Optimal Prescribed Performance Control for Euler-Lagrange Systems.
International Conference on Systems and Control(2023)
摘要
This work introduces an optimal control policy aiming to stabilize an Euler-Lagrange system of unknown dynamics, while simultaneously satisfying predefined response criteria. The proposed methodology unfolds in two stages. In the initial phase, a neural network is trained online via an iterative process to capture the system's dynamics. Subsequently, in the second phase, by utilizing the acquired dynamics, a successive approximation algorithm is employed to derive a nearly optimal control law while taking into consideration prescribed performance specifications such as convergence speed and steady state error. Validation of our method is achieved through a simulated paradigm, confirming the success of both the identification process and the minimization of the adopted integral cost.
更多查看译文
关键词
Prescribed Performance Control,Euler-Lagrange Systems,Neural Network,Steady State,System Dynamics,Optimal Control,Convergence Rate,Optimal Policy,Steady-state Error,Unknown Dynamics,Optimal Control Policy,Optimal Control Law,Degrees Of Freedom,Optimization Problem,Cost Function,Control Problem,Transient State,Dynamic Programming,Function Approximation,Tracking Error,Optimal Control Problem,Reference Trajectory,Absence Of Knowledge,Admission Policies,Shallow Neural Network,Knowledge Of Dynamics,Steady-state Response,Gravity Vector,Regulation Problem,Transient Performance
AI 理解论文
溯源树
样例
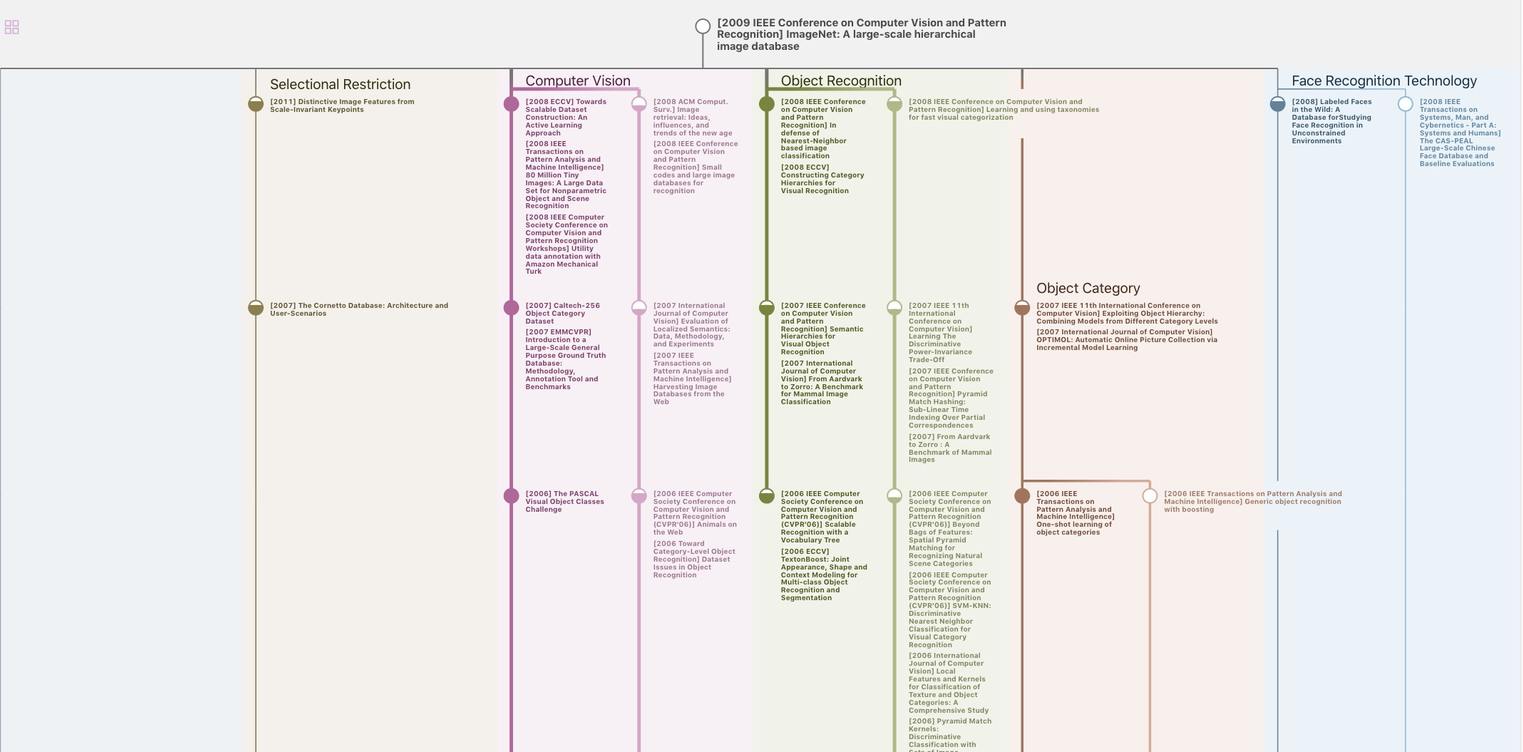
生成溯源树,研究论文发展脉络
Chat Paper
正在生成论文摘要