Structured methods for parameter inference and uncertainty quantification for mechanistic models in the life sciences
arxiv(2024)
摘要
Parameter inference and uncertainty quantification are important steps when
relating mathematical models to real-world observations, and when estimating
uncertainty in model predictions. However, methods for doing this can be
computationally expensive, particularly when the number of unknown model
parameters is large. The aim of this study is to develop and test an efficient
profile likelihood-based method, which takes advantage of the structure of the
mathematical model being used. We do this by identifying specific parameters
that affect model output in a known way, such as a linear scaling. We
illustrate the method by applying it to three caricature models from different
areas of the life sciences: (i) a predator-prey model from ecology; (ii) a
compartment-based epidemic model from health sciences; and, (iii) an
advection-diffusion-reaction model describing transport of dissolved solutes
from environmental science. We show that the new method produces results of
comparable accuracy to existing profile likelihood methods, but with
substantially fewer evaluations of the forward model. We conclude that our
method could provide a much more efficient approach to parameter inference for
models where a structure approach is feasible. Code to apply the new method to
user-supplied models and data is provided via a publicly accessible repository.
更多查看译文
AI 理解论文
溯源树
样例
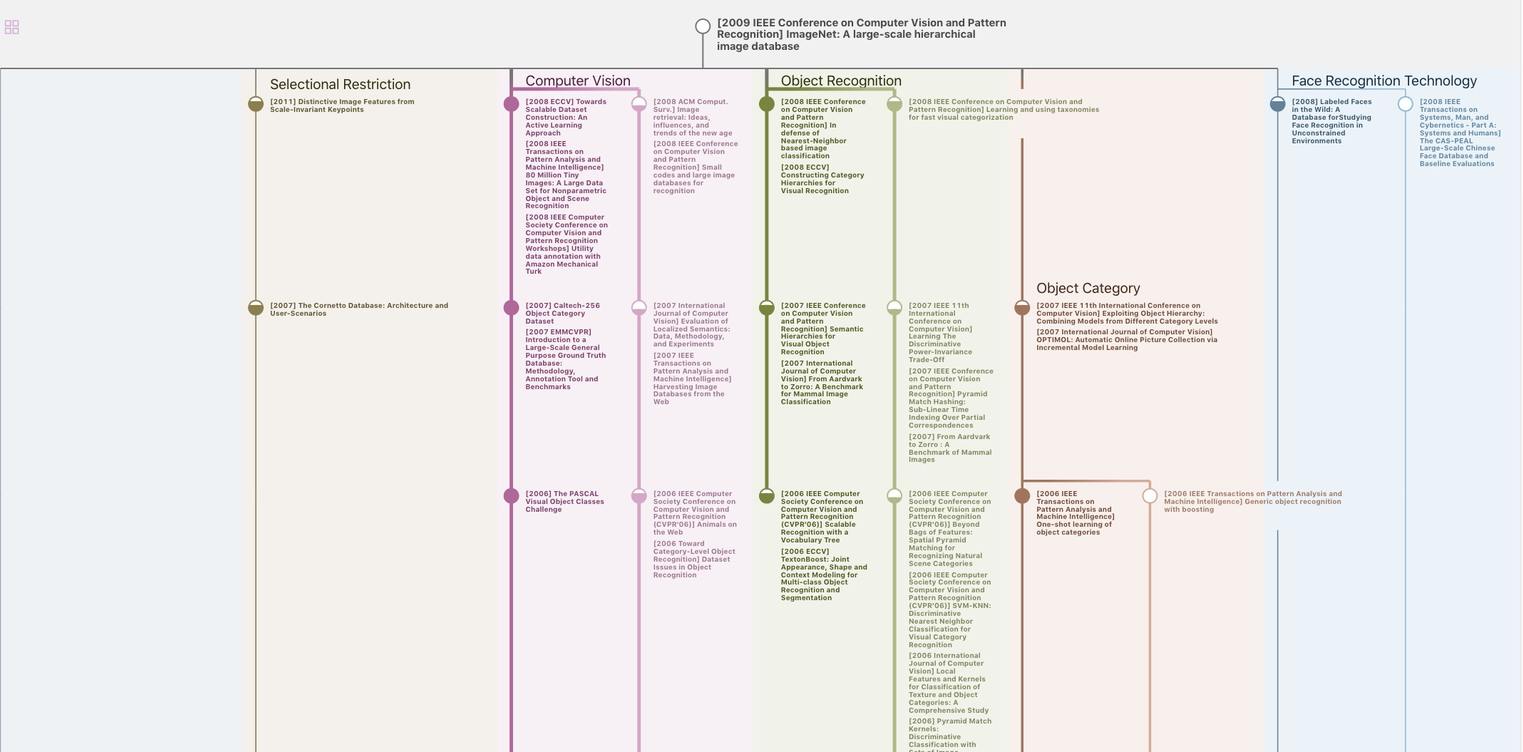
生成溯源树,研究论文发展脉络
Chat Paper
正在生成论文摘要