Fragment-Based Deep Learning for Simultaneous Prediction of Polarizabilities and NMR Shieldings of Macromolecules and Their Aggregates
JOURNAL OF CHEMICAL THEORY AND COMPUTATION(2024)
摘要
Simultaneous prediction of the molecular response properties, such as polarizability and the NMR shielding constant, at a low computational cost is an unresolved issue. We propose to combine a linear-scaling generalized energy-based fragmentation (GEBF) method and deep learning (DL) with both molecular and atomic information-theoretic approach (ITA) quantities as effective descriptors. In GEBF, the total molecular polarizability can be assembled as a linear combination of the corresponding quantities calculated from a set of small embedded subsystems in GEBF. In the new GEBF-DL(ITA) protocol, one can predict subsystem polarizabilities based on the corresponding molecular wave function (thus electron density and ITA quantities) and DL model rather than calculate them from the computationally intensive coupled-perturbed Hartree-Fock or Kohn-Sham equations and finally obtain the total molecular polarizability via a linear combination equation. As a proof-of-concept application, we predict the molecular polarizabilities of large proteins and protein aggregates. GEBF-DL(ITA) is shown to be as accurate enough as GEBF, with mean absolute percentage error <1%. For the largest protein aggregate (>4000 atoms), GEBF-DL(ITA) gains a speedup ratio of 3 compared with GEBF. It is anticipated that when more advanced electronic structure methods are used, this advantage will be more appealing. Moreover, one can also predict the NMR chemical shieldings of proteins with reasonably good accuracy. Overall, the cost-efficient GEBF-DL(ITA) protocol should be a robust theoretical tool for simultaneously predicting polarizabilities and NMR shieldings of large systems.
更多查看译文
AI 理解论文
溯源树
样例
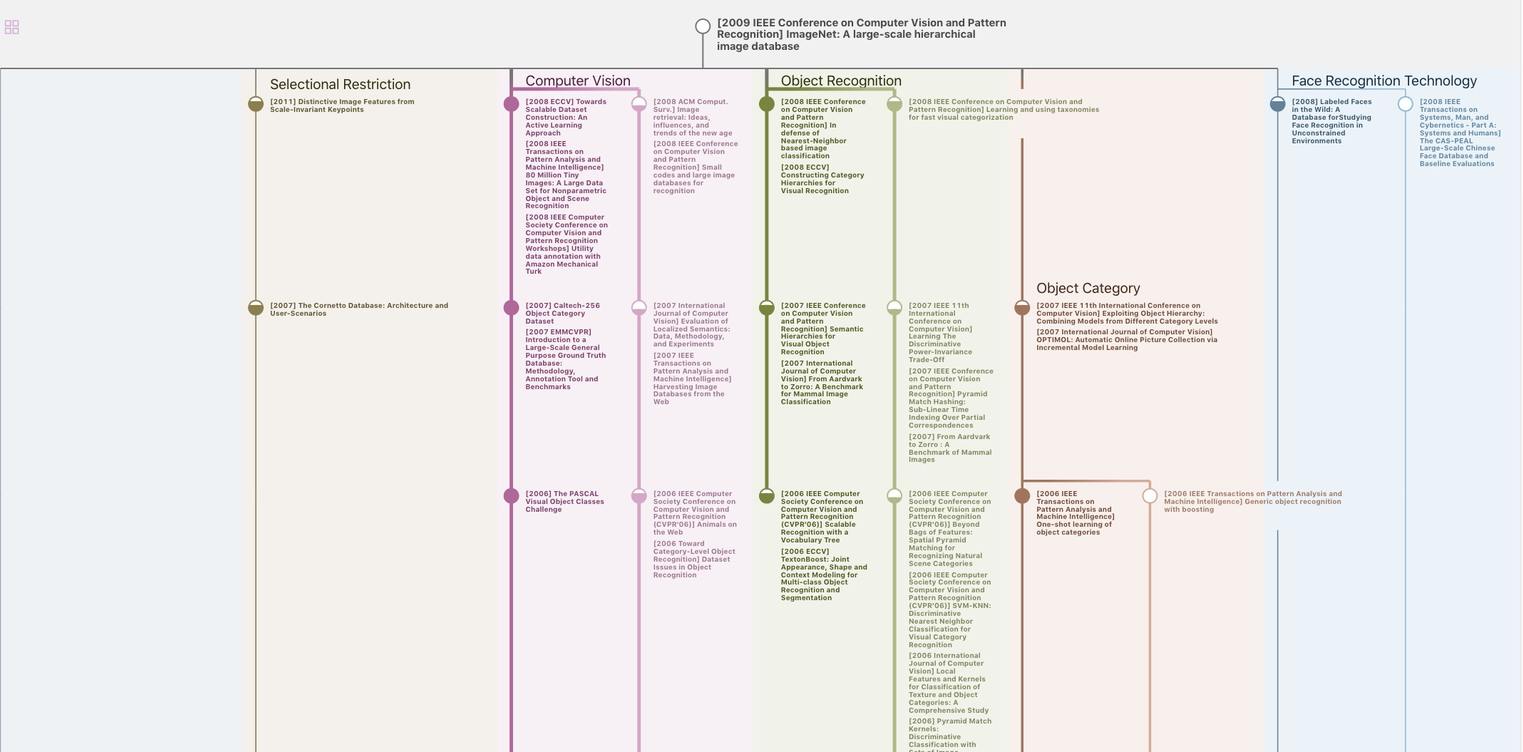
生成溯源树,研究论文发展脉络
Chat Paper
正在生成论文摘要