MRI-CE: Minimal rare itemset discovery using the cross-entropy method
Information Sciences(2024)
摘要
Rare itemsets Image 1 studied Image 2 than frequent itemsets, Image 3 have Image 4 potential applications in black swan events, Image 5. Mining rare itemsets poses two challenges: too many results Image 6, and Image 7 a high computational overhead. To overcome these two challenges, we Image 8 mine minimal rare itemsets (MRIs) and use heuristic methods to mine approximate results instead of exact results. This paper describes a novel algorithm for mining MRIs using cross-entropy (CE). We present the modeling method for MRI-CE and introduce a progressive checking strategy that enables more MRIs to be discovered in each iteration. The discovered MRIs are then used to update a probability vector. We design two optimization strategies to improve the algorithm's performance. The adaptive sample size strategy narrows the search space as the number of iterations increases, and the crossover-based individual generation strategy improves the Image 9. To evaluate the performance of MRI-CE, we select six competitive algorithms and conduct extensive experiments on publicly available datasets. The results show that the proposed algorithm is not only efficient, but also highly accurate. Furthermore, we verify the effectiveness of the two optimization strategies experimentally.
更多查看译文
关键词
minimal rare itemset,cross-entropy,progressive checking,adaptive sample size,crossover-based individual generation
AI 理解论文
溯源树
样例
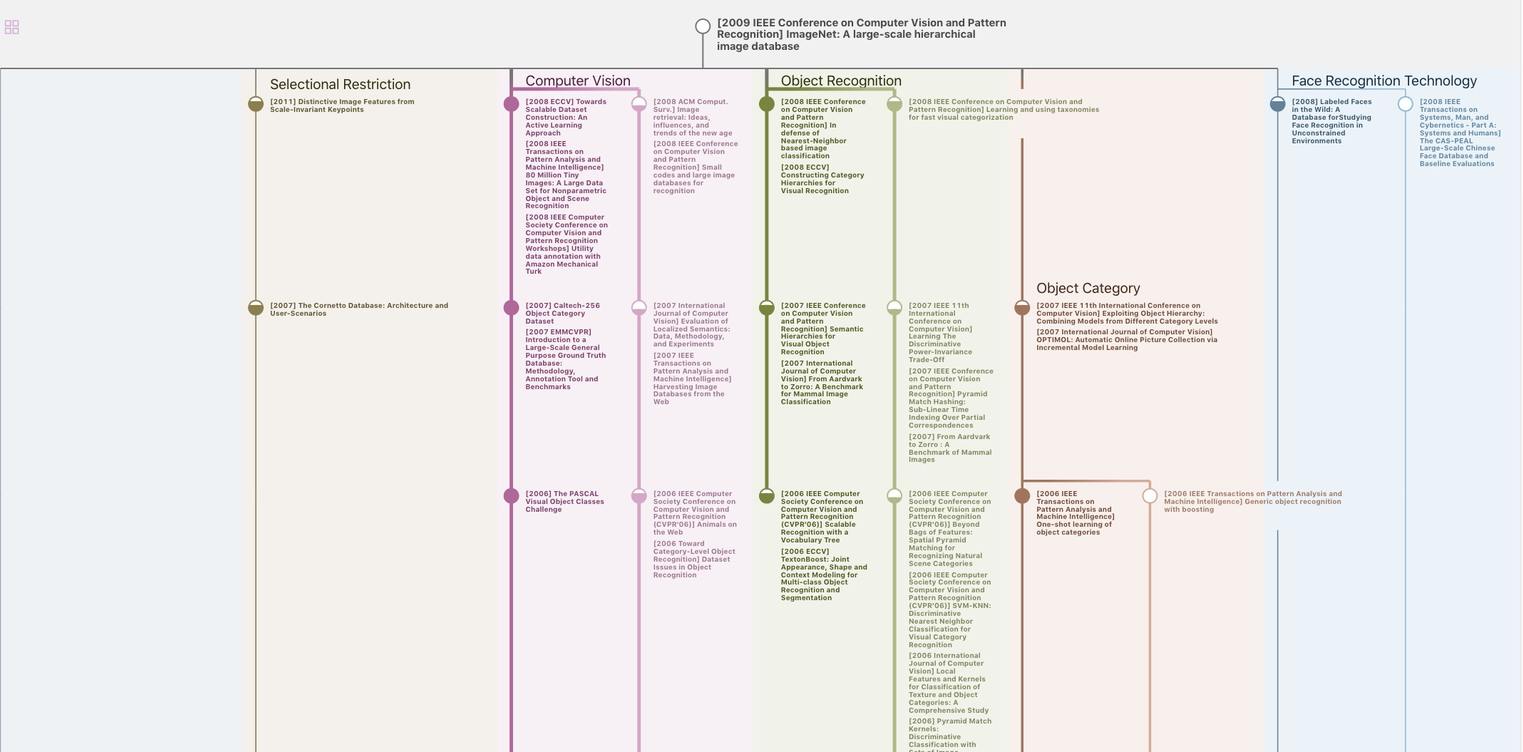
生成溯源树,研究论文发展脉络
Chat Paper
正在生成论文摘要