Corr-Track: Category-Level 6D Pose Tracking with Soft-Correspondence Matrix Estimation.
IEEE transactions on visualization and computer graphics(2024)
摘要
Category-level pose tracking methods can continuously track the pose of objects without requiring any prior knowledge of the specific shape of the tracked instance. This makes them advantageous in augmented reality and virtual reality applications. The key challenge is how to train neural networks to accurately predict the poses of objects they have never seen before and exhibit strong generalization performance. We propose a novel category-level 6D pose tracking method Corr-Track, which is capable of accurately tracking objects belonging to the same category from depth video streams. Our approach utilizes direct soft correspondence constraints to train a neural network, which estimates bidirectional soft correspondences between sparsely sampled point clouds of objects in two frames. We first introduce a soft correspondence matrix for pose tracking tasks and establish effective constraints through direct spatial point-to-point correspondence representations in the sparse point cloud correspondence matrix. We propose the "point cloud expansion" strategy to address the "point cloud shrinkage" problem resulting from soft correspondences. This strategy ensures that the corresponding point cloud accurately reproduces the shape of the target point cloud, leading to precise pose tracking results. We evaluated our approach on the NOCS-REAL275 and Wild6D dataset and observed superior performance compared to previous methods. Additionally, we conducted cross-category experiments that further demonstrated its generalization capability.
更多查看译文
关键词
Category-level object pose estimation,object tracking
AI 理解论文
溯源树
样例
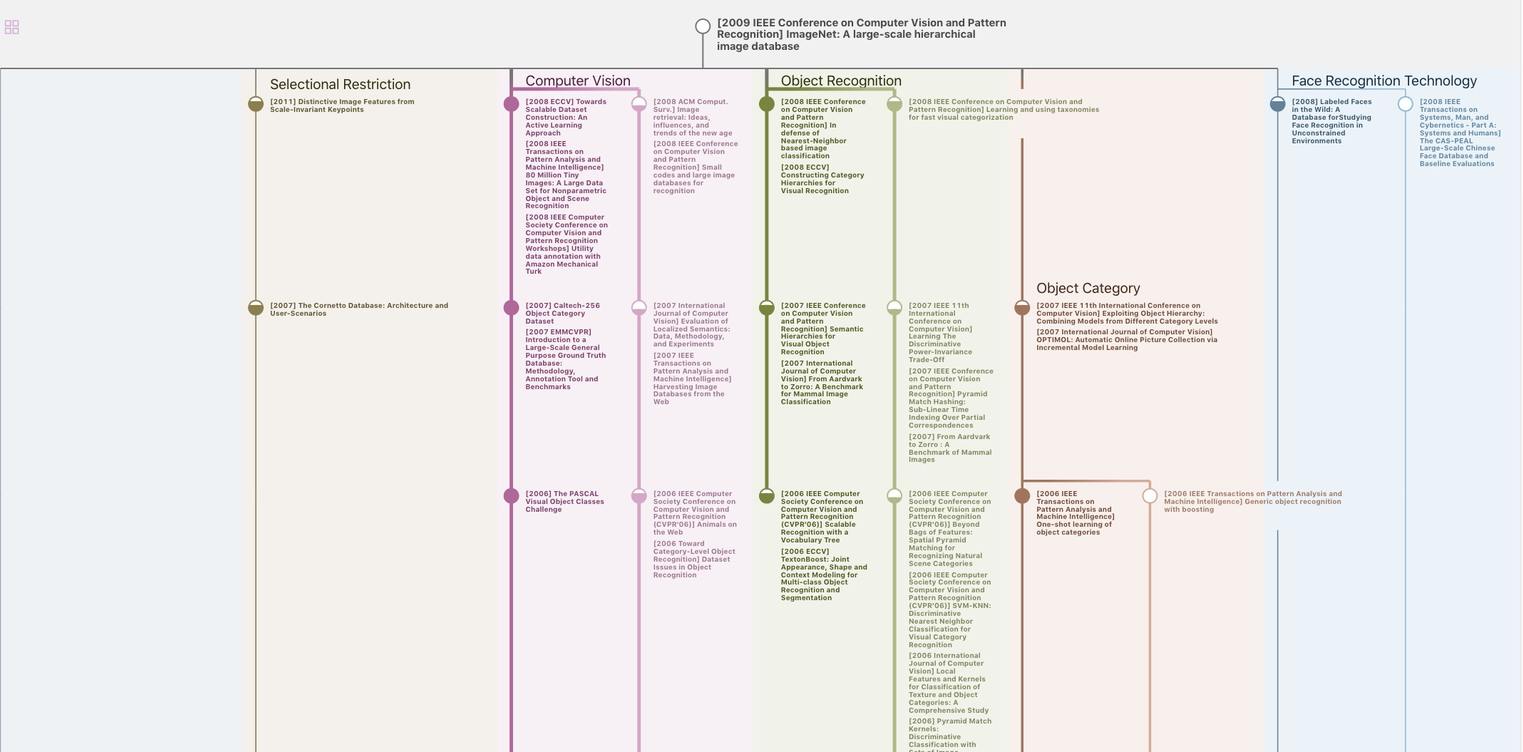
生成溯源树,研究论文发展脉络
Chat Paper
正在生成论文摘要