Hybrid MLP-CNN-based ground sink susceptibility prediction in urban area using underground pipe map
Reliability Engineering & System Safety(2024)
摘要
In urban areas, ground sinks are generally attributed to underground pipe damages resulting in leakage. Hence, it is essential to forecast ground sinks in advance because they might cause detrimental effects on infrastructure, including the roads, bridges, and railroads. Prior studies have focused on geological and topographical factors, with an inconsiderable amount of attention paid to the study of underground pipes. This study proposes a hybrid multilayer perceptron-convolutional neural network (MLP-CNN) model to predict ground sink susceptibility in urban areas using an underground pipe map. A dataset was constructed by extracting table and image data from the underground pipe map, and it was used to train the model. The test results indicated that the model could accurately predict ground sink susceptibility with an accuracy of 93.87 % and f1-score of 0.9478. Furthermore, we generated a ground sink susceptibility map (GSSM) using a trained model. The findings of this study confirmed that the hybrid MLP-CNN model is capable of reliably predicting ground sink susceptibility.
更多查看译文
关键词
Deep learning,Hybrid MLP-CNN,Urban area,Underground pipe,Ground sink susceptibility
AI 理解论文
溯源树
样例
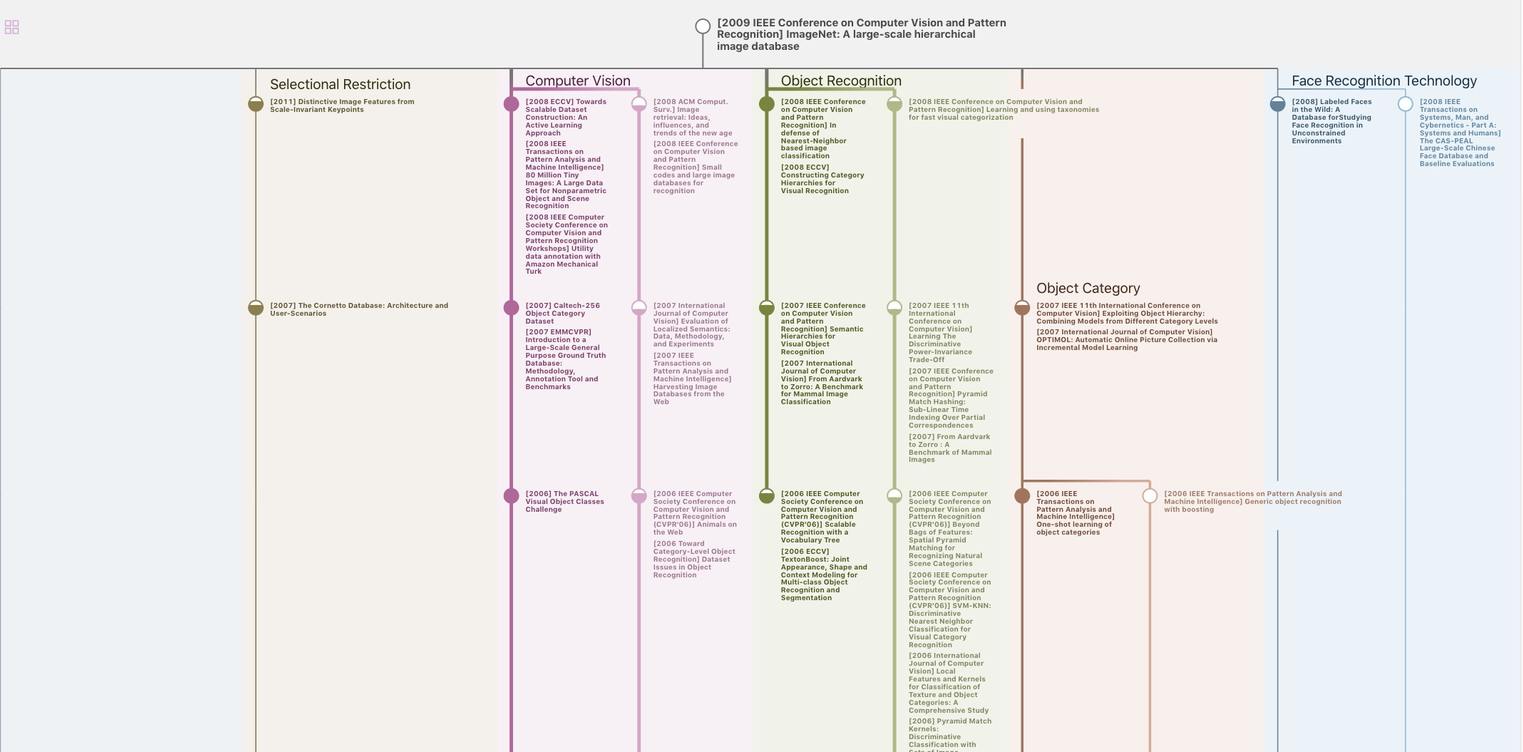
生成溯源树,研究论文发展脉络
Chat Paper
正在生成论文摘要