HIL Testbed-based Auto Feature Extraction and Data Generation Framework for ML/DL-based Anomaly Detection and Classification.
2024 IEEE Power & Energy Society Innovative Smart Grid Technologies Conference (ISGT)(2024)
摘要
In smart grid operations, sufficient data generation, including events such as faults, cyberattacks, and perturbations, is one of the key requirements for accurate Machine Learning (ML) and Deep Learning (DL) based anomaly detection and classification. However, the public datasets in power systems pose data sufficiency, bias, and uncertainty challenges. Moreover, synthetic datasets may only capture some feature vectors in power systems and complex dynamics, resulting in sub-optimal performance of ML/DL models. In this paper, we proposed the Auto Feature Extraction and Data Generation (AFEDG) framework for ML and DL models in smart grid applications such as anomaly detection and classification. We developed a Python-based Application Programming Interface (API) to automate the extraction of feature vectors and data generation processes by integrating virtual sensors. The API dynamically modulates parameters and signals within the power system model, ensuring comprehensive coverage of all event scenarios and generating unbiased, balanced datasets. The proposed API is designed to seamlessly integrate with power systems, exhibiting scalability and adaptability regardless of the number of buses within the system. We implemented the proposed AFEDG framework on a Hardware-in-the-Loop (HIL) Cyber-Physical System (CPS) testbed to generate large-scale real-time datasets. In our case study, we used the Python API to generate electric fault datasets on the modified SSN-distribution bus grid model in OPAL-RT, integrating with RT-LAB and MATLAB.
更多查看译文
关键词
feature vectors extraction,Python API,real-time data,RT-LAB,Smart Grid
AI 理解论文
溯源树
样例
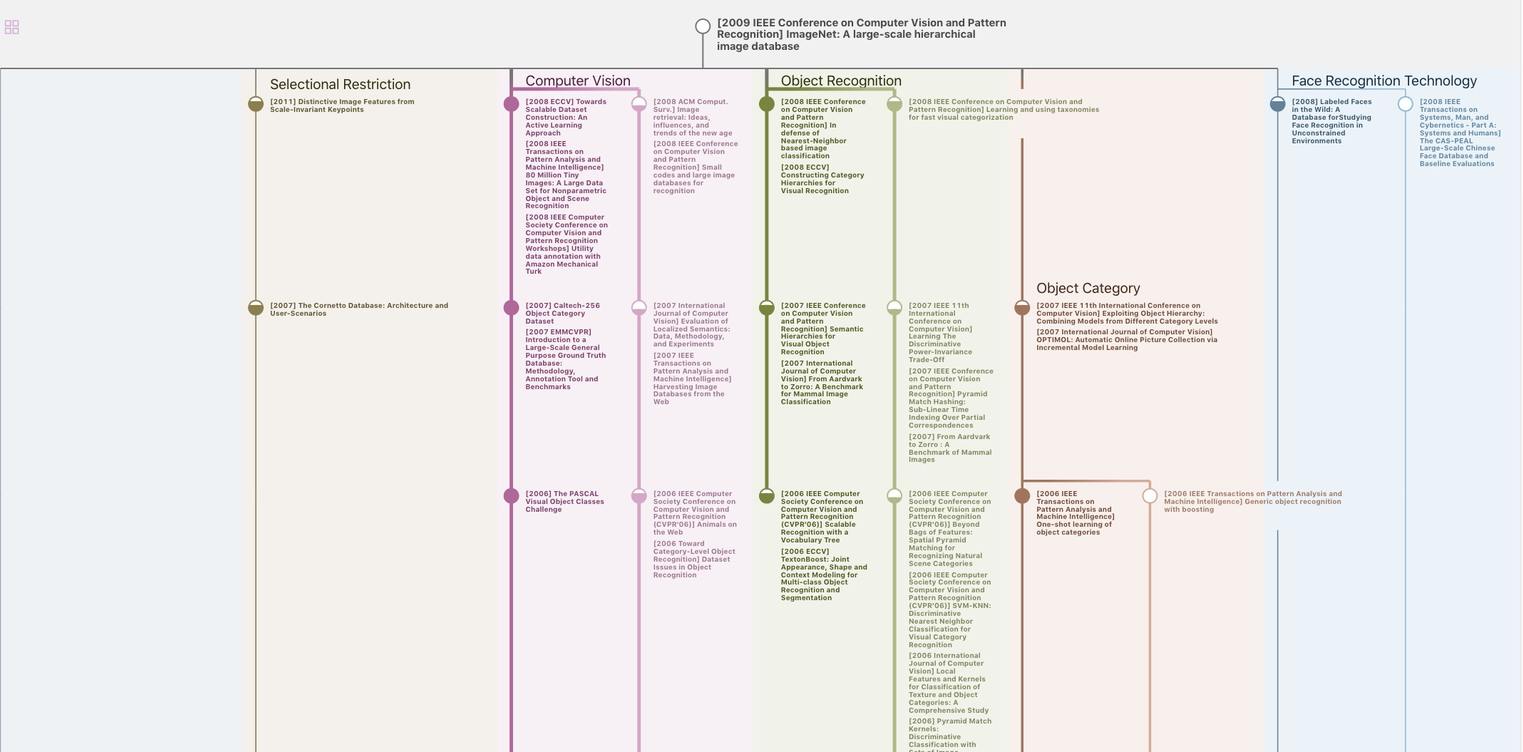
生成溯源树,研究论文发展脉络
Chat Paper
正在生成论文摘要