HINTs: Sensemaking on large collections of documents with Hypergraph visualization and INTelligent agents
arxiv(2024)
摘要
Sensemaking on a large collection of documents (corpus) is a challenging task
often found in fields such as market research, legal studies, intelligence
analysis, political science, computational linguistics, etc. Previous works
approach this problem either from a topic- or entity-based perspective, but
they lack interpretability and trust due to poor model alignment. In this
paper, we present HINTs, a visual analytics approach that combines topic- and
entity-based techniques seamlessly and integrates Large Language Models (LLMs)
as both a general NLP task solver and an intelligent agent. By leveraging the
extraction capability of LLMs in the data preparation stage, we model the
corpus as a hypergraph that matches the user's mental model when making sense
of the corpus. The constructed hypergraph is hierarchically organized with an
agglomerative clustering algorithm by combining semantic and connectivity
similarity. The system further integrates an LLM-based intelligent chatbot
agent in the interface to facilitate sensemaking. To demonstrate the
generalizability and effectiveness of the HINTs system, we present two case
studies on different domains and a comparative user study. We report our
insights on the behavior patterns and challenges when intelligent agents are
used to facilitate sensemaking. We find that while intelligent agents can
address many challenges in sensemaking, the visual hints that visualizations
provide are necessary to address the new problems brought by intelligent
agents. We discuss limitations and future work for combining interactive
visualization and LLMs more profoundly to better support corpus analysis.
更多查看译文
AI 理解论文
溯源树
样例
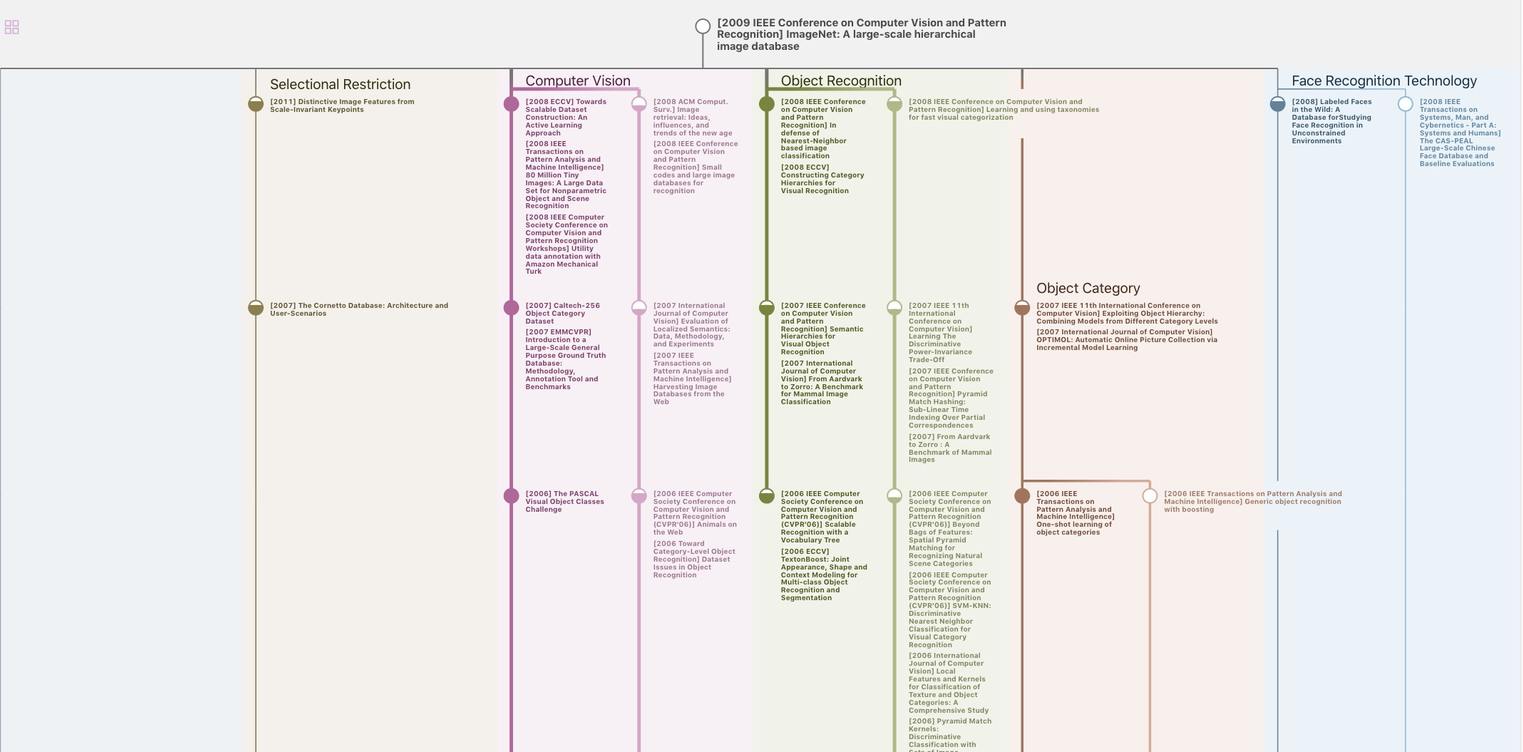
生成溯源树,研究论文发展脉络
Chat Paper
正在生成论文摘要