Intra-hour solar irradiance forecasting using topology data analysis and physics-driven deep learning
Renewable Energy(2024)
摘要
Real-time Direct Normal Irradiance (DNI) prediction is crucial for reliable and economic operation of Concentrated photothermal Solar Power (CSP) system in arid desert areas. However, the stochastic characteristics of short-term multidimensional meteorological time series make intra-hour DNI prediction a challenging task. In this study, we have proposed a deep learning model called TLD, which is combined with topological features captured by Topology Data Analysis (TDA) and temporal features captured by LSTM to address this challenge. Experimental results demonstrated that TLD outperformed the five latest models (Ridge, RF, C_GRU, BiLSTM, and GBRT) on seven solar radiation datasets in arid desert areas. Further analysis revealed that the proportion of cloudy days is a key factor affecting the model's performance. To enhance the forecast ability of TLD, we developed a physics-informed hybrid model named TLDP based on TLD and a smart persistence model, which fully combines the DNI prediction ability of TLD under cloudy conditions and that of the smart persistence model under sunny conditions. Experimental results of eight datasets collected from real-world solar photothermal power stations indicated that TLDP outperformed existing models, which may lay a foundation for more economical and stable operation of CSP plants in arid desert areas.
更多查看译文
关键词
Time series analysis,Topology data analysis,LSTM,DNI forecasting,TLDP
AI 理解论文
溯源树
样例
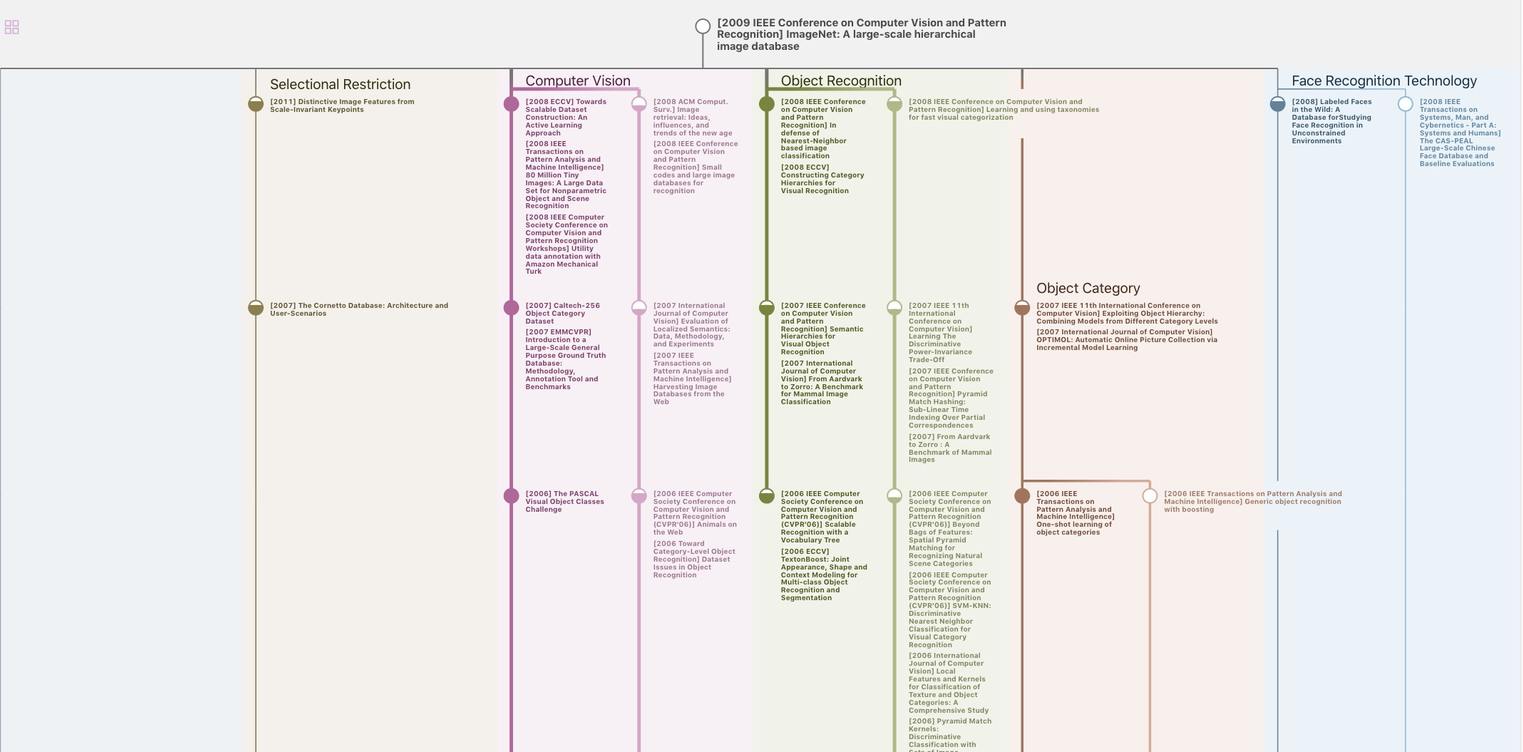
生成溯源树,研究论文发展脉络
Chat Paper
正在生成论文摘要