Improving adversarial transferability through frequency enhanced momentum
Information Sciences(2024)
摘要
The emergence of adversarial examples seriously affects the practical security deployment of convolutional neural networks. The existing attack algorithms perform brilliantly under white-box scenarios, but they show weak transferability when faced with unknown black-box models. Recent studies have revealed that models have different interests in different frequency components of images, and the low-frequency characteristics play a non-negligible role in the decision-making of models. In this article, we present the frequency enhanced momentum iterative attack, called FE-MI-FGSM. Specifically, we use multiple convolution kernels for Gaussian filtering of the image before each gradient update to push the processed image closer to the common decision boundaries of multiple models. Then, the average gradient of the white-box model to these processed images is obtained and used as the perturbation direction to generate adversarial examples with a high success rate of white-box attack and high transferability. The empirical results show that compared with the current mainstream gradient-based methods, our method performs better on both normally trained and adversarially trained models. Besides, our method can combine with gradient-based methods which integrate convergence algorithms or input transformations for the sake of reaching satisfactory improvement of the transferability.
更多查看译文
关键词
Adversarial example,Gradient-based attack,Adversarial transferability,Convolutional neural network,Frequency domain
AI 理解论文
溯源树
样例
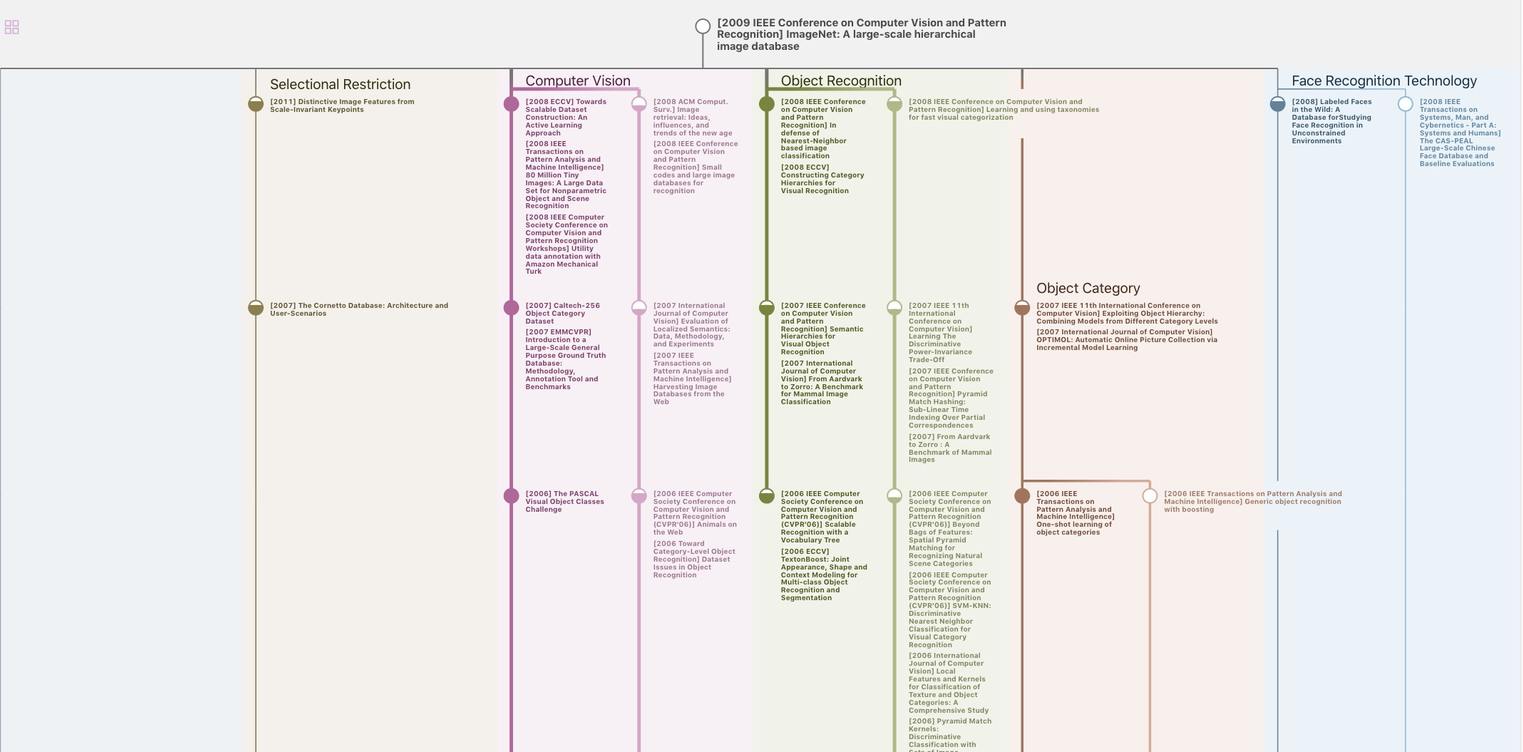
生成溯源树,研究论文发展脉络
Chat Paper
正在生成论文摘要