Perturbation and inverse problems of stochastic matrices
SIAM JOURNAL ON MATRIX ANALYSIS AND APPLICATIONS(2024)
摘要
It is a classical task in perturbation analysis to find norm bounds on the effect of a perturbation \Delta of a stochastic matrix G to its stationary distribution, i.e., to the unique normalized left Perron eigenvector. A common assumption is to consider \Delta to be given and to find bounds on its impact, but in this paper, we rather focus on an inverse optimization problem called the target stationary distribution problem (TSDP). The starting point is a target stationary distribution, and we search for a perturbation \Delta of the minimum norm such that G+ \Delta remains stochastic and has the desired target stationary distribution. It is shown that TSDP has relevant applications in the design of, for example, road networks, social networks, hyperlink networks, and queuing systems. The key to our approach is that we work with rank-1 perturbations. Building on those results for rank-1 perturbations, we provide heuristics for the TSDP that construct arbitrary rank perturbations as sums of appropriately constructed rank-1 perturbations.
更多查看译文
关键词
Key words. Markov chains,perturbation analysis,inverse problems,target stationary distribu- tion problem
AI 理解论文
溯源树
样例
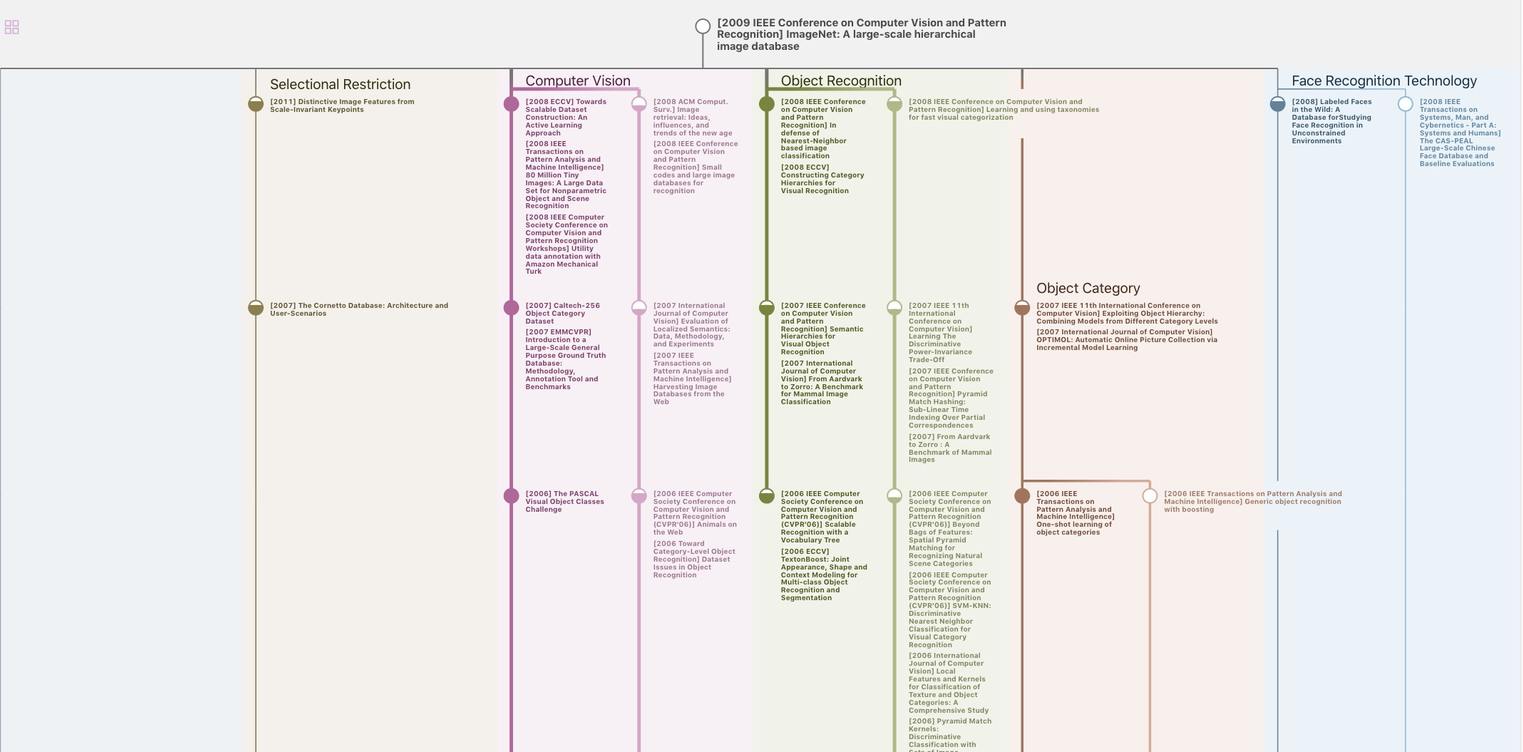
生成溯源树,研究论文发展脉络
Chat Paper
正在生成论文摘要