Statistical Query Lower Bounds for Learning Truncated Gaussians
arxiv(2024)
摘要
We study the problem of estimating the mean of an identity covariance
Gaussian in the truncated setting, in the regime when the truncation set comes
from a low-complexity family 𝒞 of sets. Specifically, for a fixed
but unknown truncation set S ⊆ℝ^d, we are given access to
samples from the distribution 𝒩(μ, 𝐈)
truncated to the set S. The goal is to estimate μ within
accuracy ϵ>0 in ℓ_2-norm. Our main result is a Statistical Query
(SQ) lower bound suggesting a super-polynomial information-computation gap for
this task. In more detail, we show that the complexity of any SQ algorithm for
this problem is d^poly(1/ϵ), even when the class
𝒞 is simple so that poly(d/ϵ) samples
information-theoretically suffice. Concretely, our SQ lower bound applies when
𝒞 is a union of a bounded number of rectangles whose VC dimension
and Gaussian surface are small. As a corollary of our construction, it also
follows that the complexity of the previously known algorithm for this task is
qualitatively best possible.
更多查看译文
AI 理解论文
溯源树
样例
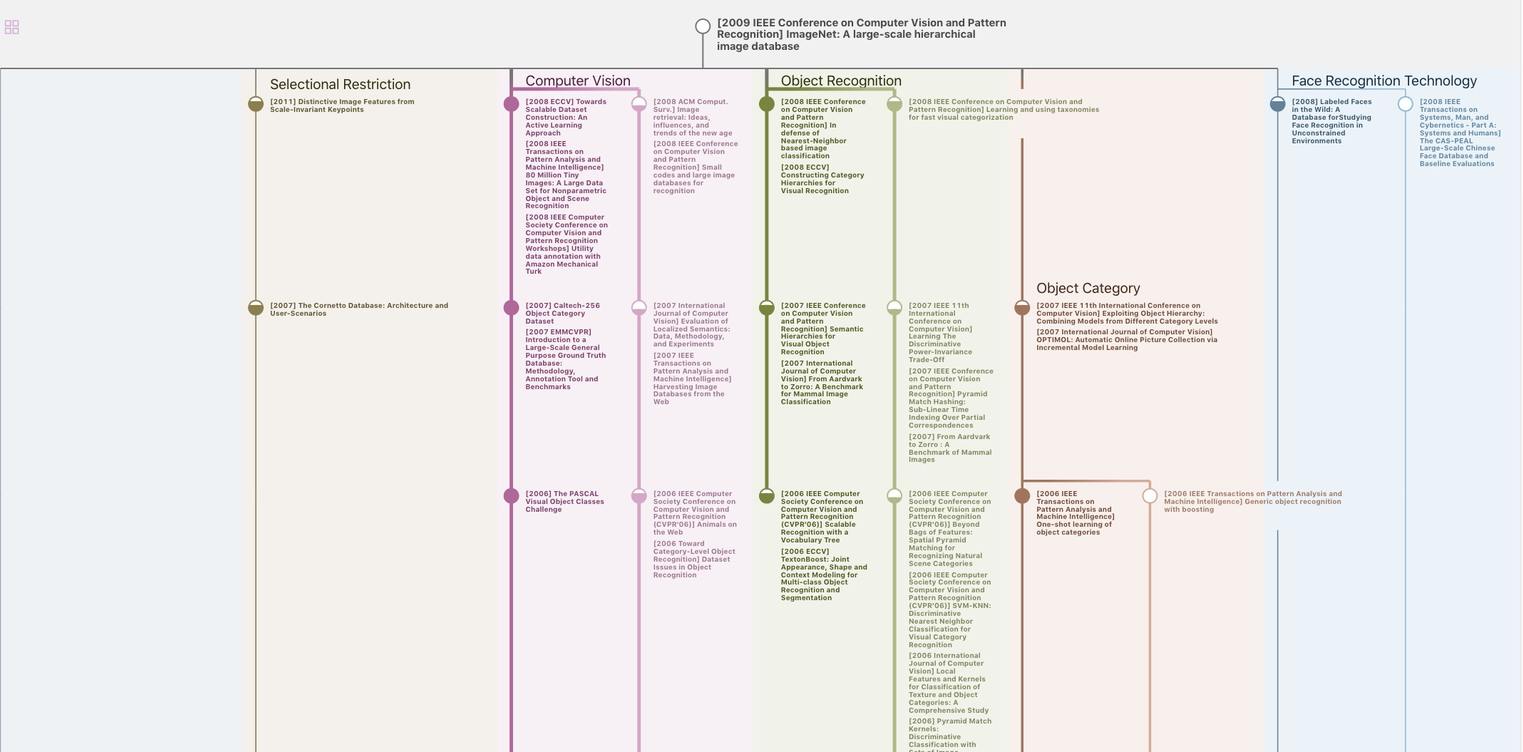
生成溯源树,研究论文发展脉络
Chat Paper
正在生成论文摘要