Evaluation of Deep Learning CTV auto-contouring for MRI-guided online adaptive treatment of rectal cancer
Advances in Radiation Oncology(2024)
摘要
Introduction
Segmentation of clinical target volumes (CTV) on medical images can be time-consuming and is prone to inter-observer variation (IOV). This is a problem for online adaptive radiotherapy, where CTV segmentation must be performed every treatment fraction, leading to longer treatment times and logistic challenges. Deep learning (DL)-based auto-contouring has the potential to speed up CTV contouring. But its current clinical use is limited. One reason for this is that it can be time-consuming to verify the accuracy of CTV contours produced using auto-contouring, and there is a risk of bias being introduced. To be accepted by clinicians, auto-contouring must be trustworthy. Therefore, there is a need for a comprehensive commissioning framework when introducing DL-based auto-contouring in clinical practice. We present such a framework and apply it to an in-house developed DL model for auto-contouring of the CTV in rectal cancer patients treated with MR-guided online adaptive radiotherapy.
Material and methods
The proposed framework for evaluating DL-based auto-contouring consists of three steps: 1. Quantitative evaluation of the model's performance and comparison with IOV2. Expert observations and corrections3. Evaluation of the impact on expected volumetric target coverage.These steps are performed on independent datasets. The framework is applied to an in-house trained nnU-Net model, using the data of 44 rectal cancer patients treated at our institution.
Results
The framework established that the model's performance after expert corrections was comparable to IOV, and while the model introduced a bias, this had no relevant impact on clinical practice. Additionally, we found a substantial time gain without reducing quality as determined by volumetric target coverage.
Discussion
Our framework provides a comprehensive evaluation of the performance and clinical usability of target auto-contouring models. Based on the results, we conclude that the model is eligible for clinical use.
更多查看译文
AI 理解论文
溯源树
样例
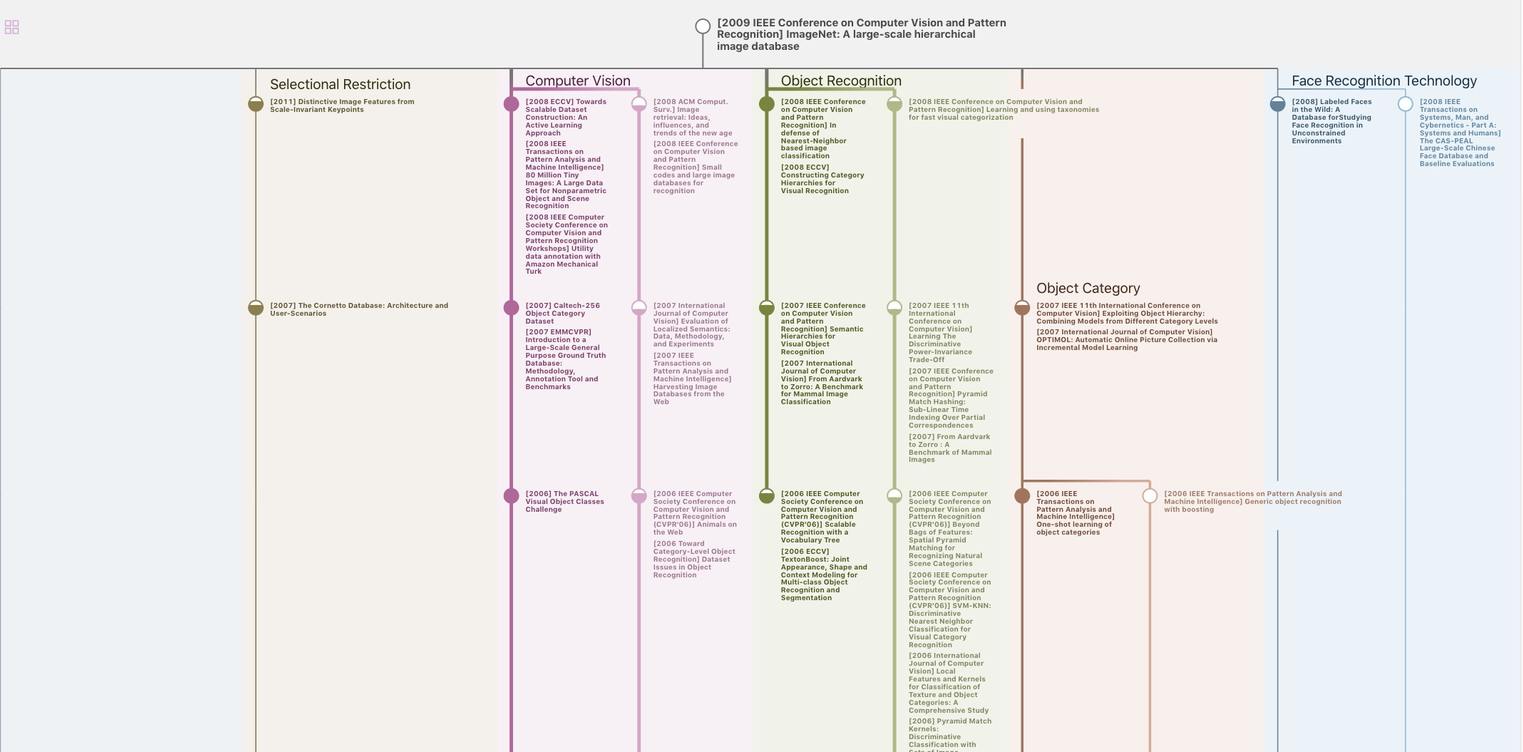
生成溯源树,研究论文发展脉络
Chat Paper
正在生成论文摘要