Development of a Prototype Clinical Decision Support Tool for a Machine Learning Based Individualized Prediction of Outcomes of Hematopoietic Cell Transplantation for Sickle Cell Disease
Transplantation and Cellular Therapy(2024)
摘要
Introduction
Hematopoietic cell transplantation (HCT) remains the only curative therapy for sickle cell disease (SCD), but the lack of a prediction model that addresses the uncertainty regarding individual outcomes and adverse events is a barrier for shared decision making. Computational machine-learning (ML) has the potential to determine generalizable predictive patterns and quantifying uncertainty in those estimates allowing clinicians to assess the confidence level of the predictions and avoid over-reliance on potentially incorrect results, allow for risk management and mitigation by considering the potential range of outcomes, permit better decision-making
Methods
We applied a supervised random forest ML model to prognostically relevant clinical parameters and both categorical and time-to-event outcomes in a de-identified CIBMTR dataset of patients undergoing HCT and reported to CIBMTR 1990-2021. To counter the imbalance bias towards predicting positive outcomes due to the small number of negative outcomes we constructed a training dataset taking each outcome variable of interest, and included randomly sampled positive outcomes, typically 1.5-3 times the total instances of the variable of interest. We ran the test dataset, and used a random forest on a two-times Repeated 10 Fold Cross Validation, to demonstrate our model's versatility and response to unknown data. We estimated balanced accuracy which is the arithmetic mean of sensitivity and specificity. We also measured Area under the Receiver Operator Characteristic Curve (AUC), a measure of the ability of a binary classifier to distinguish between classes.
Results
We examined de-identified records of 1641 patients who were followed for a median of 47.8 months (0.3-312.9). Patient age at HCT <18 years in 73.4%, Karnofsky-Lansky (KPS) score ≥ 90 in 74.7%, overall survival 91.2%, event-free survival 75.5%, graft failure (GF) 17.9%, AGVHD 18.3%, and CGVHD 22.3%. Overall, the predictive model provided acceptable AUC (0.68-0.88), Balanced accuracy,(0.61-0.77) positive predictive value ( 0.1-0.51), and sensitivity ( 0.41-0.69) . We then developed a prototype decision support tool using Shiny App package (Posit Inc. Boston, MA). The tool allows for user input of various parameters, the choice to implement published conditioning and GVHD prophylaxis regimens or create custom ones and provides the predicted probability of several outcomes. (Figure is a Screenshot of the decision support tool with an individualized prediction of outcomes for a hypothetical patient).
Conclusions
We report the development, testing, and validation of an ML model for individualized prediction of outcomes of HCT for SCD with acceptable AUC, accuracy, balanced accuracy, positive predictive value and sensitivity. The decision support tool has the potential to aid clinicians in making shared decisions with their patients regarding HCT for SCD.
更多查看译文
AI 理解论文
溯源树
样例
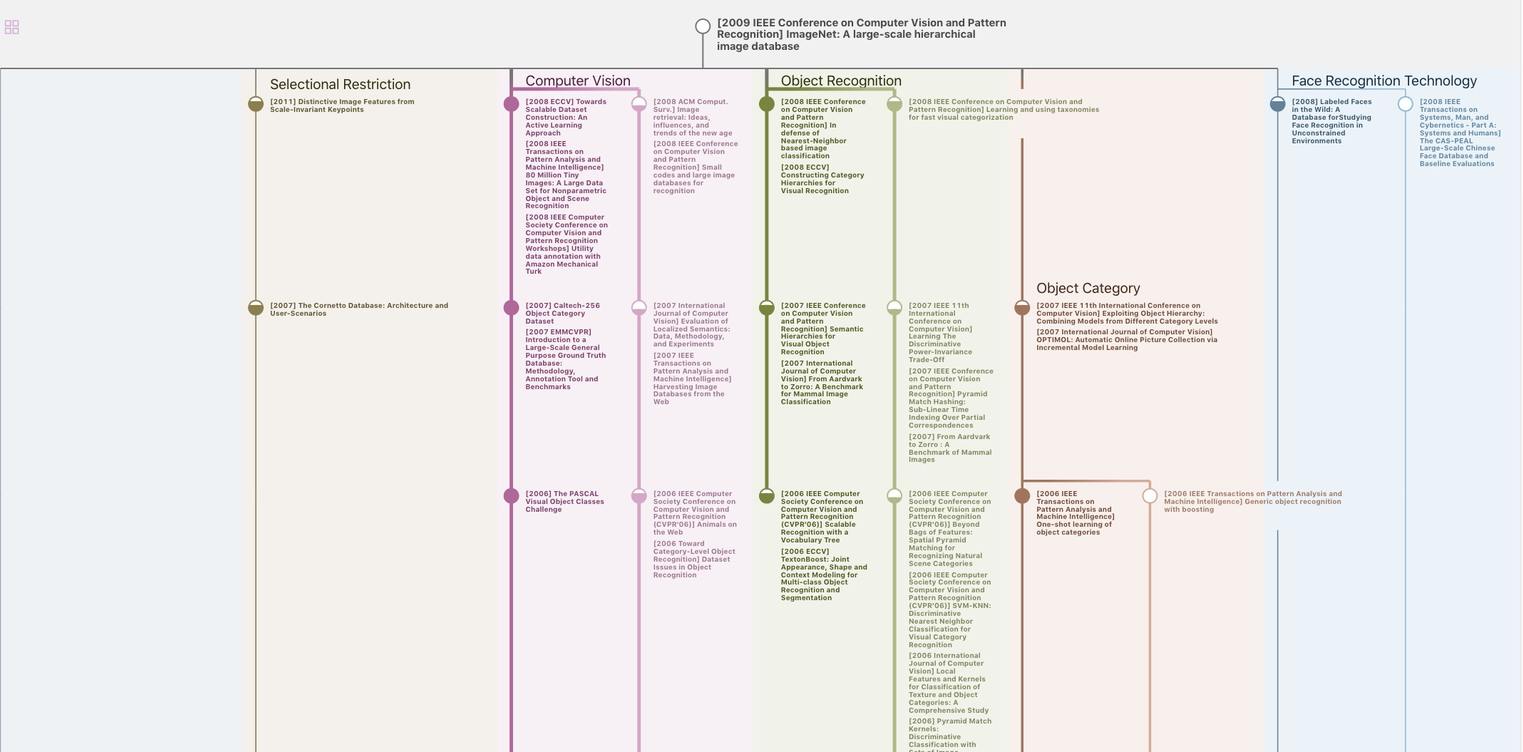
生成溯源树,研究论文发展脉络
Chat Paper
正在生成论文摘要