A Safe Screening Rule with Bi-level Optimization of ν Support Vector Machine
arxiv(2024)
摘要
Support vector machine (SVM) has achieved many successes in machine learning,
especially for a small sample problem. As a famous extension of the traditional
SVM, the ν support vector machine (ν-SVM) has shown outstanding
performance due to its great model interpretability. However, it still faces
challenges in training overhead for large-scale problems. To address this
issue, we propose a safe screening rule with bi-level optimization for
ν-SVM (SRBO-ν-SVM) which can screen out inactive samples before
training and reduce the computational cost without sacrificing the prediction
accuracy. Our SRBO-ν-SVM is strictly deduced by integrating the
Karush-Kuhn-Tucker (KKT) conditions, the variational inequalities of convex
problems and the ν-property. Furthermore, we develop an efficient dual
coordinate descent method (DCDM) to further improve computational speed.
Finally, a unified framework for SRBO is proposed to accelerate many SVM-type
models, and it is successfully applied to one-class SVM. Experimental results
on 6 artificial data sets and 30 benchmark data sets have verified the
effectiveness and safety of our proposed methods in supervised and unsupervised
tasks.
更多查看译文
AI 理解论文
溯源树
样例
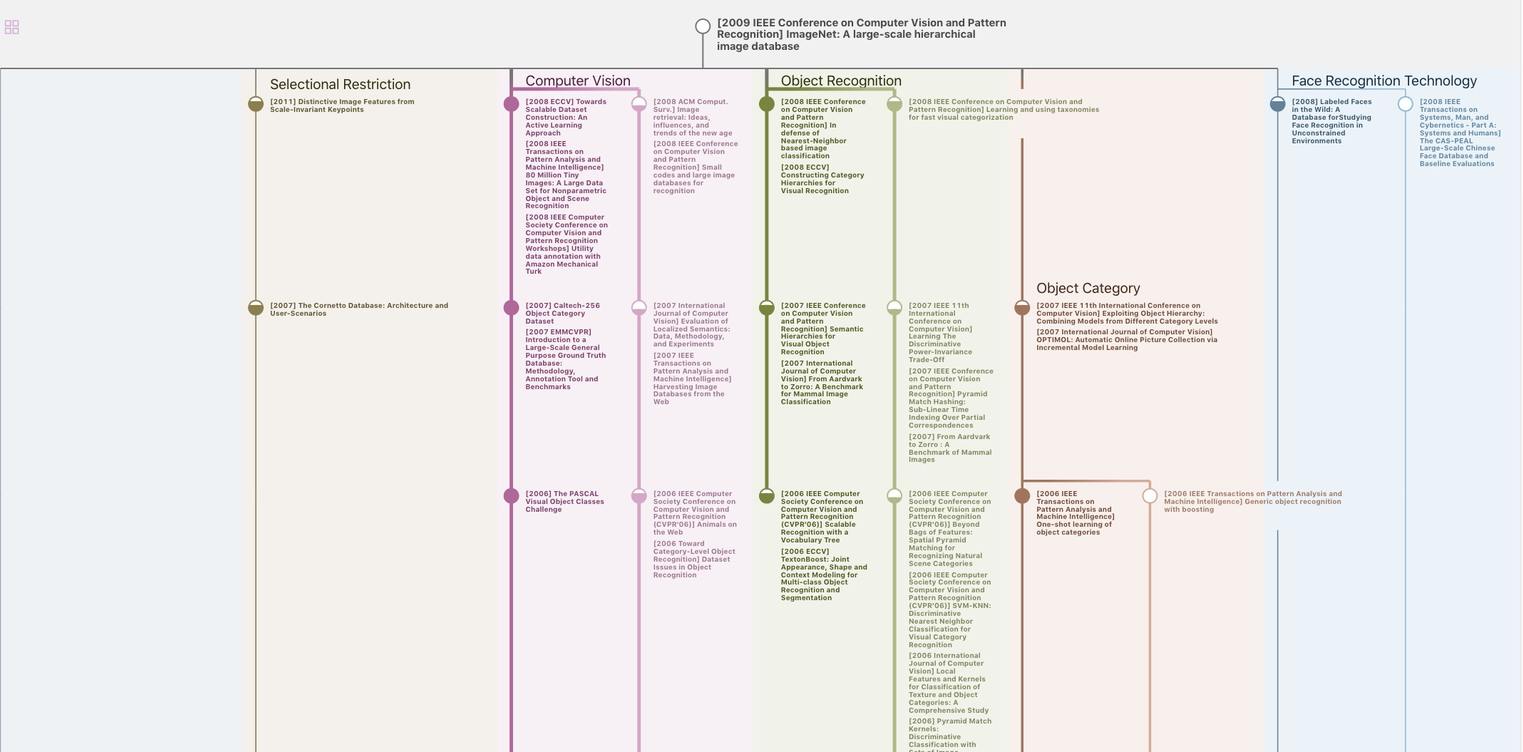
生成溯源树,研究论文发展脉络
Chat Paper
正在生成论文摘要