissimilarity maximization
AI 理解论文
溯源树
样例
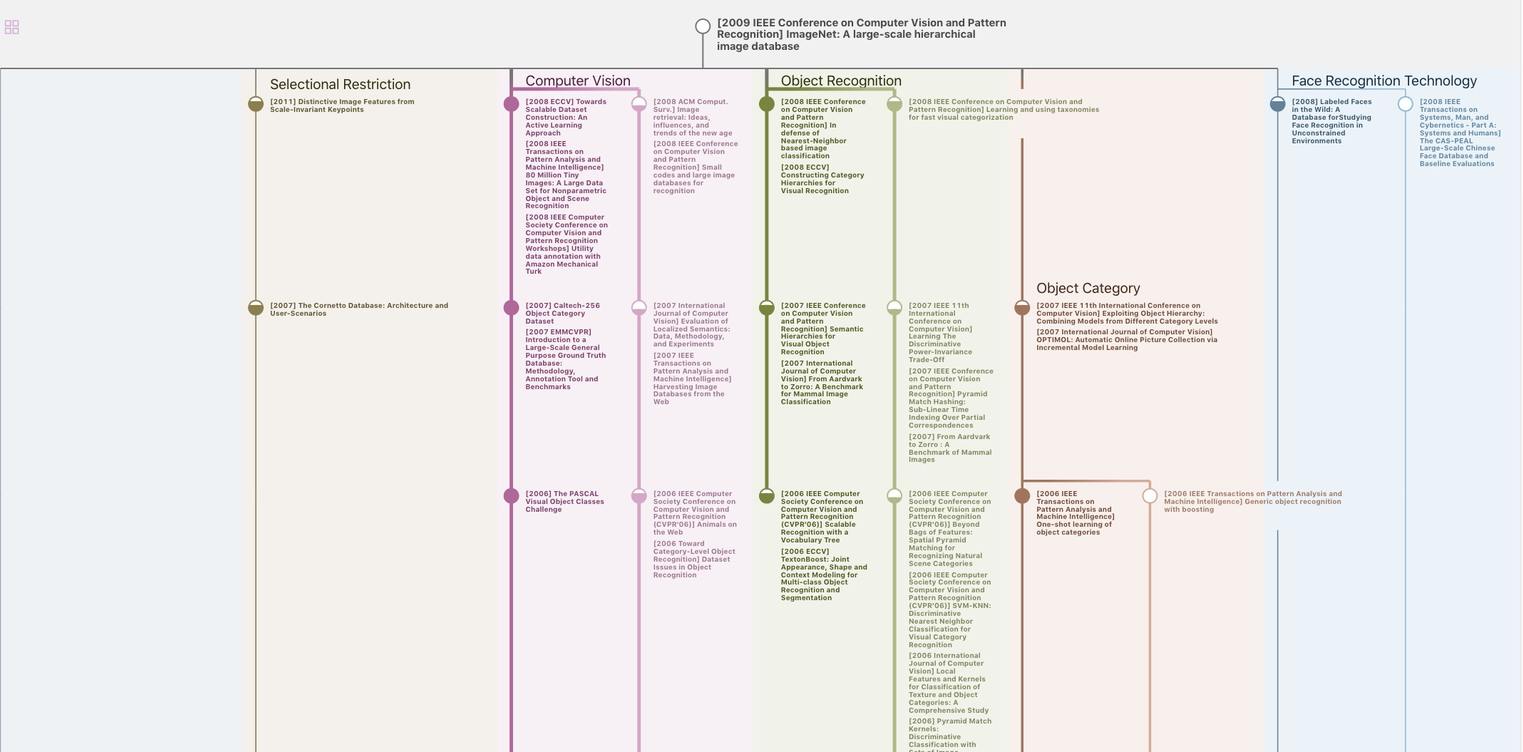
生成溯源树,研究论文发展脉络
Chat Paper
正在生成论文摘要