Unsupervised incremental estimation of Gaussian mixture models with 1D split moves
Pattern Recognition(2024)
摘要
In this paper, we propose a new type of split rule for incremental estimation of Gaussian Mixture Models with model selection. Split-based methods typically start with a mixture composed of a single component representing all data, and successively split and optimize components for a given model selection criterion. These algorithms are typically faster than alternatives, but depend critically on the component splitting method, since a good split rule promotes a faster convergence of the mixture optimization phase. We propose a new efficient and robust split rule that projects mixture components onto a 1D subspace and fits a two-component model to the projected data with the Expectation Maximization algorithm. The proposed approach is fast and robust to parameter tuning, being the ideal choice for applications that favor speed while still maintaining an acceptable accuracy. We illustrate the validity of the method through a series of experiments on synthetic and real datasets comparing the proposed method to alternatives of the state-of-the-art in terms of efficiency, accuracy, and sensitivity to parameter tuning.
更多查看译文
关键词
Unsupervised learning,Gaussian mixture models,Model selection,Split and merge methods
AI 理解论文
溯源树
样例
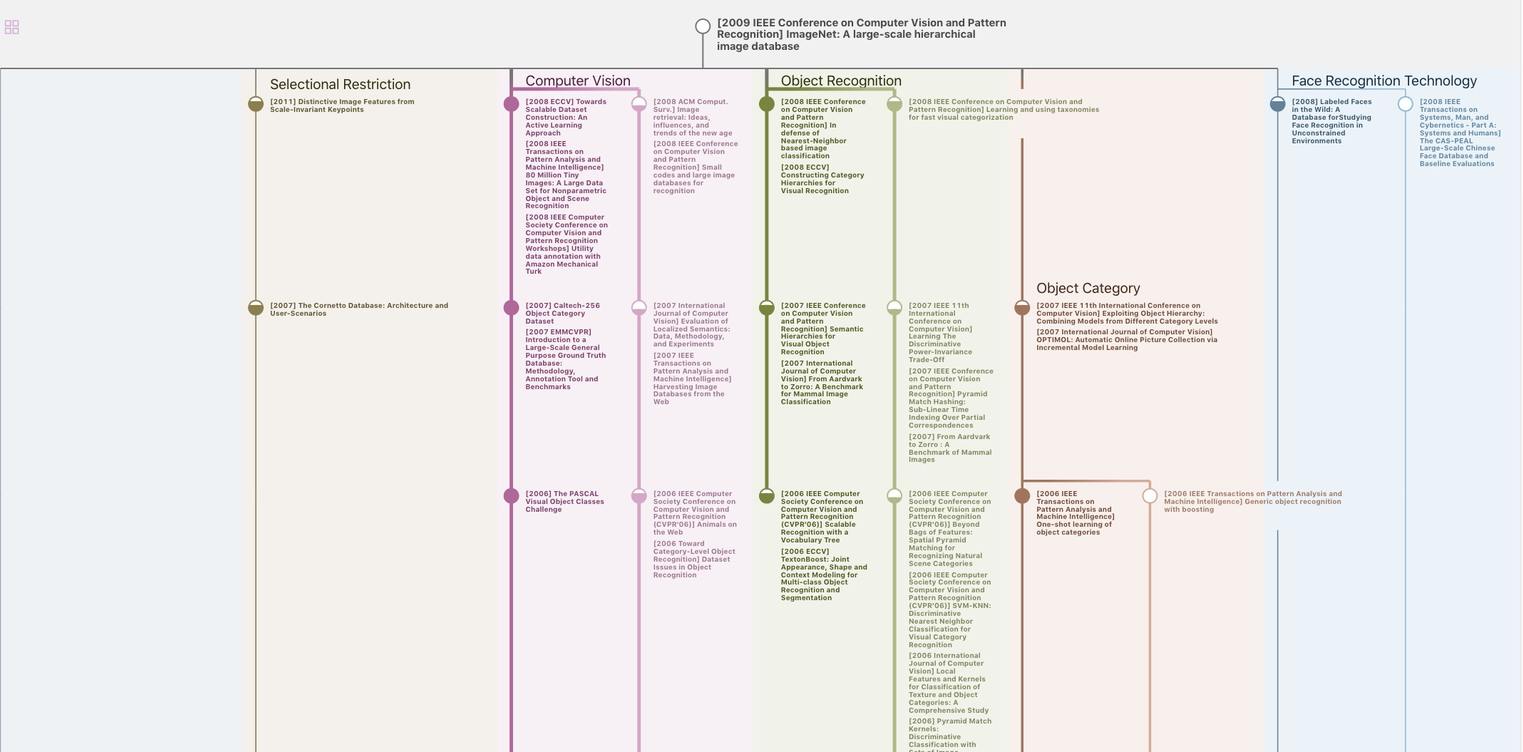
生成溯源树,研究论文发展脉络
Chat Paper
正在生成论文摘要