Democratizing traffic control in smart cities
TRANSPORTATION RESEARCH PART C-EMERGING TECHNOLOGIES(2024)
摘要
To improve the performance of systems, optimization has been the prevailing approach in the past. However, the approach faces challenges when multiple goals shall be simultaneously achieved. For illustration, we study a multi -agent system, where agents have a plurality of different, and mutually inconsistent goals. We then allow agents in the system to vote on which traffic signal controllers, which were trained on different goals using deep reinforcement learning, would control the intersection. Taking decisions based on suitable voting procedures turns out to lead to favorable solutions, which perform highly for several goals rather than optimally for one goal and poorly for others. This opens up new opportunities for the management or even self -governance of complex systems that require the consideration and achievement of multiple goals, such as many systems involving humans. Here, we present results for traffic flows in urban street networks, which suggest that "democratizing traffic"would be a promising alternative to centralized control of traffic flows.
更多查看译文
关键词
Traffic control,Deep reinforcement learning,Social participation,Voting,Smart cities
AI 理解论文
溯源树
样例
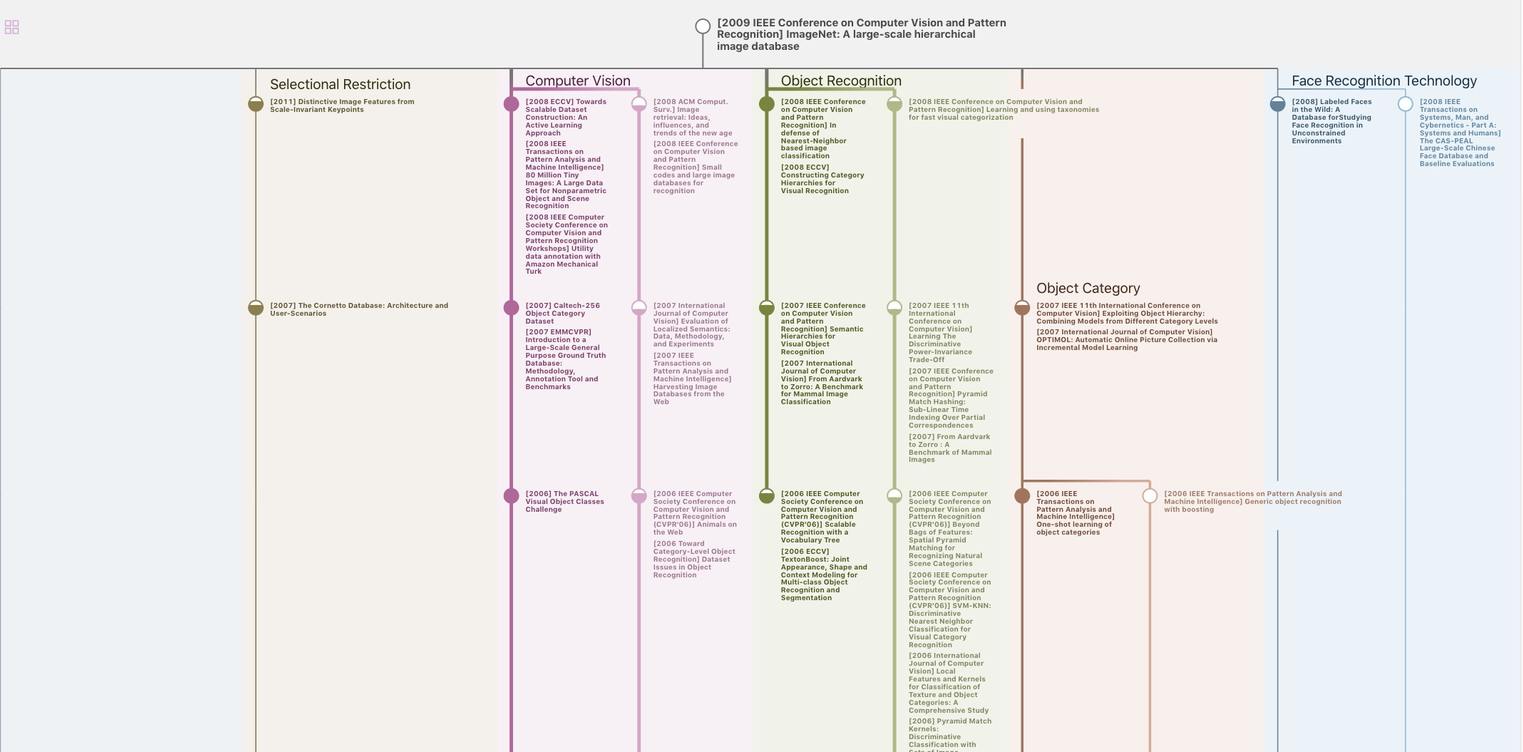
生成溯源树,研究论文发展脉络
Chat Paper
正在生成论文摘要