DSCA: A Dual Semantic Correlation Alignment Method for domain adaptation object detection
Pattern Recognition(2024)
摘要
In self-driving cars, adverse weather (e.g., fog, rain, snow, and cloud) or occlusion scenarios result in domain shift being unavoidable in object detection. Researchers have recently proposed Domain Adaptive Object Detection (DAOD), i.e., aligning the source and target domains at the image and instance levels distribution by utilizing the Unsupervised Domain Adaptation (UDA) method. However, the semantic correlation information is ignored leading to the effect of aligning not good, and low detection accuracy of objects in adverse weather or occlusion scenarios. Here, we propose a Dual Semantic Correlation Alignment (DSCA) method for DAOD to address the problem. The core idea behind DSCA is to make full use of semantic correlation information including context correlation semantic information and class correlation semantic information to align object semantic information in source and target domains, which supplement and enhance the missing information for target domains. It consists of a two-level semantic alignment: (1) context correlation semantic alignment is developed to obtain the context correlation semantic information of the object to align context semantic information at the image level; (2) class correlation semantic alignment is proposed to obtain the class correlation semantic information of the object to align class semantic information at the instance level. The two-level semantic alignment can effectively decrease negative transfer and complete object information to improve the detection accuracy of objects in different domains. Experiments on four challenging benchmarks show that our proposed DSCA method outperforms state-of-the-art DAOD methods.
更多查看译文
关键词
Object detection,Domain Adaptive Object Detection,Domain adaptation,Semantic information,Semantic Correlation Alignment
AI 理解论文
溯源树
样例
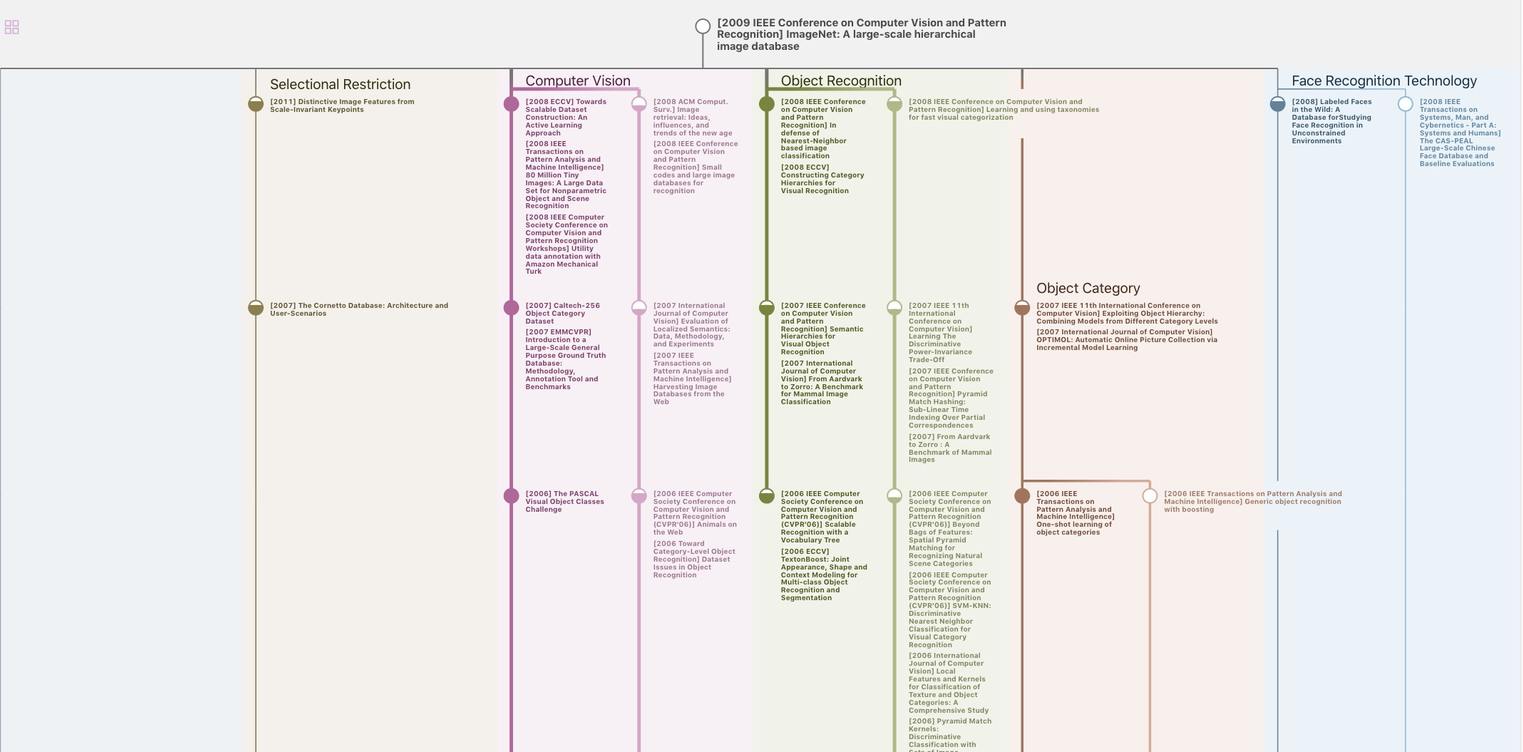
生成溯源树,研究论文发展脉络
Chat Paper
正在生成论文摘要