Machine learning-assisted collection of reduced sensor data for improved analytics pipeline
Procedia CIRP(2024)
摘要
Sensor data is increasingly offering better operational visibility. However, the data deluge is also posing cost and complexity challenges on the data analytics pipeline, which comprises of edge computing, power, transmission, and storage for data-driven decision making. To address the data deluge problem, we propose a machine learning assisted approach of collecting less data upfront to solve different sensor data analytics problems. While sampling at Nyquist rates, we do not collect every data point, but rather sample according to the information content in the signal. A comprehensive experimental design is undertaken to show that collecting more than a certain fraction of raw data only leads to infinitesimal performance improvements. The engineering advantages of the proposed near real-time approach are quantified showing a significant reduction in analytics pipeline resources required for industrial digital transformation applications.
更多查看译文
关键词
Edge computing,machine learning,big data,digital transformation,compressed sensing
AI 理解论文
溯源树
样例
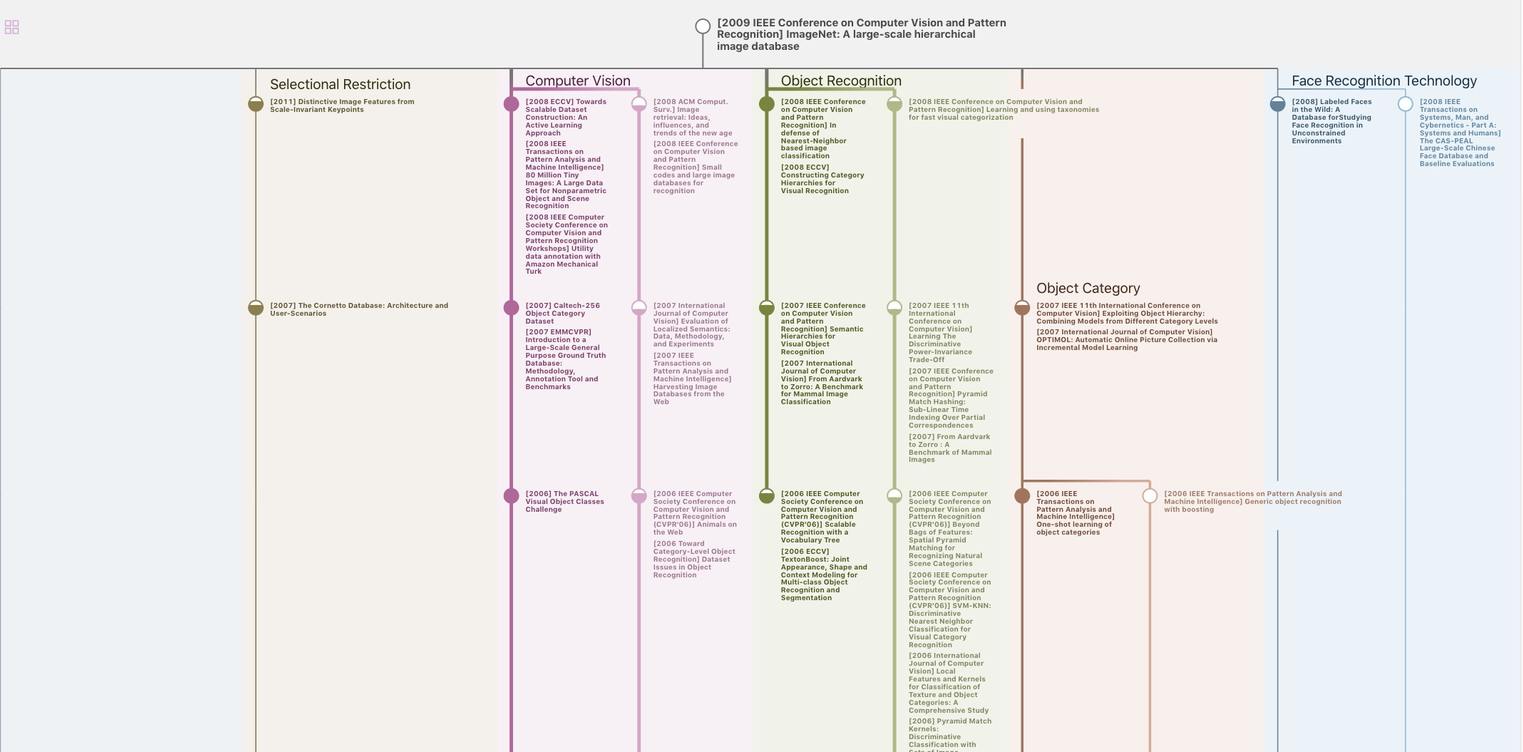
生成溯源树,研究论文发展脉络
Chat Paper
正在生成论文摘要