On the performance of pothole detection algorithms enhanced via data augmentation
Transportation Research Procedia(2024)
摘要
Road infrastructure is necessary for any country to function as daily life depends upon it. Transport authorities monitor road infrastructure to identify distressed areas and repair them, enhancing users’ comfort and safety. Major steps have been taken in automating road monitoring using sensing- and vision-based technologies, reducing the level of subjectivity caused by traditional manual inspection processes. However, automated detection of distressed areas is often challenged by an insufficient amount of training data or uneven class balance within datasets. Data augmentation techniques offer the opportunity to enlarge the size and quality of existing datasets. This paper investigates how various augmentation techniques impact the performance of a set of representative detection algorithms applied to detecting highway potholes. The results suggest that: firstly, no specific augmentation seems to be more beneficial than the others in this domain; and second, using a moderate amount of augmentation techniques can provide performance gains. This work forms the basis for performance improvement of multiple detection algorithms, used for transport infrastructure management, and it consequently contributes to enhanced transport systems simulation and monitoring.
更多查看译文
关键词
pavement defect classification,computer vision,machine learning,syntetic data
AI 理解论文
溯源树
样例
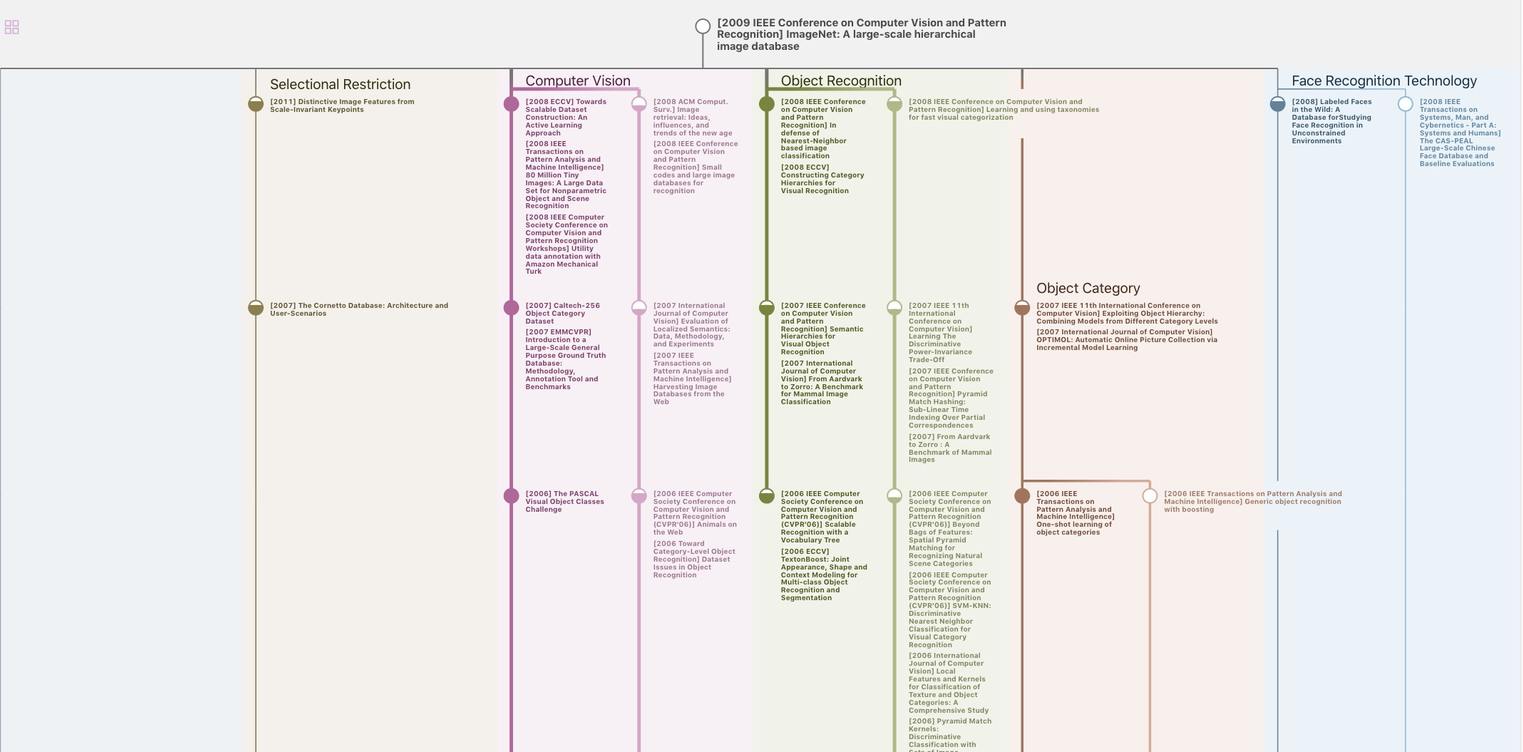
生成溯源树,研究论文发展脉络
Chat Paper
正在生成论文摘要