Deep-NFA: A deep a contrario framework for tiny object detection
PATTERN RECOGNITION(2024)
摘要
The detection of tiny objects is a challenging task in computer vision. Conventional object detection methods have difficulties in finding the balance between high detection rate and low false alarm rate. In the literature, some methods have addressed this issue by enhancing the feature map responses for small objects, but without guaranteeing robustness with respect to the number of false alarms induced by background elements. To tackle this problem, we introduce an a contrario decision criterion into the learning process to take into account the unexpectedness of tiny objects. This statistic criterion enhances the feature map responses while controlling the number of false alarms (NFA) and can be integrated as an add-on into any semantic segmentation neural network. Our add-on NFA module not only allows us to obtain competitive results for small target, road crack and ship detection tasks respectively, but also leads to more robust and interpretable results.
更多查看译文
关键词
A contrario reasoning,One-class semantic segmentation,Deep learning,Convolutional neural networks,Small target detection
AI 理解论文
溯源树
样例
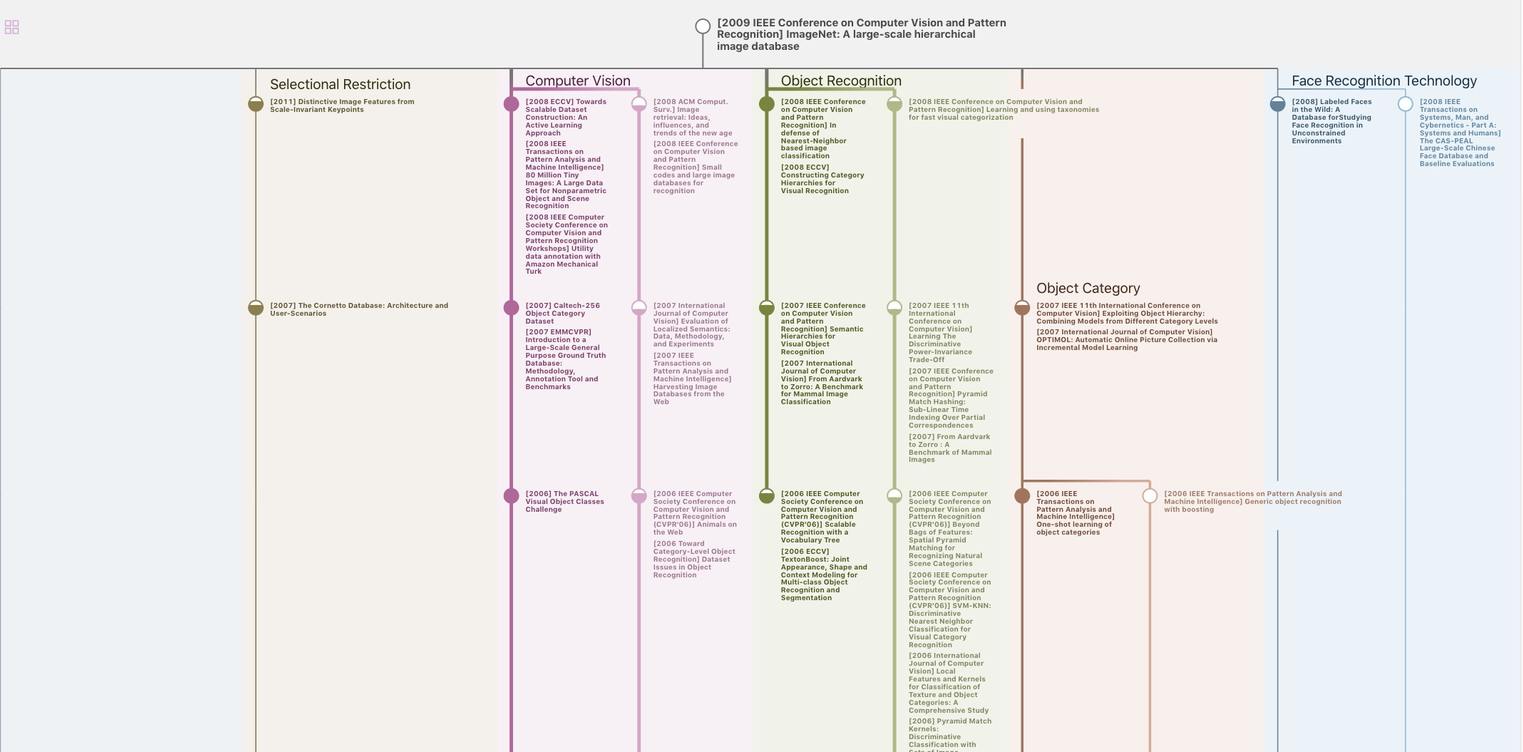
生成溯源树,研究论文发展脉络
Chat Paper
正在生成论文摘要