Improving generalized zero-shot learning via cluster-based semantic disentangling representation
PATTERN RECOGNITION(2024)
摘要
Generalized Zero -Shot Learning (GZSL) aims to recognize both seen and unseen classes by training only the seen classes, in which the instances of unseen classes tend to be biased towards the seen class. In this paper, we propose a Cluster -based Semantic Disentangling Representation (CSDR) method to improve GZSL by alleviating the problems of domain shift and semantic gap. First, we cluster the seen data into multiple clusters, where the samples in each cluster belong to several original seen categories, so as to facilitate finegrained semantic disentangling of visual feature vectors. Then, we introduce representation random swapping and contrastive learning based on the clustering results to realize the disentangling semantic representations of semantic -unspecific, class -shared, and class -unique. The fine-grained semantic disentangling representations show high intra-class similarity and inter -class discriminability, which improve the performance of GZSL by alleviating the problem of domain shift. Finally, we construct the visual -semantic embedding space by the variational auto -encoder and alignment module, which can bridge the semantic gap by generating strongly discriminative unseen class samples. Extensive experimental results on four public data sets prove that our method significantly outperforms state-of-the-art methods in generalized and conventional settings.
更多查看译文
关键词
Generalized zero-shot learning,Domain shift,Semantic gap,Cluster,Semantic disentangling representation
AI 理解论文
溯源树
样例
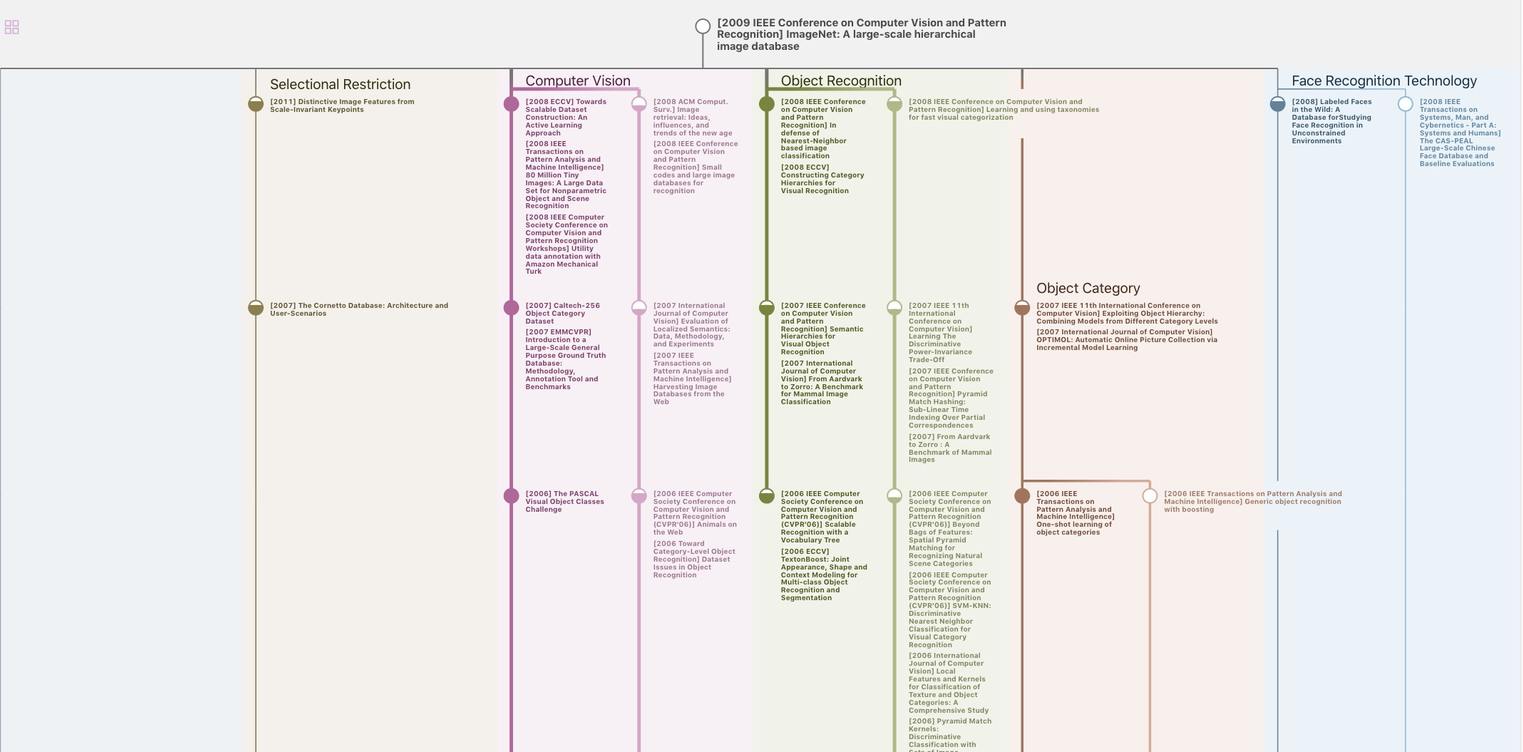
生成溯源树,研究论文发展脉络
Chat Paper
正在生成论文摘要