Improving the perception of low-light enhanced images
OPTICS EXPRESS(2024)
摘要
Improving images captured under low -light conditions has become an important topic in computational color imaging, as it has a wide range of applications. Most current methods are either based on handcrafted features or on end -to -end training of deep neural networks that mostly focus on minimizing some distortion metric -such as PSNR or SSIM- on a set of training images. However, the minimization of distortion metrics does not mean that the results are optimal in terms of perception (i.e. perceptual quality). As an example, the perception -distortion trade-off states that, close to the optimal results, improving distortion results in worsening perception. This means that current low -light image enhancement methods -that focus on distortion minimization- cannot be optimal in the sense of obtaining a good image in terms of perception errors. In this paper, we propose a post -processing approach in which, given the original low -light image and the result of a specific method, we are able to obtain a result that resembles as much as possible that of the original method, but, at the same time, giving an improvement in the perception of the final image. More in detail, our method follows the hypothesis that in order to minimally modify the perception of an input image, any modification should be a combination of a local change in the shading across a scene and a global change in illumination color. We demonstrate the ability of our method quantitatively using perceptual blind image metrics such as BRISQUE, NIQE, or UNIQUE, and through user preference tests. Published by Optica Publishing Group under the terms of the Creative Commons Attribution 4.0 License. Further distribution of this work must maintain attribution to the author(s) and the published article's title, journal citation, and DOI.
更多查看译文
AI 理解论文
溯源树
样例
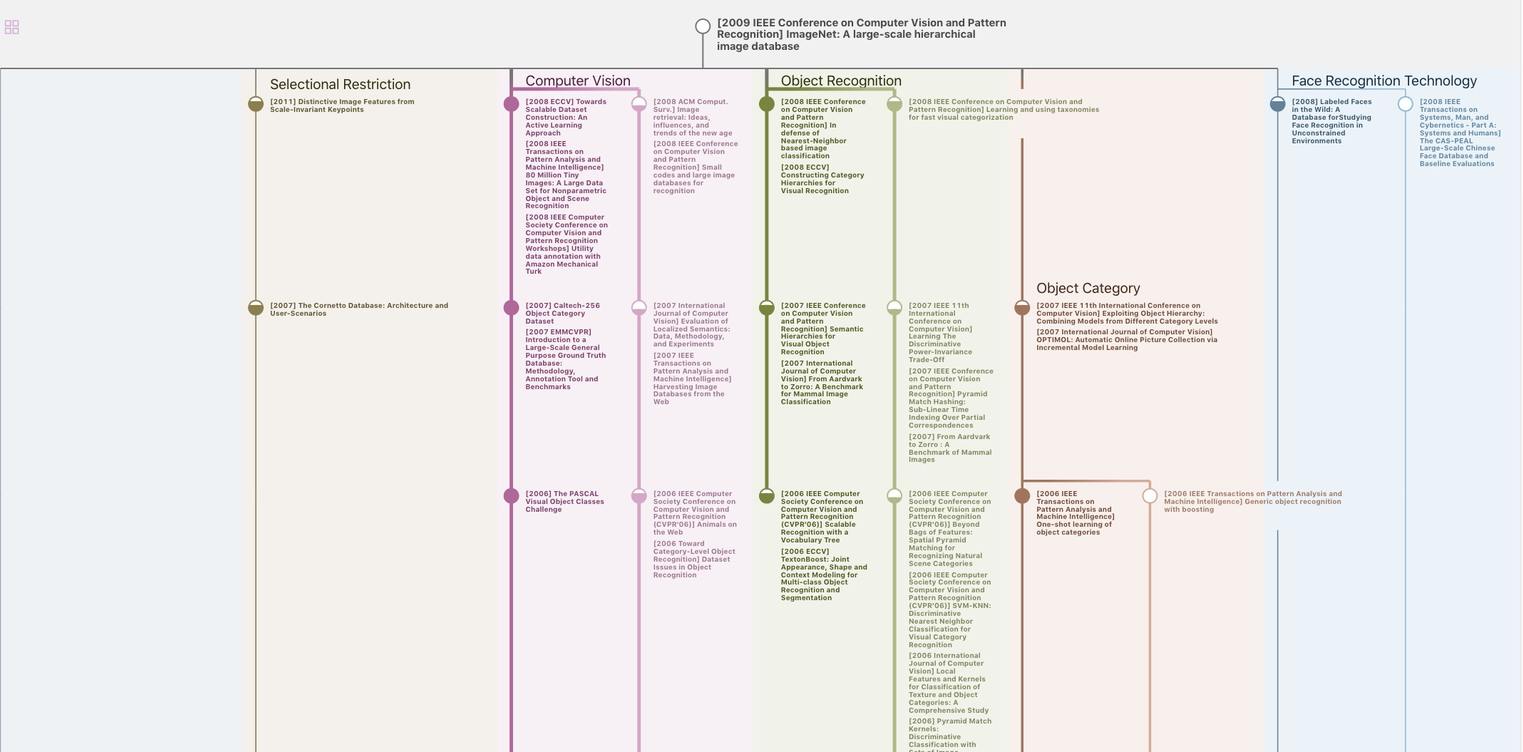
生成溯源树,研究论文发展脉络
Chat Paper
正在生成论文摘要