Toward predicting thermal conductivity of hybrid nanofluids: Application of a committee of robust neural networks, theoretical, and empirical models
POWDER TECHNOLOGY(2024)
摘要
In this work, three optimized intelligent models on the basis of multilayer perceptron (MLP), two radial basis function (RBF) models (including RBF- particle swarm optimization (PSO) and RBF- farmland fertility algorithm (FFA)), and a committee machine intelligent system (CMIS) were used to estimate Thermal Conductivity (TC) of hybrid nanofluids. Base fluid TC, TC of first and second nanoparticles, size of first and second nanoparticles, volume fractions of first and second nanoparticles, and temperature were considered as inputs to model TC of hybrid nanofluids. Based on the results, the CMIS model emerged as the most accurate model for predicting TC coefficient of hybrid nanofluids, boasting an average absolute percent relative error (AAPRE) of just 1.064%. Comparison with various empirical and theoretical models further highlighted the superior predictive performance of the CMIS model in assessing the TC of hybrid nanofluids. Ultimately, the leverage technique underscored the reliability of the CMIS model.
更多查看译文
关键词
Thermal conductivity,Hybrid nanofluids,MLP,RBF,CMIS,Leverage technique
AI 理解论文
溯源树
样例
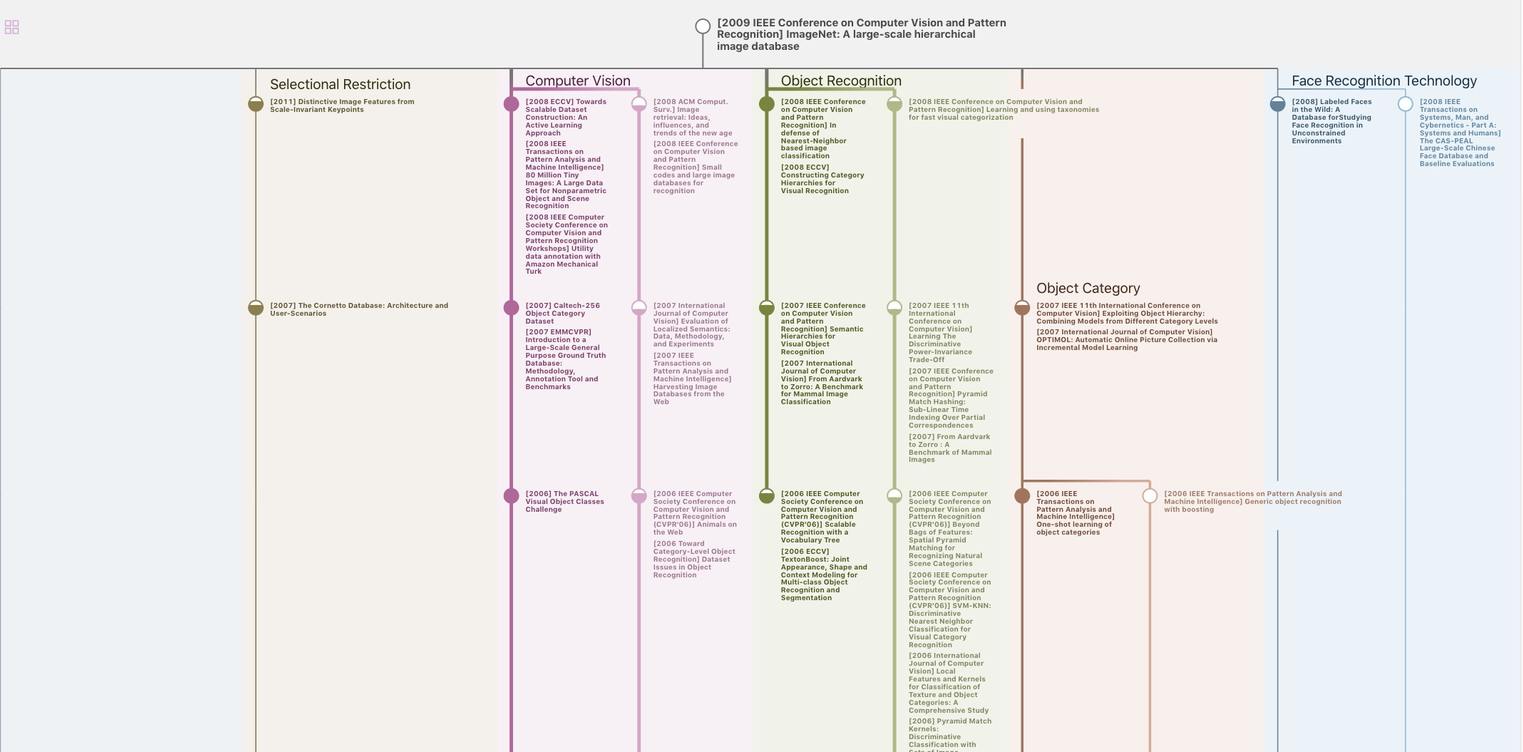
生成溯源树,研究论文发展脉络
Chat Paper
正在生成论文摘要